- Deutschland
- United Kingdom
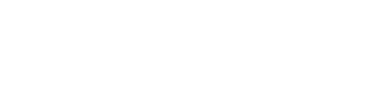
- PhD Dissertations
- Master’s Dissertations
- Bachelor’s Dissertations
- Scientific Dissertations
- Medical Dissertations
- Bioscience Dissertations
- Social Sciences Dissertations
- Psychology Dissertations
- Humanities Dissertations
- Engineering Dissertations
- Economics Dissertations
- Service Overview
- Revisión en inglés
- Relecture en anglais
- Revisão em inglês

Manuscript Editing
- Research Paper Editing
- Lektorat Doktorarbeit
- Dissertation Proofreading
- Englisches Lektorat
- Journal Manuscript Editing
- Scientific Manuscript Editing Services
- Book Manuscript Editing
- PhD Thesis Proofreading Services
- Wissenschaftslektorat
- Korektura anglického textu
- Akademisches Lektorat
- Journal Article Editing
- Manuscript Editing Services
PhD Thesis Editing
- Medical Editing Sciences
- Proofreading Rates UK
- Medical Proofreading
- PhD Proofreading
- Academic Proofreading
- PhD Proofreaders
- Best Dissertation Proofreaders
- Masters Dissertation Proofreading
- Proofreading PhD Thesis Price
- PhD Dissertation Editing
- Lektorat Englisch Preise
- Lektorieren Englisch
- Wissenschaftliches Lektorat
- Thesis Proofreading Services
- PhD Thesis Proofreading
- Proofreading Thesis Cost
- Proofreading Thesis
- Thesis Editing Services
- Professional Thesis Editing
- PhD Thesis Editing Services
- Thesis Editing Cost
- Dissertation Proofreading Services
- Proofreading Dissertation
PhD Dissertation Proofreading
- Dissertation Proofreading Cost
- Dissertation Proofreader
- Correção de Artigos Científicos
- Correção de Trabalhos Academicos
- Serviços de Correção de Inglês
- Correção de Dissertação
- Correção de Textos Precos
- Revision en Ingles
- Revision de Textos en Ingles
- Revision de Tesis
- Revision Medica en Ingles
- Revision de Tesis Precio
- Revisão de Artigos Científicos
- Revisão de Trabalhos Academicos
- Serviços de Revisão de Inglês
- Revisão de Dissertação
- Revisão de Textos Precos
- Corrección de Textos en Ingles
- Corrección de Tesis
- Corrección de Tesis Precio
- Corrección Medica en Ingles
- Corrector ingles
- Choosing the right Journal
- Journal Editor’s Feedback
- Dealing with Rejection
- Quantitative Research Examples
- Number of scientific papers published per year
- Acknowledgements Example
- ISO, ANSI, CFR & Other
- Types of Peer Review
- Withdrawing a Paper
- What is a good h-index
- Appendix paper
- Cover Letter Templates
- Writing an Article
- How To Write the Findings
- Abbreviations: ‘Ibid.’ & ‘Id.’
- Sample letter to editor for publication
- Tables and figures in research paper
- Journal Metrics
- Revision Process of Journal Publishing
- JOURNAL GUIDELINES
Select Page
Writing the Data Analysis Chapter(s): Results and Evidence
Posted by Rene Tetzner | Oct 19, 2021 | PhD Success | 0 |
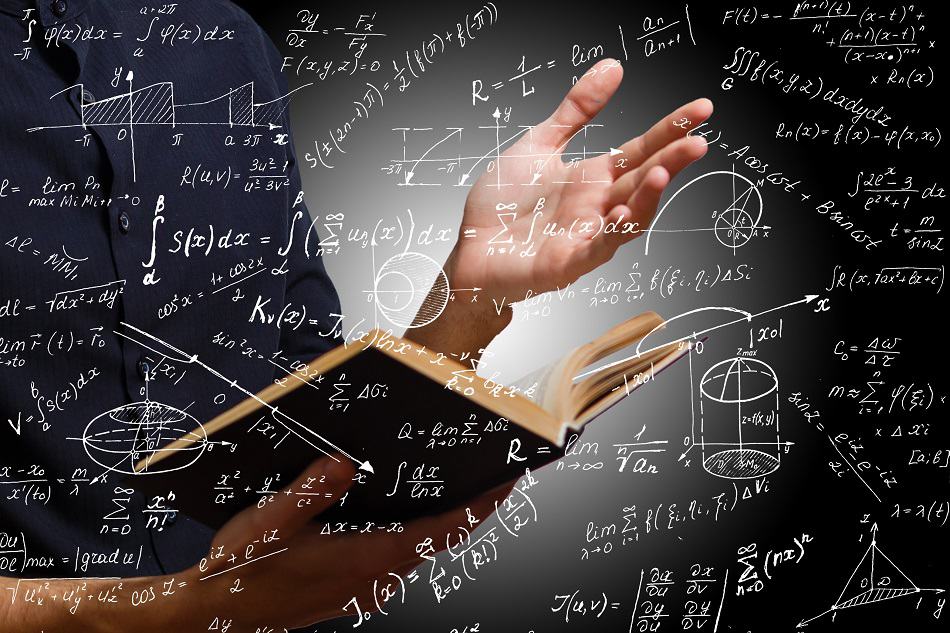
4.4 Writing the Data Analysis Chapter(s): Results and Evidence
Unlike the introduction, literature review and methodology chapter(s), your results chapter(s) will need to be written for the first time as you draft your thesis even if you submitted a proposal, though this part of your thesis will certainly build upon the preceding chapters. You should have carefully recorded and collected the data (test results, participant responses, computer print outs, observations, transcriptions, notes of various kinds etc.) from your research as you conducted it, so now is the time to review, organise and analyse the data. If your study is quantitative in nature, make sure that you know what all the numbers mean and that you consider them in direct relation to the topic, problem or phenomenon you are investigating, and especially in relation to your research questions and hypotheses. You may find that you require the services of a statistician to help make sense of the data, in which case, obtaining that help sooner rather than later is advisable, because you need to understand your results thoroughly before you can write about them. If, on the other hand, your study is qualitative, you will need to read through the data you have collected several times to become familiar with them both as a whole and in detail so that you can establish important themes, patterns and categories. Remember that ‘qualitative analysis is a creative process and requires thoughtful judgments about what is significant and meaningful in the data’ (Roberts, 2010, p.174; see also Miles & Huberman, 1994) – judgements that often need to be made before the findings can be effectively analysed and presented. If you are combining methodologies in your research, you will also need to consider relationships between the results obtained from the different methods, integrating all the data you have obtained and discovering how the results of one approach support or correlate with the results of another. Ideally, you will have taken careful notes recording your initial thoughts and analyses about the sources you consulted and the results and evidence provided by particular methods and instruments as you put them into practice (as suggested in Sections 2.1.2 and 2.1.4), as these will prove helpful while you consider how best to present your results in your thesis.
Although the ways in which to present and organise the results of doctoral research differ markedly depending on the nature of the study and its findings, as on author and committee preferences and university and department guidelines, there are several basic principles that apply to virtually all theses. First and foremost is the need to present the results of your research both clearly and concisely, and in as objective and factual a manner as possible. There will be time and space to elaborate and interpret your results and speculate on their significance and implications in the final discussion chapter(s) of your thesis, but, generally speaking, such reflection on the meaning of the results should be entirely separate from the factual report of your research findings. There are exceptions, of course, and some candidates, supervisors and departments may prefer the factual presentation and interpretive discussion of results to be blended, just as some thesis topics may demand such treatment, but this is rare and best avoided unless there are persuasive reasons to avoid separating the facts from your thoughts about them. If you do find that you need to blend facts and interpretation in reporting your results, make sure that your language leaves no doubt about the line between the two: words such as ‘seems,’ ‘appears,’ ‘may,’ ‘might,’ probably’ and the like will effectively distinguish analytical speculation from more factual reporting (see also Section 4.5).

You need not dedicate much space in this part of the thesis to the methods you used to arrive at your results because these have already been described in your methodology chapter(s), but they can certainly be revisited briefly to clarify or lend structure to your report. Results are most often presented in a straightforward narrative form which is often supplemented by tables and perhaps by figures such as graphs, charts and maps. An effective approach is to decide immediately which information would be best included in tables and figures, and then to prepare those tables and figures before you begin writing the text for the chapter (see Section 4.4.1 on designing effective tables and figures). Arranging your data into the visually immediate formats provided by tables and figures can, for one, produce interesting surprises by enabling you to see trends and details that you may not have noticed previously, and writing the report of your results will prove easier when you have the tables and figures to work with just as your readers ultimately will. In addition, while the text of the results chapter(s) should certainly highlight the most notable data included in tables and figures, it is essential not to repeat information unnecessarily, so writing with the tables and figures already constructed will help you keep repetition to a minimum. Finally, writing about the tables and figures you create will help you test their clarity and effectiveness for your readers, and you can make any necessary adjustments to the tables and figures as you work. Be sure to refer to each table and figure by number in your text and to make it absolutely clear what you want your readers to see or understand in the table or figure (e.g., ‘see Table 1 for the scores’ and ‘Figure 2 shows this relationship’).

Beyond combining textual narration with the data presented in tables and figures, you will need to organise your report of the results in a manner best suited to the material. You may choose to arrange the presentation of your results chronologically or in a hierarchical order that represents their importance; you might subdivide your results into sections (or separate chapters if there is a great deal of information to accommodate) focussing on the findings of different kinds of methodology (quantitative versus qualitative, for instance) or of different tests, trials, surveys, reviews, case studies and so on; or you may want to create sections (or chapters) focussing on specific themes, patterns or categories or on your research questions and/or hypotheses. The last approach allows you to cluster results that relate to a particular question or hypothesis into a single section and can be particularly useful because it provides cohesion for the thesis as a whole and forces you to focus closely on the issues central to the topic, problem or phenomenon you are investigating. You will, for instance, be able to refer back to the questions and hypotheses presented in your introduction (see Section 3.1), to answer the questions and confirm or dismiss the hypotheses and to anticipate in relation to those questions and hypotheses the discussion and interpretation of your findings that will appear in the next part of the thesis (see Section 4.5). Less effective is an approach that organises the presentation of results according to the items of a survey or questionnaire, because these lend the structure of the instrument used to the results instead of connecting those results directly to the aims, themes and argument of your thesis, but such an organisation can certainly be an important early step in your analysis of the findings and might even be valid for the final thesis if, for instance, your work focuses on developing the instrument involved.

The results generated by doctoral research are unique, and this book cannot hope to outline all the possible approaches for presenting the data and analyses that constitute research results, but it is essential that you devote considerable thought and special care to the way in which you structure the report of your results (Section 6.1 on headings may prove helpful). Whatever structure you choose should accurately reflect the nature of your results and highlight their most important and interesting trends, and it should also effectively allow you (in the next part of the thesis) to discuss and speculate upon your findings in ways that will test the premises of your study, work well in the overall argument of your thesis and lead to significant implications for your research. Regardless of how you organise the main body of your results chapter(s), however, you should include a final paragraph (or more than one paragraph if necessary) that briefly summarises and explains the key results and also guides the reader on to the discussion and interpretation of those results in the following chapter(s).
Why PhD Success?
To Graduate Successfully
This article is part of a book called "PhD Success" which focuses on the writing process of a phd thesis, with its aim being to provide sound practices and principles for reporting and formatting in text the methods, results and discussion of even the most innovative and unique research in ways that are clear, correct, professional and persuasive.
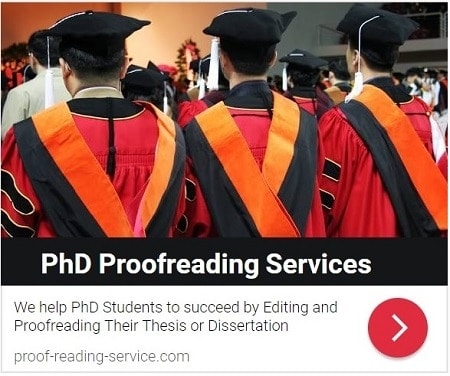
The assumption of the book is that the doctoral candidate reading it is both eager to write and more than capable of doing so, but nonetheless requires information and guidance on exactly what he or she should be writing and how best to approach the task. The basic components of a doctoral thesis are outlined and described, as are the elements of complete and accurate scholarly references, and detailed descriptions of writing practices are clarified through the use of numerous examples.
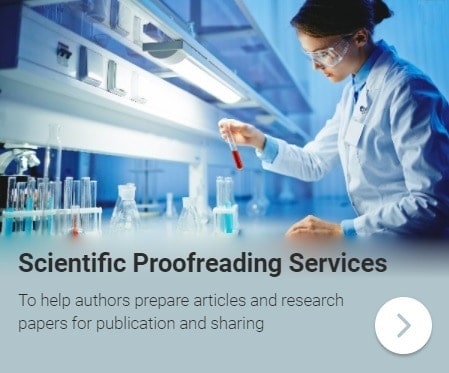
The basic components of a doctoral thesis are outlined and described, as are the elements of complete and accurate scholarly references, and detailed descriptions of writing practices are clarified through the use of numerous examples. PhD Success provides guidance for students familiar with English and the procedures of English universities, but it also acknowledges that many theses in the English language are now written by candidates whose first language is not English, so it carefully explains the scholarly styles, conventions and standards expected of a successful doctoral thesis in the English language.
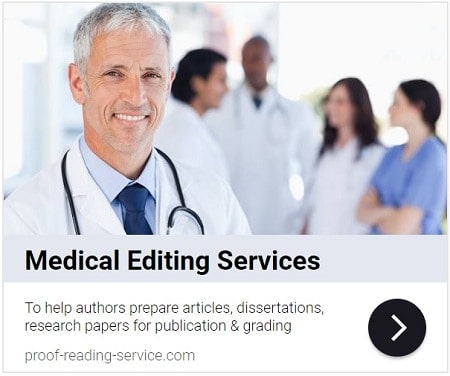
Individual chapters of this book address reflective and critical writing early in the thesis process; working successfully with thesis supervisors and benefiting from commentary and criticism; drafting and revising effective thesis chapters and developing an academic or scientific argument; writing and formatting a thesis in clear and correct scholarly English; citing, quoting and documenting sources thoroughly and accurately; and preparing for and excelling in thesis meetings and examinations.
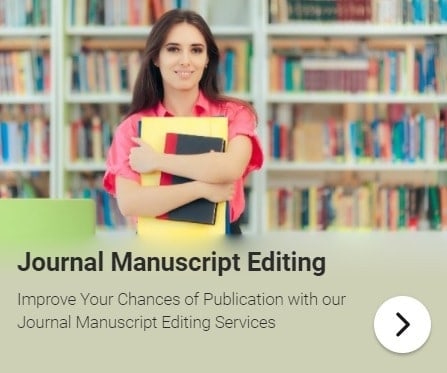
Completing a doctoral thesis successfully requires long and penetrating thought, intellectual rigour and creativity, original research and sound methods (whether established or innovative), precision in recording detail and a wide-ranging thoroughness, as much perseverance and mental toughness as insight and brilliance, and, no matter how many helpful writing guides are consulted, a great deal of hard work over a significant period of time. Writing a thesis can be an enjoyable as well as a challenging experience, however, and even if it is not always so, the personal and professional rewards of achieving such an enormous goal are considerable, as all doctoral candidates no doubt realise, and will last a great deal longer than any problems that may be encountered during the process.
Interested in Proofreading your PhD Thesis? Get in Touch with us
If you are interested in proofreading your PhD thesis or dissertation, please explore our expert dissertation proofreading services.

Rene Tetzner
Rene Tetzner's blog posts dedicated to academic writing. Although the focus is on How To Write a Doctoral Thesis, many other important aspects of research-based writing, editing and publishing are addressed in helpful detail.
Related Posts
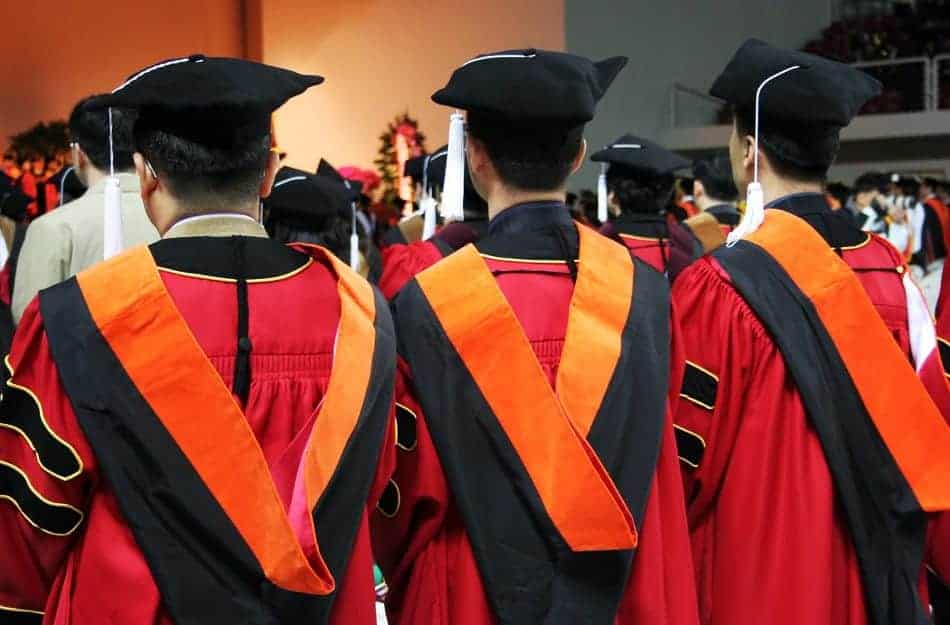
PhD Success – How To Write a Doctoral Thesis
October 1, 2021
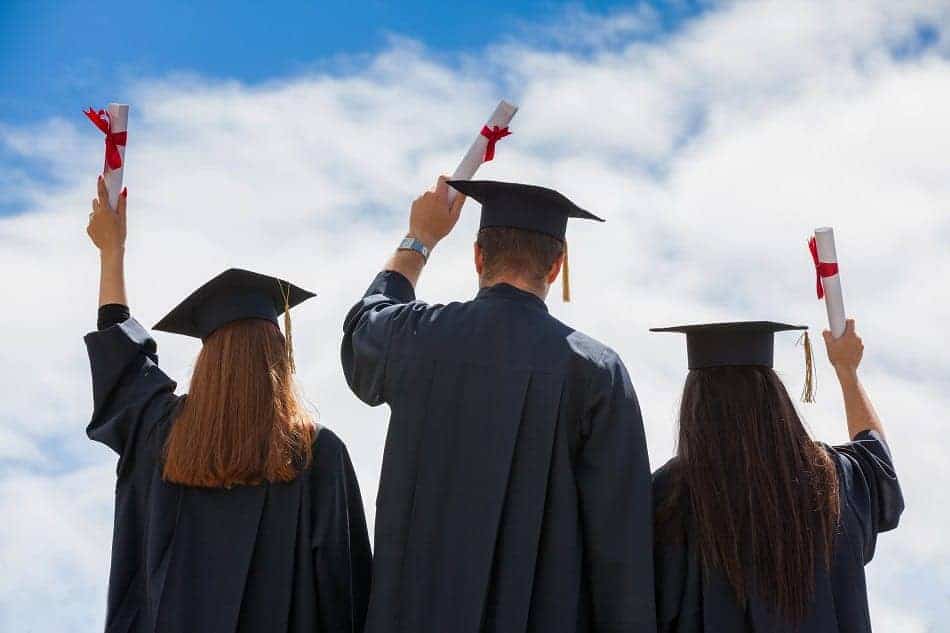
Table of Contents – PhD Success
October 2, 2021
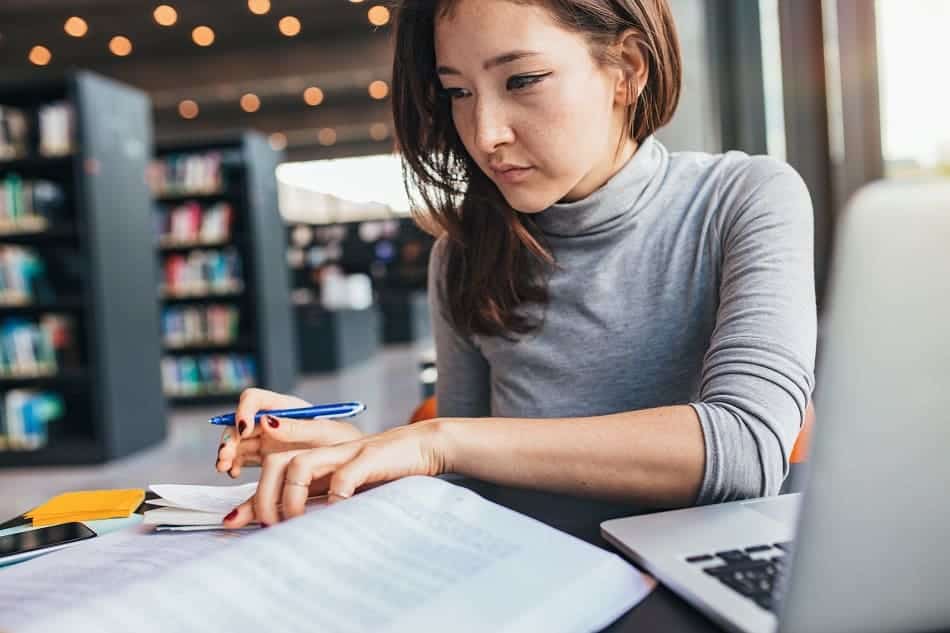
The Essential – Preliminary Matter
October 3, 2021
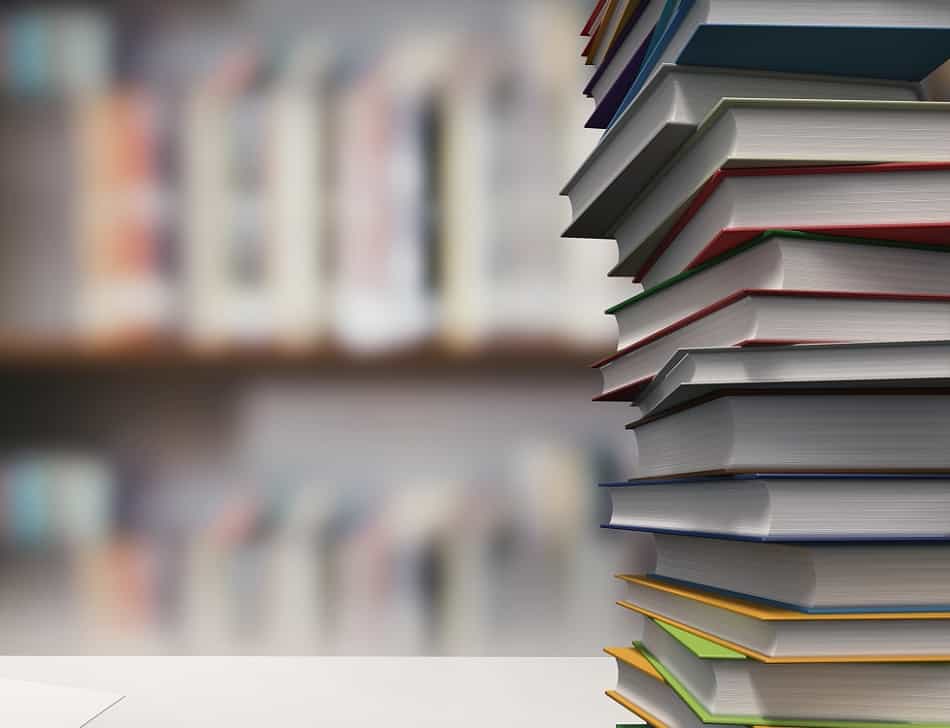
The Main Body of the Thesis
October 4, 2021
- Cookies & Privacy
- GETTING STARTED
- Introduction
- FUNDAMENTALS

Getting to the main article
Choosing your route
Setting research questions/ hypotheses
Assessment point
Building the theoretical case
Setting your research strategy
Data collection
Data analysis
Data analysis techniques
In STAGE NINE: Data analysis , we discuss the data you will have collected during STAGE EIGHT: Data collection . However, before you collect your data, having followed the research strategy you set out in this STAGE SIX , it is useful to think about the data analysis techniques you may apply to your data when it is collected.
The statistical tests that are appropriate for your dissertation will depend on (a) the research questions/hypotheses you have set, (b) the research design you are using, and (c) the nature of your data. You should already been clear about your research questions/hypotheses from STAGE THREE: Setting research questions and/or hypotheses , as well as knowing the goal of your research design from STEP TWO: Research design in this STAGE SIX: Setting your research strategy . These two pieces of information - your research questions/hypotheses and research design - will let you know, in principle , the statistical tests that may be appropriate to run on your data in order to answer your research questions.
We highlight the words in principle and may because the most appropriate statistical test to run on your data not only depend on your research questions/hypotheses and research design, but also the nature of your data . As you should have identified in STEP THREE: Research methods , and in the article, Types of variables , in the Fundamentals part of Lærd Dissertation, (a) not all data is the same, and (b) not all variables are measured in the same way (i.e., variables can be dichotomous, ordinal or continuous). In addition, not all data is normal , nor is the data when comparing groups necessarily equal , terms we explain in the Data Analysis section in the Fundamentals part of Lærd Dissertation. As a result, you might think that running a particular statistical test is correct at this point of setting your research strategy (e.g., a statistical test called a dependent t-test ), based on the research questions/hypotheses you have set, but when you collect your data (i.e., during STAGE EIGHT: Data collection ), the data may fail certain assumptions that are important to such a statistical test (i.e., normality and homogeneity of variance ). As a result, you have to run another statistical test (e.g., a Wilcoxon signed-rank test instead of a dependent t-test ).
At this stage in the dissertation process, it is important, or at the very least, useful to think about the data analysis techniques you may apply to your data when it is collected. We suggest that you do this for two reasons:
REASON A Supervisors sometimes expect you to know what statistical analysis you will perform at this stage of the dissertation process
This is not always the case, but if you have had to write a Dissertation Proposal or Ethics Proposal , there is sometimes an expectation that you explain the type of data analysis that you plan to carry out. An understanding of the data analysis that you will carry out on your data can also be an expected component of the Research Strategy chapter of your dissertation write-up (i.e., usually Chapter Three: Research Strategy ). Therefore, it is a good time to think about the data analysis process if you plan to start writing up this chapter at this stage.
REASON B It takes time to get your head around data analysis
When you come to analyse your data in STAGE NINE: Data analysis , you will need to think about (a) selecting the correct statistical tests to perform on your data, (b) running these tests on your data using a statistics package such as SPSS, and (c) learning how to interpret the output from such statistical tests so that you can answer your research questions or hypotheses. Whilst we show you how to do this for a wide range of scenarios in the in the Data Analysis section in the Fundamentals part of Lærd Dissertation, it can be a time consuming process. Unless you took an advanced statistics module/option as part of your degree (i.e., not just an introductory course to statistics, which are often taught in undergraduate and master?s degrees), it can take time to get your head around data analysis. Starting this process at this stage (i.e., STAGE SIX: Research strategy ), rather than waiting until you finish collecting your data (i.e., STAGE EIGHT: Data collection ) is a sensible approach.
Final thoughts...
Setting the research strategy for your dissertation required you to describe, explain and justify the research paradigm, quantitative research design, research method(s), sampling strategy, and approach towards research ethics and data analysis that you plan to follow, as well as determine how you will ensure the research quality of your findings so that you can effectively answer your research questions/hypotheses. However, from a practical perspective, just remember that the main goal of STAGE SIX: Research strategy is to have a clear research strategy that you can implement (i.e., operationalize ). After all, if you are unable to clearly follow your plan and carry out your research in the field, you will struggle to answer your research questions/hypotheses. Once you are sure that you have a clear plan, it is a good idea to take a step back, speak with your supervisor, and assess where you are before moving on to collect data. Therefore, when you are ready, proceed to STAGE SEVEN: Assessment point .
The Art of Data Analysis
- First Online: 05 January 2021
Cite this chapter
- Sarah Cuschieri 2
Part of the book series: SpringerBriefs in Public Health ((BRIEFSPUBLIC))
542 Accesses
1 Citations
Descriptive and analytic studies will need to be performed as part of most PhD theses. The initial step is to identify whether the data you have gathered follows a normal or a skewed distribution pattern. In normal distribution data, parametric tests need to be used (e.g. mean and student t -test), while in skewed data, non-parametric tests are used (e.g. median and Mann–Whitney U test). Your data can be either continuous or categorical. Statistical testing for comparisons and establishing relationships will depend on the data type (continuous or categorical) and the distribution of the data (normal or skewed).
This is a preview of subscription content, log in via an institution to check access.
Access this chapter
- Available as EPUB and PDF
- Read on any device
- Instant download
- Own it forever
- Compact, lightweight edition
- Dispatched in 3 to 5 business days
- Free shipping worldwide - see info
Tax calculation will be finalised at checkout
Purchases are for personal use only
Institutional subscriptions
Cuschieri S (2019) The burden of type 2 diabetes mellitus, dysglycaemia and their co-determinants in the adult population of Malta. University of Malta
Google Scholar
Download references
Author information
Authors and affiliations.
University of Malta, Msida, Malta
Sarah Cuschieri
You can also search for this author in PubMed Google Scholar
Rights and permissions
Reprints and permissions
Copyright information
© 2021 The Author(s), under exclusive license to Springer Nature Switzerland AG
About this chapter
Cuschieri, S. (2021). The Art of Data Analysis. In: To Do or Not to Do a PhD?. SpringerBriefs in Public Health. Springer, Cham. https://doi.org/10.1007/978-3-030-64671-4_5
Download citation
DOI : https://doi.org/10.1007/978-3-030-64671-4_5
Published : 05 January 2021
Publisher Name : Springer, Cham
Print ISBN : 978-3-030-64670-7
Online ISBN : 978-3-030-64671-4
eBook Packages : Medicine Medicine (R0)
Share this chapter
Anyone you share the following link with will be able to read this content:
Sorry, a shareable link is not currently available for this article.
Provided by the Springer Nature SharedIt content-sharing initiative
- Publish with us
Policies and ethics
- Find a journal
- Track your research
ON YOUR 1ST ORDER
Mastering Dissertation Data Analysis: A Comprehensive Guide
By Laura Brown on 29th December 2023
To craft an effective dissertation data analysis chapter, you need to follow some simple steps:
- Start by planning the structure and objectives of the chapter.
- Clearly set the stage by providing a concise overview of your research design and methodology.
- Proceed to thorough data preparation, ensuring accuracy and organisation.
- Justify your methods and present the results using visual aids for clarity.
- Discuss the findings within the context of your research questions.
- Finally, review and edit your chapter to ensure coherence.
This approach will ensure a well-crafted and impactful analysis section.
Before delving into details on how you can come up with an engaging data analysis show in your dissertation, we first need to understand what it is and why it is required.
What Is Data Analysis In A Dissertation?
The data analysis chapter is a crucial section of a research dissertation that involves the examination, interpretation, and synthesis of collected data. In this chapter, researchers employ statistical techniques, qualitative methods, or a combination of both to make sense of the data gathered during the research process.
Why Is The Data Analysis Chapter So Important?
The primary objectives of the data analysis chapter are to identify patterns, trends, relationships, and insights within the data set. Researchers use various tools and software to conduct a thorough analysis, ensuring that the results are both accurate and relevant to the research questions or hypotheses. Ultimately, the findings derived from this chapter contribute to the overall conclusions of the dissertation, providing a basis for drawing meaningful and well-supported insights.
Steps Required To Craft Data Analysis Chapter To Perfection
Now that we have an idea of what a dissertation analysis chapter is and why it is necessary to put it in the dissertation, let’s move towards how we can create one that has a significant impact. Our guide will move around the bulleted points that have been discussed initially in the beginning. So, it’s time to begin.
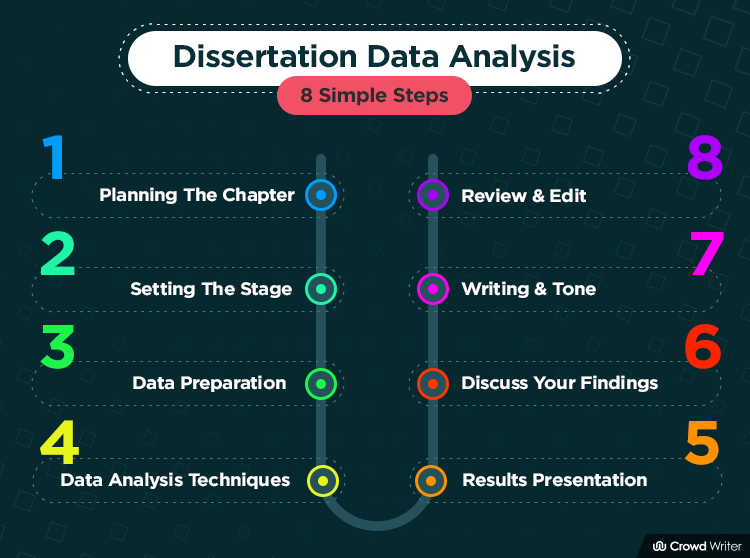
Step 1: Planning Your Data Analysis Chapter
Planning your data analysis chapter is a critical precursor to its successful execution.
- Begin by outlining the chapter structure to provide a roadmap for your analysis.
- Start with an introduction that succinctly introduces the purpose and significance of the data analysis in the context of your research.
- Following this, delineate the chapter into sections such as Data Preparation, where you detail the steps taken to organise and clean your data.
- Plan on to clearly define the Data Analysis Techniques employed, justifying their relevance to your research objectives.
- As you progress, plan for the Results Presentation, incorporating visual aids for clarity. Lastly, earmark a section for the Discussion of Findings, where you will interpret results within the broader context of your research questions.
This structured approach ensures a comprehensive and cohesive data analysis chapter, setting the stage for a compelling narrative that contributes significantly to your dissertation. You can always seek our dissertation data analysis help to plan your chapter.
Step 2: Setting The Stage – Introduction to Data Analysis
Your primary objective is to establish a solid foundation for the analytical journey. You need to skillfully link your data analysis to your research questions, elucidating the direct relevance and purpose of the upcoming analysis.
Simultaneously, define key concepts to provide clarity and ensure a shared understanding of the terms integral to your study. Following this, offer a concise overview of your data set characteristics, outlining its source, nature, and any noteworthy features.
This meticulous groundwork alongside our help with dissertation data analysis lays the base for a coherent and purposeful chapter, guiding readers seamlessly into the subsequent stages of your dissertation.
Step 3: Data Preparation
Now this is another pivotal phase in the data analysis process, ensuring the integrity and reliability of your findings. You should start with an insightful overview of the data cleaning and preprocessing procedures, highlighting the steps taken to refine and organise your dataset. Then, discuss any challenges encountered during the process and the strategies employed to address them.
Moving forward, delve into the specifics of data transformation procedures, elucidating any alterations made to the raw data for analysis. Clearly describe the methods employed for normalisation, scaling, or any other transformations deemed necessary. It will not only enhance the quality of your analysis but also foster transparency in your research methodology, reinforcing the robustness of your data-driven insights.
Step 4: Data Analysis Techniques
The data analysis section of a dissertation is akin to choosing the right tools for an artistic masterpiece. Carefully weigh the quantitative and qualitative approaches, ensuring a tailored fit for the nature of your data.
Quantitative Analysis
- Descriptive Statistics: Paint a vivid picture of your data through measures like mean, median, and mode. It’s like capturing the essence of your data’s personality.
- Inferential Statistics:Take a leap into the unknown, making educated guesses and inferences about your larger population based on a sample. It’s statistical magic in action.
Qualitative Analysis
- Thematic Analysis: Imagine your data as a novel, and thematic analysis as the tool to uncover its hidden chapters. Dissect the narrative, revealing recurring themes and patterns.
- Content Analysis: Scrutinise your data’s content like detectives, identifying key elements and meanings. It’s a deep dive into the substance of your qualitative data.
Providing Rationale for Chosen Methods
You should also articulate the why behind the chosen methods. It’s not just about numbers or themes; it’s about the story you want your data to tell. Through transparent rationale, you should ensure that your chosen techniques align seamlessly with your research goals, adding depth and credibility to the analysis.
Step 5: Presentation Of Your Results
You can simply break this process into two parts.
a. Creating Clear and Concise Visualisations
Effectively communicate your findings through meticulously crafted visualisations. Use tables that offer a structured presentation, summarising key data points for quick comprehension. Graphs, on the other hand, visually depict trends and patterns, enhancing overall clarity. Thoughtfully design these visual aids to align with the nature of your data, ensuring they serve as impactful tools for conveying information.
b. Interpreting and Explaining Results
Go beyond mere presentation by providing insightful interpretation by taking data analysis services for dissertation. Show the significance of your findings within the broader research context. Moreover, articulates the implications of observed patterns or relationships. By weaving a narrative around your results, you guide readers through the relevance and impact of your data analysis, enriching the overall understanding of your dissertation’s key contributions.
Step 6: Discussion of Findings
While discussing your findings and dissertation discussion chapter , it’s like putting together puzzle pieces to understand what your data is saying. You can always take dissertation data analysis help to explain what it all means, connecting back to why you started in the first place.
Be honest about any limitations or possible biases in your study; it’s like showing your cards to make your research more trustworthy. Comparing your results to what other smart people have found before you adds to the conversation, showing where your work fits in.
Looking ahead, you suggest ideas for what future researchers could explore, keeping the conversation going. So, it’s not just about what you found, but also about what comes next and how it all fits into the big picture of what we know.
Step 7: Writing Style and Tone
In order to perfectly come up with this chapter, follow the below points in your writing and adjust the tone accordingly,
- Use clear and concise language to ensure your audience easily understands complex concepts.
- Avoid unnecessary jargon in data analysis for thesis, and if specialised terms are necessary, provide brief explanations.
- Keep your writing style formal and objective, maintaining an academic tone throughout.
- Avoid overly casual language or slang, as the data analysis chapter is a serious academic document.
- Clearly define terms and concepts, providing specific details about your data preparation and analysis procedures.
- Use precise language to convey your ideas, minimising ambiguity.
- Follow a consistent formatting style for headings, subheadings, and citations to enhance readability.
- Ensure that tables, graphs, and visual aids are labelled and formatted uniformly for a polished presentation.
- Connect your analysis to the broader context of your research by explaining the relevance of your chosen methods and the importance of your findings.
- Offer a balance between detail and context, helping readers understand the significance of your data analysis within the larger study.
- Present enough detail to support your findings but avoid overwhelming readers with excessive information.
- Use a balance of text and visual aids to convey information efficiently.
- Maintain reader engagement by incorporating transitions between sections and effectively linking concepts.
- Use a mix of sentence structures to add variety and keep the writing engaging.
- Eliminate grammatical errors, typos, and inconsistencies through thorough proofreading.
- Consider seeking feedback from peers or mentors to ensure the clarity and coherence of your writing.
You can seek a data analysis dissertation example or sample from CrowdWriter to better understand how we write it while following the above-mentioned points.
Step 8: Reviewing and Editing
Reviewing and editing your data analysis chapter is crucial for ensuring its effectiveness and impact. By revising your work, you refine the clarity and coherence of your analysis, enhancing its overall quality.
Seeking feedback from peers, advisors or dissertation data analysis services provides valuable perspectives, helping identify blind spots and areas for improvement. Addressing common writing pitfalls, such as grammatical errors or unclear expressions, ensures your chapter is polished and professional.
Taking the time to review and edit not only strengthens the academic integrity of your work but also contributes to a final product that is clear, compelling, and ready for scholarly scrutiny.
Concluding On This Data Analysis Help
Be it master thesis data analysis, an undergraduate one or for PhD scholars, the steps remain almost the same as we have discussed in this guide. The primary focus is to be connected with your research questions and objectives while writing your data analysis chapter.
Do not lose your focus and choose the right analysis methods and design. Make sure to present your data through various visuals to better explain your data and engage the reader as well. At last, give it a detailed read and seek assistance from experts and your supervisor for further improvement.
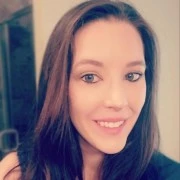
Laura Brown, a senior content writer who writes actionable blogs at Crowd Writer.
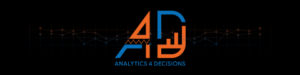
11 Tips For Writing a Dissertation Data Analysis
Since the evolution of the fourth industrial revolution – the Digital World; lots of data have surrounded us. There are terabytes of data around us or in data centers that need to be processed and used. The data needs to be appropriately analyzed to process it, and Dissertation data analysis forms its basis. If data analysis is valid and free from errors, the research outcomes will be reliable and lead to a successful dissertation.
Considering the complexity of many data analysis projects, it becomes challenging to get precise results if analysts are not familiar with data analysis tools and tests properly. The analysis is a time-taking process that starts with collecting valid and relevant data and ends with the demonstration of error-free results.
So, in today’s topic, we will cover the need to analyze data, dissertation data analysis, and mainly the tips for writing an outstanding data analysis dissertation. If you are a doctoral student and plan to perform dissertation data analysis on your data, make sure that you give this article a thorough read for the best tips!
What is Data Analysis in Dissertation?
Dissertation Data Analysis is the process of understanding, gathering, compiling, and processing a large amount of data. Then identifying common patterns in responses and critically examining facts and figures to find the rationale behind those outcomes.
Even f you have the data collected and compiled in the form of facts and figures, it is not enough for proving your research outcomes. There is still a need to apply dissertation data analysis on your data; to use it in the dissertation. It provides scientific support to the thesis and conclusion of the research.
Data Analysis Tools
There are plenty of indicative tests used to analyze data and infer relevant results for the discussion part. Following are some tests used to perform analysis of data leading to a scientific conclusion:
11 Most Useful Tips for Dissertation Data Analysis
Doctoral students need to perform dissertation data analysis and then dissertation to receive their degree. Many Ph.D. students find it hard to do dissertation data analysis because they are not trained in it.
1. Dissertation Data Analysis Services
The first tip applies to those students who can afford to look for help with their dissertation data analysis work. It’s a viable option, and it can help with time management and with building the other elements of the dissertation with much detail.
Dissertation Analysis services are professional services that help doctoral students with all the basics of their dissertation work, from planning, research and clarification, methodology, dissertation data analysis and review, literature review, and final powerpoint presentation.
One great reference for dissertation data analysis professional services is Statistics Solutions , they’ve been around for over 22 years helping students succeed in their dissertation work. You can find the link to their website here .
For a proper dissertation data analysis, the student should have a clear understanding and statistical knowledge. Through this knowledge and experience, a student can perform dissertation analysis on their own.
Following are some helpful tips for writing a splendid dissertation data analysis:
2. Relevance of Collected Data
If the data is irrelevant and not appropriate, you might get distracted from the point of focus. To show the reader that you can critically solve the problem, make sure that you write a theoretical proposition regarding the selection and analysis of data.
3. Data Analysis
For analysis, it is crucial to use such methods that fit best with the types of data collected and the research objectives. Elaborate on these methods and the ones that justify your data collection methods thoroughly. Make sure to make the reader believe that you did not choose your method randomly. Instead, you arrived at it after critical analysis and prolonged research.
On the other hand, quantitative analysis refers to the analysis and interpretation of facts and figures – to build reasoning behind the advent of primary findings. An assessment of the main results and the literature review plays a pivotal role in qualitative and quantitative analysis.
The overall objective of data analysis is to detect patterns and inclinations in data and then present the outcomes implicitly. It helps in providing a solid foundation for critical conclusions and assisting the researcher to complete the dissertation proposal.
4. Qualitative Data Analysis
Qualitative data refers to data that does not involve numbers. You are required to carry out an analysis of the data collected through experiments, focus groups, and interviews. This can be a time-taking process because it requires iterative examination and sometimes demanding the application of hermeneutics. Note that using qualitative technique doesn’t only mean generating good outcomes but to unveil more profound knowledge that can be transferrable.
Presenting qualitative data analysis in a dissertation can also be a challenging task. It contains longer and more detailed responses. Placing such comprehensive data coherently in one chapter of the dissertation can be difficult due to two reasons. Firstly, we cannot figure out clearly which data to include and which one to exclude. Secondly, unlike quantitative data, it becomes problematic to present data in figures and tables. Making information condensed into a visual representation is not possible. As a writer, it is of essence to address both of these challenges.
Qualitative Data Analysis Methods
Following are the methods used to perform quantitative data analysis.
- Deductive Method
This method involves analyzing qualitative data based on an argument that a researcher already defines. It’s a comparatively easy approach to analyze data. It is suitable for the researcher with a fair idea about the responses they are likely to receive from the questionnaires.
- Inductive Method
In this method, the researcher analyzes the data not based on any predefined rules. It is a time-taking process used by students who have very little knowledge of the research phenomenon.
5. Quantitative Data Analysis
Quantitative data contains facts and figures obtained from scientific research and requires extensive statistical analysis. After collection and analysis, you will be able to conclude. Generic outcomes can be accepted beyond the sample by assuming that it is representative – one of the preliminary checkpoints to carry out in your analysis to a larger group. This method is also referred to as the “scientific method”, gaining its roots from natural sciences.
The Presentation of quantitative data depends on the domain to which it is being presented. It is beneficial to consider your audience while writing your findings. Quantitative data for hard sciences might require numeric inputs and statistics. As for natural sciences , such comprehensive analysis is not required.
Quantitative Analysis Methods
Following are some of the methods used to perform quantitative data analysis.
- Trend analysis: This corresponds to a statistical analysis approach to look at the trend of quantitative data collected over a considerable period.
- Cross-tabulation: This method uses a tabula way to draw readings among data sets in research.
- Conjoint analysis : Quantitative data analysis method that can collect and analyze advanced measures. These measures provide a thorough vision about purchasing decisions and the most importantly, marked parameters.
- TURF analysis: This approach assesses the total market reach of a service or product or a mix of both.
- Gap analysis: It utilizes the side-by-side matrix to portray quantitative data, which captures the difference between the actual and expected performance.
- Text analysis: In this method, innovative tools enumerate open-ended data into easily understandable data.
6. Data Presentation Tools
Since large volumes of data need to be represented, it becomes a difficult task to present such an amount of data in coherent ways. To resolve this issue, consider all the available choices you have, such as tables, charts, diagrams, and graphs.
Tables help in presenting both qualitative and quantitative data concisely. While presenting data, always keep your reader in mind. Anything clear to you may not be apparent to your reader. So, constantly rethink whether your data presentation method is understandable to someone less conversant with your research and findings. If the answer is “No”, you may need to rethink your Presentation.
7. Include Appendix or Addendum
After presenting a large amount of data, your dissertation analysis part might get messy and look disorganized. Also, you would not be cutting down or excluding the data you spent days and months collecting. To avoid this, you should include an appendix part.
The data you find hard to arrange within the text, include that in the appendix part of a dissertation . And place questionnaires, copies of focus groups and interviews, and data sheets in the appendix. On the other hand, one must put the statistical analysis and sayings quoted by interviewees within the dissertation.
8. Thoroughness of Data
It is a common misconception that the data presented is self-explanatory. Most of the students provide the data and quotes and think that it is enough and explaining everything. It is not sufficient. Rather than just quoting everything, you should analyze and identify which data you will use to approve or disapprove your standpoints.
Thoroughly demonstrate the ideas and critically analyze each perspective taking care of the points where errors can occur. Always make sure to discuss the anomalies and strengths of your data to add credibility to your research.
9. Discussing Data
Discussion of data involves elaborating the dimensions to classify patterns, themes, and trends in presented data. In addition, to balancing, also take theoretical interpretations into account. Discuss the reliability of your data by assessing their effect and significance. Do not hide the anomalies. While using interviews to discuss the data, make sure you use relevant quotes to develop a strong rationale.
It also involves answering what you are trying to do with the data and how you have structured your findings. Once you have presented the results, the reader will be looking for interpretation. Hence, it is essential to deliver the understanding as soon as you have submitted your data.
10. Findings and Results
Findings refer to the facts derived after the analysis of collected data. These outcomes should be stated; clearly, their statements should tightly support your objective and provide logical reasoning and scientific backing to your point. This part comprises of majority part of the dissertation.
In the finding part, you should tell the reader what they are looking for. There should be no suspense for the reader as it would divert their attention. State your findings clearly and concisely so that they can get the idea of what is more to come in your dissertation.
11. Connection with Literature Review
At the ending of your data analysis in the dissertation, make sure to compare your data with other published research. In this way, you can identify the points of differences and agreements. Check the consistency of your findings if they meet your expectations—lookup for bottleneck position. Analyze and discuss the reasons behind it. Identify the key themes, gaps, and the relation of your findings with the literature review. In short, you should link your data with your research question, and the questions should form a basis for literature.

The Role of Data Analytics at The Senior Management Level
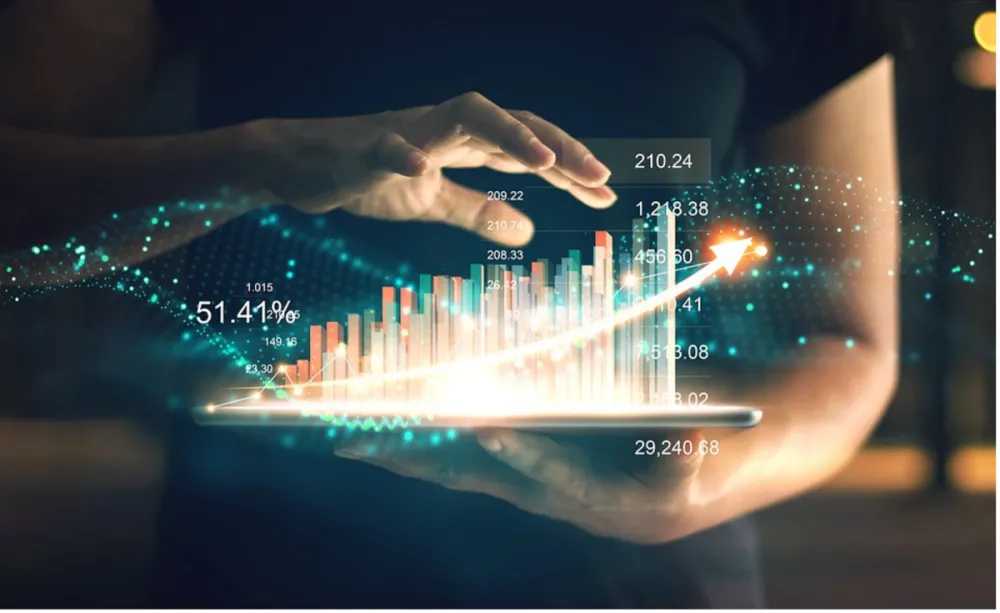
From small and medium-sized businesses to Fortune 500 conglomerates, the success of a modern business is now increasingly tied to how the company implements its data infrastructure and data-based decision-making. According
The Decision-Making Model Explained (In Plain Terms)

Any form of the systematic decision-making process is better enhanced with data. But making sense of big data or even small data analysis when venturing into a decision-making process might
13 Reasons Why Data Is Important in Decision Making
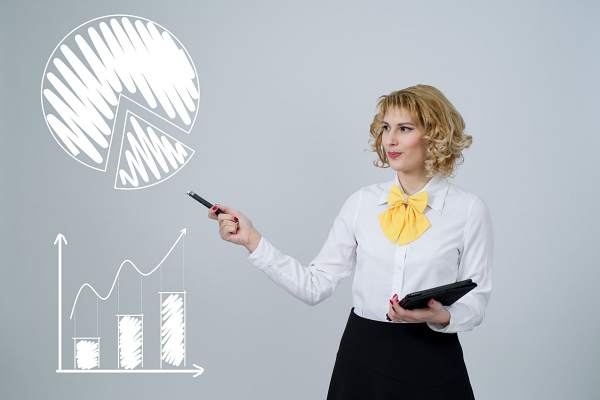
Wrapping Up
Writing data analysis in the dissertation involves dedication, and its implementations demand sound knowledge and proper planning. Choosing your topic, gathering relevant data, analyzing it, presenting your data and findings correctly, discussing the results, connecting with the literature and conclusions are milestones in it. Among these checkpoints, the Data analysis stage is most important and requires a lot of keenness.
In this article, we thoroughly looked at the tips that prove valuable for writing a data analysis in a dissertation. Make sure to give this article a thorough read before you write data analysis in the dissertation leading to the successful future of your research.
Oxbridge Essays. Top 10 Tips for Writing a Dissertation Data Analysis.
Emidio Amadebai
As an IT Engineer, who is passionate about learning and sharing. I have worked and learned quite a bit from Data Engineers, Data Analysts, Business Analysts, and Key Decision Makers almost for the past 5 years. Interested in learning more about Data Science and How to leverage it for better decision-making in my business and hopefully help you do the same in yours.
Recent Posts
Causal vs Evidential Decision-making (How to Make Businesses More Effective)
In today’s fast-paced business landscape, it is crucial to make informed decisions to stay in the competition which makes it important to understand the concept of the different characteristics and...
Bootstrapping vs. Boosting
Over the past decade, the field of machine learning has witnessed remarkable advancements in predictive techniques and ensemble learning methods. Ensemble techniques are very popular in machine...


Library Guides
Dissertations 5: findings, analysis and discussion: home.
- Results/Findings
Alternative Structures
The time has come to show and discuss the findings of your research. How to structure this part of your dissertation?
Dissertations can have different structures, as you can see in the dissertation structure guide.
Dissertations organised by sections
Many dissertations are organised by sections. In this case, we suggest three options. Note that, if within your course you have been instructed to use a specific structure, you should do that. Also note that sometimes there is considerable freedom on the structure, so you can come up with other structures too.
A) More common for scientific dissertations and quantitative methods:
- Results chapter
- Discussion chapter
Example:
- Introduction
- Literature review
- Methodology
- (Recommendations)
if you write a scientific dissertation, or anyway using quantitative methods, you will have some objective results that you will present in the Results chapter. You will then interpret the results in the Discussion chapter.
B) More common for qualitative methods
- Analysis chapter. This can have more descriptive/thematic subheadings.
- Discussion chapter. This can have more descriptive/thematic subheadings.
- Case study of Company X (fashion brand) environmental strategies
- Successful elements
- Lessons learnt
- Criticisms of Company X environmental strategies
- Possible alternatives
C) More common for qualitative methods
- Analysis and discussion chapter. This can have more descriptive/thematic titles.
- Case study of Company X (fashion brand) environmental strategies
If your dissertation uses qualitative methods, it is harder to identify and report objective data. Instead, it may be more productive and meaningful to present the findings in the same sections where you also analyse, and possibly discuss, them. You will probably have different sections dealing with different themes. The different themes can be subheadings of the Analysis and Discussion (together or separate) chapter(s).
Thematic dissertations
If the structure of your dissertation is thematic , you will have several chapters analysing and discussing the issues raised by your research. The chapters will have descriptive/thematic titles.
- Background on the conflict in Yemen (2004-present day)
- Classification of the conflict in international law
- International law violations
- Options for enforcement of international law
- Next: Results/Findings >>
- Last Updated: Aug 4, 2023 2:17 PM
- URL: https://libguides.westminster.ac.uk/c.php?g=696975
CONNECT WITH US

Ace Your Data Analysis
Get hands-on help analysing your data from a friendly Grad Coach. It’s like having a professor in your pocket.

Students Helped
Client pass rate, trustpilot score, facebook rating, how we help you .
Whether you’ve just started collecting your data, are in the thick of analysing it, or you’ve already written a draft chapter – we’re here to help.
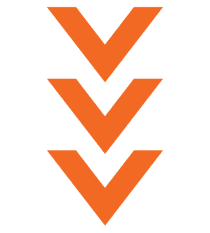
Make sense of the data
If you’ve collected your data, but are feeling confused about what to do and how to make sense of it all, we can help. One of our friendly coaches will hold your hand through each step and help you interpret your dataset .
Alternatively, if you’re still planning your data collection and analysis strategy, we can help you craft a rock-solid methodology that sets you up for success.
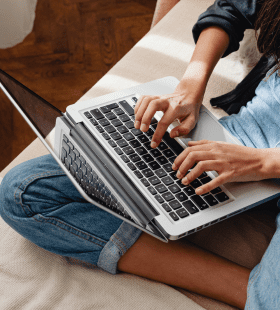
Get your thinking onto paper
If you’ve analysed your data, but are struggling to get your thoughts onto paper, one of our friendly Grad Coaches can help you structure your results and/or discussion chapter to kickstart your writing.
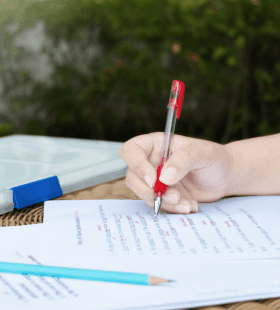
Refine your writing
If you’ve already written up your results but need a second set of eyes, our popular Content Review service can help you identify and address key issues within your writing, before you submit it for grading .
Why Grad Coach ?
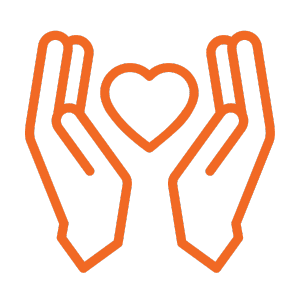
It's all about you
We take the time to understand your unique challenges and work with you to achieve your specific academic goals . Whether you're aiming to earn top marks or just need to cross the finish line, we're here to help.
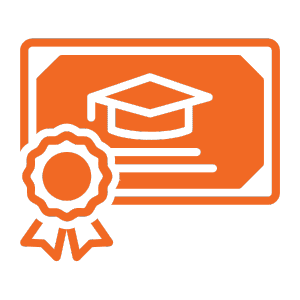
An insider advantage
Our award-winning Dissertation Coaches all hold doctoral-level degrees and share 100+ years of combined academic experience. Having worked on "the inside", we know exactly what markers want .
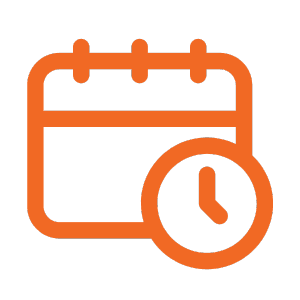
Any time, anywhere
Getting help from your dedicated Dissertation Coach is simple. Book a live video /voice call, chat via email or send your document to us for an in-depth review and critique . We're here when you need us.
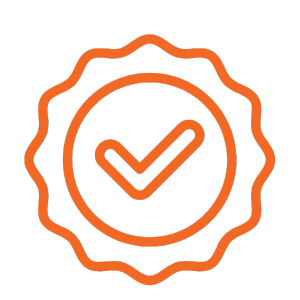
A track record you can trust
Over 10 million students have enjoyed our online lessons and courses, while 3000+ students have benefited from 1:1 Private Coaching. The plethora of glowing reviews reflects our commitment.
Chat With A Friendly Coach, Today
Prefer email? No problem - you c an email us here .

Have a question ?
Below we address some of the most popular questions we receive regarding our data analysis support, but feel free to get in touch if you have any other questions.
Dissertation Coaching
I have no idea where to start. can you help.
Absolutely. We regularly work with students who are completely new to data analysis (both qualitative and quantitative) and need step-by-step guidance to understand and interpret their data.
Can you analyse my data for me?
The short answer – no.
The longer answer:
If you’re undertaking qualitative research , we can fast-track your project with our Qualitative Coding Service. With this service, we take care of the initial coding of your dataset (e.g., interview transcripts), providing a firm foundation on which you can build your qualitative analysis (e.g., thematic analysis, content analysis, etc.).
If you’re undertaking quantitative research , we can fast-track your project with our Statistical Testing Service . With this service, we run the relevant statistical tests using SPSS or R, and provide you with the raw outputs. You can then use these outputs/reports to interpret your results and develop your analysis.
Importantly, in both cases, we are not analysing the data for you or providing an interpretation or write-up for you. If you’d like coaching-based support with that aspect of the project, we can certainly assist you with this (i.e., provide guidance and feedback, review your writing, etc.). But it’s important to understand that you, as the researcher, need to engage with the data and write up your own findings.
Can you help me choose the right data analysis methods?
Yes, we can assist you in selecting appropriate data analysis methods, based on your research aims and research questions, as well as the characteristics of your data.
Which data analysis methods can you assist with?
We can assist with most qualitative and quantitative analysis methods that are commonplace within the social sciences.
Qualitative methods:
- Qualitative content analysis
- Thematic analysis
- Discourse analysis
- Narrative analysis
- Grounded theory
Quantitative methods:
- Descriptive statistics
- Inferential statistics
Can you provide data sets for me to analyse?
If you are undertaking secondary research , we can potentially assist you in finding suitable data sets for your analysis.
If you are undertaking primary research , we can help you plan and develop data collection instruments (e.g., surveys, questionnaires, etc.), but we cannot source the data on your behalf.
Can you write the analysis/results/discussion chapter/section for me?
No. We can provide you with hands-on guidance through each step of the analysis process, but the writing needs to be your own. Writing anything for you would constitute academic misconduct .
Can you help me organise and structure my results/discussion chapter/section?
Yes, we can assist in structuring your chapter to ensure that you have a clear, logical structure and flow that delivers a clear and convincing narrative.
Can you review my writing and give me feedback?
Absolutely. Our Content Review service is designed exactly for this purpose and is one of the most popular services here at Grad Coach. In a Content Review, we carefully read through your research methodology chapter (or any other chapter) and provide detailed comments regarding the key issues/problem areas, why they’re problematic and what you can do to resolve the issues. You can learn more about Content Review here .
Do you provide software support (e.g., SPSS, R, etc.)?
It depends on the software package you’re planning to use, as well as the analysis techniques/tests you plan to undertake. We can typically provide support for the more popular analysis packages, but it’s best to discuss this in an initial consultation.
Can you help me with other aspects of my research project?
Yes. Data analysis support is only one aspect of our offering at Grad Coach, and we typically assist students throughout their entire dissertation/thesis/research project. You can learn more about our full service offering here .
Can I get a coach that specialises in my topic area?
It’s important to clarify that our expertise lies in the research process itself , rather than specific research areas/topics (e.g., psychology, management, etc.).
In other words, the support we provide is topic-agnostic, which allows us to support students across a very broad range of research topics. That said, if there is a coach on our team who has experience in your area of research, as well as your chosen methodology, we can allocate them to your project (dependent on their availability, of course).
If you’re unsure about whether we’re the right fit, feel free to drop us an email or book a free initial consultation.
What qualifications do your coaches have?
All of our coaches hold a doctoral-level degree (for example, a PhD, DBA, etc.). Moreover, they all have experience working within academia, in many cases as dissertation/thesis supervisors. In other words, they understand what markers are looking for when reviewing a student’s work.
Is my data/topic/study kept confidential?
Yes, we prioritise confidentiality and data security. Your written work and personal information are treated as strictly confidential. We can also sign a non-disclosure agreement, should you wish.
I still have questions…
No problem. Feel free to email us or book an initial consultation to discuss.
What our clients say
We've worked 1:1 with 3000+ students . Here's what some of them have to say:
David's depth of knowledge in research methodology was truly impressive. He demonstrated a profound understanding of the nuances and complexities of my research area, offering insights that I hadn't even considered. His ability to synthesize information, identify key research gaps, and suggest research topics was truly inspiring. I felt like I had a true expert by my side, guiding me through the complexities of the proposal.
Cyntia Sacani (US)
I had been struggling with the first 3 chapters of my dissertation for over a year. I finally decided to give GradCoach a try and it made a huge difference. Alexandra provided helpful suggestions along with edits that transformed my paper. My advisor was very impressed.
Tracy Shelton (US)
Working with Kerryn has been brilliant. She has guided me through that pesky academic language that makes us all scratch our heads. I can't recommend Grad Coach highly enough; they are very professional, humble, and fun to work with. If like me, you know your subject matter but you're getting lost in the academic language, look no further, give them a go.
Tony Fogarty (UK)
So helpful! Amy assisted me with an outline for my literature review and with organizing the results for my MBA applied research project. Having a road map helped enormously and saved a lot of time. Definitely worth it.
Jennifer Hagedorn (Canada)
Everything about my experience was great, from Dr. Shaeffer’s expertise, to her patience and flexibility. I reached out to GradCoach after receiving a 78 on a midterm paper. Not only did I get a 100 on my final paper in the same class, but I haven’t received a mark less than A+ since. I recommend GradCoach for everyone who needs help with academic research.
Antonia Singleton (Qatar)
I started using Grad Coach for my dissertation and I can honestly say that if it wasn’t for them, I would have really struggled. I would strongly recommend them – worth every penny!
Richard Egenreider (South Africa)
Fast-Track Your Data Analysis, Today
Enter your details below, pop us an email, or book an introductory consultation .
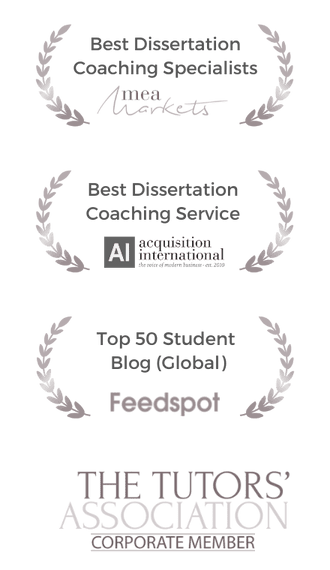
- Hire a PhD Guide
- Guidance Process
- PhD Topic and Proposal Help
- PhD Thesis Chapters Writing
- PhD Literature Review Writing Help
- PhD Research Methodology Chapter Help
- Questionnaire Design for PhD Research
PhD Statistical Analysis Help
- Qualitative Analysis Help for PhD Research
- Software Implementation Help for PhD Projects
- Journal Paper Publication Assistance
- Addressing Comments, Revisions in PhD Thesis
- Enhance the Quality of Your PhD Thesis with Professional Thesis Editing Services
- PhD Thesis Defence Preparation

Ethical research guidance and consulting services for PhD candidates since 2008
Topic selection & proposal development, enquire now, software implementation using matlab, questionnaire designing & data analysis, chapters writing & journal papers.
Statistical data analysis is at the heart of your research, as all your findings are based on the way you analyze your raw data. Thus, it can be costly to make mistakes at this stage of research. If you do not use the right statistical tests, tools and techniques, it can be disastrous for your entire research. It is important to be accurate while analyzing and interpreting your findings. This is where our team of professional statisticians can support you.
- check Questionnaire Design
- check Questionnaire Reliability and Validity Testing
- check Descriptive Analysis
- check Inferential Analysis
- check Predictive Analysis
- check Structural Equation Modelling
- check Anova
- check Manova
- check T Test
- check Chi Square
- check Logistic Regression
- check Correlation
- check SEM using AMOS
- check STATA
- check E-Views
Our Statisticians
Our statisticians ensure that your data is analyzed accurately and your results are well interpreted. Whether it is qualitative or quantitative research, we can handle data analysis of both types. While a quantitative study may require intense analysis, a qualitative study may also not end up with the right results if its data is improperly tabulated and further interpreted. Since both types of research require separate analytical approaches, our statisticians focus on providing relevant assistance using varied tools.
In Case of Your Quantitative Study
In case of your quantitative study, we utilize software like SPSS, Stata, EViews, SAS and AMOS. We also recommend right statistical tests which suit your quantitative study. On the other hand, we make use of packages like NVivo while analyzing data and thematic content for your qualitative study. We additionally help you in designing the methodology for your research so as to effectively answer all research questions.
Request a Quote
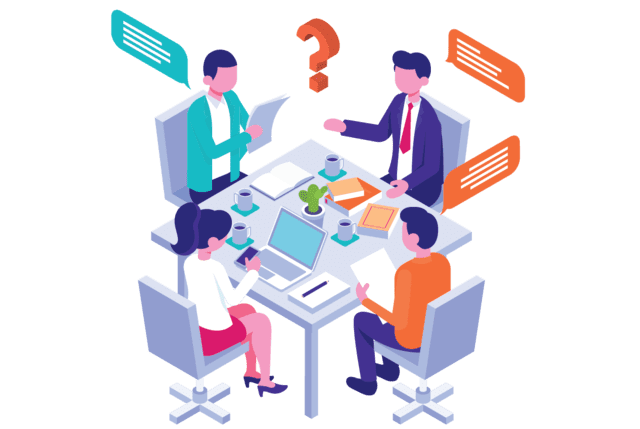
PhD candidates can meet PhD consultant, editors for discussion related to doctoral research and journal paper publications including SCI, UGC approved and IEEE journals.
Frequently Asked Questions
What if your expert do not use the right statistical tests, tools and techniques, is there any specific technique which will be used for data analysis, how will i get to know that the questionnaire developed is reliable, what will be the circumstances, if the result of the statistical help doesn’t satisfy me, how will i get to know that the statistician allocated to me is professional, request call back, quick links.
- PhD Guidance Maharashtra Trail
- Synopsis and Thesis Assistance
- Privacy Policy
- Terms of use
- Schedule Your Consultation Now
- Grievance Redressal
Information
- Geo Polymer for road construction
- Machine Learning for Image processing applications
- IoT and automation
- Concrete strength with changing flyash percentage
- Purchase regret prediction with Deep Learning
- Low Power VLSI
- Antenna design using HFSS
- PhD Planner
CONTACT DETAILS
- 022 4896 4199 (20 Lines)
- 0091 93102 29971
- [email protected]
- Copyright © 2008-2024 PhD Guidance All Rights Reserved.
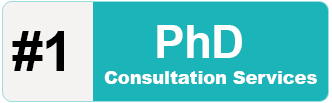
- Introduction
- Conclusions
- Article Information
a Adjusted by trial.
DAPT indicates dual antiplatelet therapy; SVG, saphenous vein graft.
a Type 0 indicates no bleeding; type 1, bleeding that is not actionable and does not cause the patient to seek unscheduled performance of studies, hospitalization, or treatment; type 2, any overt, actionable sign of hemorrhage (eg, bleeding that does not fit type 3, 4, or 5 criteria but meets at least 1 of the following: [1] requires nonsurgical medical intervention, [2] leads to hospitalization or increased level of care, or [3] prompts evaluation); type 3a, overt bleeding plus hemoglobin drop of 3 to 5 g/dL (provided hemoglobin drop is related to the bleeding event) or any transfusion with overt bleeding; 3b, overt bleeding plus hemoglobin drop of 5 g/dL (provided hemoglobin drop is related to the bleeding event), cardiac tamponade, bleeding requiring surgical intervention for control (excluding dental, nasal, skin, or hemorrhoid), and bleeding requiring intravenous vasoactive agents; 3c, intracranial hemorrhage (does not include microbleeds or hemorrhagic transformation, does include intraspinal), subcategories confirmed by autopsy or imaging or lumbar puncture, intraocular bleed compromising vision; type 4, coronary artery bypass graft surgery–related bleeding; and type 5, fatal bleeding.
b Adjusted by trial.
c Post hoc outcomes.
d Secondary outcome.
e Defined as the composite of all-cause death, myocardial infarction, stroke, or revascularization.
f Additional outcomes.
g Defined as the composite of cardiovascular death, myocardial infarction, or stroke.
Includes patients from the TAP-CABG, 8 DACAB, 9 and POPular CABG 10 trials. See the Methods section for the full names of the studies.
CV indicates cardiovascular; DAPT, dual antiplatelet therapy; HR, hazard ratio; MACCE, major adverse cardiac and cerebrovascular event; MACE, major adverse cardiovascular event; OR, odds ratio; SVG, saphenous vein graft.
Subgroup analyses for harvesting technique and target vessel territory could not be performed due to the limited number of events in the endoscopic-harvesting group and left anterior descending coronary artery territory group. Treatment duration varied in 1 trial only.
b Self-reported diagnosis, elevated hemoglobin A 1c levels, or active therapy. Definitions varied by trial.
c Includes ST-segment elevation myocardial infarction, non–ST-segment elevation ACS, or unstable angina. Definitions varied by trial.
d Defined as saphenous vein grafts with more than 1 anastomosis.
ACS indicates acute coronary syndrome; CPB, cardiopulmonary bypass; DAPT, dual antiplatelet therapy; SVG, saphenous vein graft.
eMethods 1. Search strategy
eMethods 2. Combined dataset
eMethods 3. Post hoc analyses
eTable 1. Definition of major adverse cardiac and cerebrovascular events in randomized trials included in the IPD meta-analysis
eTable 2. Patient characteristics for patients with saphenous vein grafts who did versus did not have per-protocol imaging
eTable 3. Patient characteristics for patients with saphenous vein grafts who underwent per-protocol imaging, by randomization group
eTable 4 . Patient characteristics for patients with saphenous vein grafts who did not undergo per-protocol imaging, by randomization group
eTable 5. Sensitivity analyses for the primary outcome
eTable 6. Post hoc analyses for any graft failure
eTable 7 . Net adverse events in patients receiving saphenous vein grafts
eTable 8. Comparison of outcomes for saphenous vein graft failure among patients randomized to ticagrelor monotherapy or aspirin
eTable 9. Comparison of bleeding events among patients randomized to ticagrelor monotherapy or aspirin
eFigure 1. Risk of bias in the included trials as assessed by the Cochrane risk of bias assessment tool 2
eFigure 2. PRISMA IPD Flow Diagram
eFigure 3. Individual and pooled estimates for bleeding events in the primary analysis
eFigure 4 . Sensitivity analysis for saphenous vein graft failure per anastomosis
eFigure 5. Sensitivity analysis for the primary outcome in patients with 1-year imaging
eFigure 6. Post hoc analysis for saphenous vein graft occlusion
eFigure 7. Treatment-by-subgroup interaction for any graft failure
eFigure 8. BARC type 2, 3, or 5 bleeding in subgroups
eFigure 9. Forest plot for saphenous vein graft failure for aspirin, ticagrelor dual antiplatelet therapy, and ticagrelor monotherapy in the post hoc network meta-analysis
eReferences
- Risks and Benefits of Antiplatelet Therapy After Coronary Artery Bypass Surgery JAMA Editorial August 9, 2022 Sunil V. Rao, MD; E. Magnus Ohman, MD
- Dual Antiplatelet Therapy With Ticagrelor and Vein Graft Failure After Coronary Artery Bypass Graft Surgery JAMA Comment & Response December 13, 2022 Sigrid Sandner, MD; Björn Redfors, MD, PhD; Mario Gaudino, MD, PhD
- Dual Antiplatelet Therapy With Ticagrelor and Vein Graft Failure After Coronary Artery Bypass Graft Surgery JAMA Comment & Response December 13, 2022 Bing-Yan Zeng, MD; Ping-Tao Tseng, MD, PhD; Chih-Sung Liang, MD
See More About
Select your interests.
Customize your JAMA Network experience by selecting one or more topics from the list below.
- Academic Medicine
- Acid Base, Electrolytes, Fluids
- Allergy and Clinical Immunology
- American Indian or Alaska Natives
- Anesthesiology
- Anticoagulation
- Art and Images in Psychiatry
- Artificial Intelligence
- Assisted Reproduction
- Bleeding and Transfusion
- Caring for the Critically Ill Patient
- Challenges in Clinical Electrocardiography
- Climate and Health
- Climate Change
- Clinical Challenge
- Clinical Decision Support
- Clinical Implications of Basic Neuroscience
- Clinical Pharmacy and Pharmacology
- Complementary and Alternative Medicine
- Consensus Statements
- Coronavirus (COVID-19)
- Critical Care Medicine
- Cultural Competency
- Dental Medicine
- Dermatology
- Diabetes and Endocrinology
- Diagnostic Test Interpretation
- Drug Development
- Electronic Health Records
- Emergency Medicine
- End of Life, Hospice, Palliative Care
- Environmental Health
- Equity, Diversity, and Inclusion
- Facial Plastic Surgery
- Gastroenterology and Hepatology
- Genetics and Genomics
- Genomics and Precision Health
- Global Health
- Guide to Statistics and Methods
- Hair Disorders
- Health Care Delivery Models
- Health Care Economics, Insurance, Payment
- Health Care Quality
- Health Care Reform
- Health Care Safety
- Health Care Workforce
- Health Disparities
- Health Inequities
- Health Policy
- Health Systems Science
- History of Medicine
- Hypertension
- Images in Neurology
- Implementation Science
- Infectious Diseases
- Innovations in Health Care Delivery
- JAMA Infographic
- Law and Medicine
- Leading Change
- Less is More
- LGBTQIA Medicine
- Lifestyle Behaviors
- Medical Coding
- Medical Devices and Equipment
- Medical Education
- Medical Education and Training
- Medical Journals and Publishing
- Mobile Health and Telemedicine
- Narrative Medicine
- Neuroscience and Psychiatry
- Notable Notes
- Nutrition, Obesity, Exercise
- Obstetrics and Gynecology
- Occupational Health
- Ophthalmology
- Orthopedics
- Otolaryngology
- Pain Medicine
- Palliative Care
- Pathology and Laboratory Medicine
- Patient Care
- Patient Information
- Performance Improvement
- Performance Measures
- Perioperative Care and Consultation
- Pharmacoeconomics
- Pharmacoepidemiology
- Pharmacogenetics
- Pharmacy and Clinical Pharmacology
- Physical Medicine and Rehabilitation
- Physical Therapy
- Physician Leadership
- Population Health
- Primary Care
- Professional Well-being
- Professionalism
- Psychiatry and Behavioral Health
- Public Health
- Pulmonary Medicine
- Regulatory Agencies
- Reproductive Health
- Research, Methods, Statistics
- Resuscitation
- Rheumatology
- Risk Management
- Scientific Discovery and the Future of Medicine
- Shared Decision Making and Communication
- Sleep Medicine
- Sports Medicine
- Stem Cell Transplantation
- Substance Use and Addiction Medicine
- Surgical Innovation
- Surgical Pearls
- Teachable Moment
- Technology and Finance
- The Art of JAMA
- The Arts and Medicine
- The Rational Clinical Examination
- Tobacco and e-Cigarettes
- Translational Medicine
- Trauma and Injury
- Treatment Adherence
- Ultrasonography
- Users' Guide to the Medical Literature
- Vaccination
- Venous Thromboembolism
- Veterans Health
- Women's Health
- Workflow and Process
- Wound Care, Infection, Healing
Others Also Liked
- Download PDF
- X Facebook More LinkedIn
Sandner S , Redfors B , Angiolillo DJ, et al. Association of Dual Antiplatelet Therapy With Ticagrelor With Vein Graft Failure After Coronary Artery Bypass Graft Surgery : A Systematic Review and Meta-analysis . JAMA. 2022;328(6):554–562. doi:10.1001/jama.2022.11966
Manage citations:
© 2024
- Permissions
Association of Dual Antiplatelet Therapy With Ticagrelor With Vein Graft Failure After Coronary Artery Bypass Graft Surgery : A Systematic Review and Meta-analysis
- 1 Department of Cardiac Surgery, Medical University of Vienna, Vienna, Austria
- 2 Weill Cornell Medicine, New York, New York
- 3 Department of Cardiology, Sahlgrenska University Hospital, Gothenburg, Sweden
- 4 Division of Cardiology, University of Florida College of Medicine, Jacksonville
- 5 Department of Cardiothoracic Surgery, Weill Cornell Medicine, New York, New York
- 6 Schulich Heart Centre, Sunnybrook Health Sciences Centre, University of Toronto, Toronto, Ontario, Canada
- 7 Institute of Health Policy Management and Evaluation, University of Toronto, Toronto, Ontario, Canada
- 8 Department of Cardiology, St Antonius Hospital, Nieuwegein, the Netherlands
- 9 Division of Cardiac Surgery, Boca Raton Regional Hospital and Florida Atlantic Hospital, Boca Raton
- 10 Zena and Michael A. Wiener Cardiovascular Institute, Icahn School of Medicine at Mount Sinai, New York, New York
- 11 Division of Cardiac Surgery, University of Ottawa Heart Institute, Ottawa, Ontario, Canada
- 12 Division of Cardiology, Vancouver General Hospital, British Columbia, Canada
- 13 Division of Cardiology St Paul’s Hospital, University of British Columbia, Vancouver, Canada
- 14 Ruijin Hospital Shanghai Jiao Tong University School of Medicine, Shanghai, China
- Editorial Risks and Benefits of Antiplatelet Therapy After Coronary Artery Bypass Surgery Sunil V. Rao, MD; E. Magnus Ohman, MD JAMA
- Comment & Response Dual Antiplatelet Therapy With Ticagrelor and Vein Graft Failure After Coronary Artery Bypass Graft Surgery Sigrid Sandner, MD; Björn Redfors, MD, PhD; Mario Gaudino, MD, PhD JAMA
- Comment & Response Dual Antiplatelet Therapy With Ticagrelor and Vein Graft Failure After Coronary Artery Bypass Graft Surgery Bing-Yan Zeng, MD; Ping-Tao Tseng, MD, PhD; Chih-Sung Liang, MD JAMA
Question Is ticagrelor dual antiplatelet therapy (DAPT) for patients undergoing coronary artery bypass graft surgery associated with differences in vein graft failure and bleeding events compared with aspirin?
Findings In this individual patient data meta-analysis that included 4 randomized clinical trials, 1316 patients and 1668 vein grafts, ticagrelor DAPT compared with aspirin was associated with a significantly lower incidence of vein graft failure (11.2% vs 20.0%) and a significantly higher incidence of Bleeding Academic Research Consortium type 2, 3, or 5 bleeding events (22.1% vs 8.7%).
Meaning In patients undergoing coronary artery bypass graft surgery, adding ticagrelor to aspirin was associated with a significantly decreased risk of vein graft failure, as well as a significantly increased risk of clinically important bleeding.
Importance The role of ticagrelor with or without aspirin after coronary artery bypass graft surgery remains unclear.
Objective To compare the risks of vein graft failure and bleeding associated with ticagrelor dual antiplatelet therapy (DAPT) or ticagrelor monotherapy vs aspirin among patients undergoing coronary artery bypass graft surgery.
Data Sources MEDLINE, Embase, and Cochrane Library databases from inception to June 1, 2022, without language restriction.
Study Selection Randomized clinical trials (RCTs) comparing the effects of ticagrelor DAPT or ticagrelor monotherapy vs aspirin on saphenous vein graft failure.
Data Extraction and Synthesis Individual patient data provided by each trial were synthesized into a combined data set for independent analysis. Multilevel logistic regression models were used.
Main Outcomes and Measures The primary analysis assessed the incidence of saphenous vein graft failure per graft (primary outcome) in RCTs comparing ticagrelor DAPT with aspirin. Secondary outcomes were saphenous vein graft failure per patient and Bleeding Academic Research Consortium (BARC) type 2, 3, or 5 bleeding events. A supplementary analysis included RCTs comparing ticagrelor monotherapy with aspirin.
Results A total of 4 RCTs were included in the meta-analysis, involving 1316 patients and 1668 saphenous vein grafts. Of the 871 patients in the primary analysis, 435 received ticagrelor DAPT (median age, 67 years [IQR, 60-72 years]; 65 women [14.9%]; 370 men [85.1%]) and 436 received aspirin (median age, 66 years [IQR, 61-73 years]; 63 women [14.5%]; 373 men [85.5%]). Ticagrelor DAPT was associated with a significantly lower incidence of saphenous vein graft failure (11.2%) per graft than was aspirin (20%; difference, −8.7% [95% CI, −13.5% to −3.9%]; OR, 0.51 [95% CI, 0.35 to 0.74]; P < .001) and was associated with a significantly lower incidence of saphenous vein graft failure per patient (13.2% vs 23.0%, difference, −9.7% [95% CI, −14.9% to −4.4%]; OR, 0.51 [95% CI, 0.35 to 0.74]; P < .001). Ticagrelor DAPT (22.1%) was associated with a significantly higher incidence of BARC type 2, 3, or 5 bleeding events than was aspirin (8.7%; difference, 13.3% [95% CI, 8.6% to 18.0%]; OR, 2.98 [95% CI, 1.99 to 4.47]; P < .001), but not BARC type 3 or 5 bleeding events (1.8% vs 1.8%, difference, 0% [95% CI, −1.8% to 1.8%]; OR, 1.00 [95% CI, 0.37 to 2.69]; P = .99). Compared with aspirin, ticagrelor monotherapy was not significantly associated with saphenous vein graft failure (19.3% vs 21.7%, difference, −2.6% [95% CI, −9.1% to 3.9%]; OR, 0.86 [95% CI, 0.58 to 1.27]; P = .44) or BARC type 2, 3, or 5 bleeding events (8.9% vs 7.3%, difference, 1.7% [95% CI, −2.8% to 6.1%]; OR, 1.25 [95% CI, 0.69 to 2.29]; P = .46).
Conclusions and Relevance Among patients undergoing coronary artery bypass graft surgery, adding ticagrelor to aspirin was associated with a significantly decreased risk of vein graft failure. However, this was accompanied by a significantly increased risk of clinically important bleeding.
Saphenous vein grafts are the most frequently used conduits in coronary artery bypass graft (CABG) surgery, yet as many as 10% to 25% occlude within the first year after surgery. 1 , 2 Early saphenous vein graft failure is mainly due to thrombosis subsequent to endothelial damage or endothelial activation leading to a prothrombotic phenotype. 2 , 3 Inhibition of platelet aggregation with aspirin after CABG surgery has been shown to reduce early saphenous vein graft failure and is endorsed in current practice guidelines. 4 - 6 Dual antiplatelet therapy (DAPT), consisting of aspirin and an oral platelet P2Y12 receptor inhibitor, is associated with enhanced platelet inhibitory effects. 7 Although DAPT is the guideline-recommended treatment after percutaneous coronary revascularization, 7 considerable controversy exists as to the benefit of DAPT for patients after CABG surgery. Studies comparing ticagrelor DAPT with aspirin have yielded conflicting results, 8 - 10 and the few studies comparing ticagrelor monotherapy with aspirin 9 , 11 failed to demonstrate an effect of ticagrelor monotherapy on saphenous vein graft failure; however, they were individually underpowered.
A systematic review and individual patient data meta-analysis of all randomized clinical trials (RCTs) comparing the effects of ticagrelor DAPT or ticagrelor monotherapy with aspirin on saphenous vein graft failure among patients undergoing CABG surgery was performed.
This study design was published a priori on the International Prospective Register of Systematic Reviews ( CRD42021291997 ). The statistical analysis protocol was prespecified to reduce post hoc bias. The analysis was performed in accordance with the Individual Patient Data-Preferred Reporting Items for Systematic Reviews and Meta-Analyses (IPD-PRISMA). 12 The PRISMA checklist was followed. Ethics approval and patient consent were obtained locally by each trial team. The Weill Cornell Medicine Institutional Review Board waived the need for ethics approval for the pooled analysis (protocol 22-03024559).
A medical librarian searched Ovid MEDLINE, Ovid Embase, and the Cochrane Central (Wiley) databases to identify RCTs published between database inception and June 1, 2022, comparing ticagrelor DAPT and/or ticagrelor monotherapy with aspirin in patients undergoing CABG surgery who had follow-up for graft imaging. No language restrictions were imposed. The full search strategy is provided in the Supplement (eMethods 1 in the Supplement ). Identification of studies meeting the search criteria was performed by 2 authors (S.S. and K.A.). Conflicts over inclusion were resolved by consultation with a third author (M.G.).
The principal investigators of the eligible trials were contacted and all agreed to share individual patient data. Specifications of core minimum deidentified data requirements were provided to each trial (eMethods 2 in the Supplement ). Data received from the individual trial teams by the analysis unit at Weill Cornell Medicine were checked for completeness and consistency with previous publications. Discrepancies were resolved directly with the trial investigators. For harmonization of graft failure definition across trials, occlusion and/or percent stenosis per graft or anastomosis (for sequential grafts) were provided by each trial team. Events were readjudicated centrally. For harmonization of bleeding outcomes, bleeding events were readjudicated by each trial team according to Bleeding Academic Research Consortium (BARC) criteria. 13 All analyses were performed independently on the combined data set of individual patient data provided for each trial. The risk of bias was assessed using the Cochrane risk-of-bias tool 2 14 (eFigure 1 in the Supplement ).
The primary outcome was the incidence of saphenous vein graft failure, defined as saphenous vein graft occlusion or stenosis greater than 50% per graft as assessed by either invasive angiography or computed tomographic angiography at the individual trial protocol–defined follow-up. Secondary outcomes were the incidence of saphenous vein graft failure per patient (defined as patients with ≥1 failed saphenous vein graft); the incidence of BARC type 2, 3, or 5 bleeding events; the composite of saphenous vein graft failure or cardiovascular death; and major adverse cardiac and cerebrovascular events (MACCE, defined as the composite of all-cause death, myocardial infarction, stroke, or revascularization). Definitions of events in the individual trials are provided in eTable 1 in the Supplement .
Post hoc outcomes were the incidence of saphenous vein graft occlusion per graft; any graft failure (arterial or saphenous vein grafts); BARC type 2 through 5, 3 through 5, and 3 or 5 bleeding events; the individual components of MACCE; major adverse cardiovascular events (MACE, defined as the composite of cardiovascular death, myocardial infarction, or stroke); and net adverse events (defined as graft failure [arterial or saphenous vein grafts] or BARC type ≥3 bleeding event), net adverse major clinical events (defined as all-cause death, myocardial infarction, stroke, or BARC type ≥3 bleeding event), and overall net adverse events (defined as graft failure, MACCE, or BARC type ≥2 bleeding events).
Baseline categorical variables are reported as counts and percentages. Continuous variables are reported as medians and IQRs. The primary analysis was performed according to randomization group and compared ticagrelor DAPT with aspirin. The primary analysis set was patients with saphenous vein grafts who were randomized to ticagrelor DAPT or aspirin and for whom protocol-defined imaging was available. The primary outcome was evaluated using a multilevel logistic regression model using the GLIMMIX procedure 15 that accounted for clustering of patients within trials and clustering of grafts within patients. Treatment associations are reported as odds ratios (ORs) and 95% CIs.
Secondary outcomes were evaluated using a multilevel logistic regression model with the trial as a random effect (reported as OR and 95% CI) or a Cox proportional hazards frailty model with trial as a random effect (reported as hazard ratio [HR] and 95% CI). Event rates were calculated using the Kaplan-Meier method. The proportional hazards assumption was confirmed for each end point by using Schoenfeld residuals and visual inspection of the Schoenfeld residuals, Kaplan-Meier plots, and log-log plots.
Sensitivity analyses for the primary outcome assessed the incidence of saphenous vein graft failure per anastomosis and in patients who had 1-year imaging. Sensitivity models for the primary outcome were performed in the as-treated population (according to treatment received) and per-protocol population (according to whether treatment was received in compliance with the trial protocol), and after imputation of missing data by multiple imputation (assuming a joint multivariate normal distribution for all variables and imputing 20 data sets). These sensitivity models were adjusted for baseline and procedure-related confounders that included age, sex, clinical presentation, smoking, diabetes, hypertension, hyperlipidemia, prior myocardial infarction, chronic kidney disease, use of cardiopulmonary bypass, endoscopic saphenous vein graft harvesting, and sequential saphenous vein grafting.
Prespecified subgroup analyses for the primary outcome were age, sex, diabetes, smoking, acute coronary syndrome, use of cardiopulmonary bypass, endoscopic saphenous vein graft harvesting, target vessel territory, use of sequential saphenous vein grafts, and treatment duration. For subgroup analyses, an interaction-term between the treatment and the subgroup of interest was included in the logistic regression model.
A supplementary analysis for the primary outcome compared ticagrelor monotherapy with aspirin.
Details on post hoc analyses are provided in eMethods 3 in the Supplement . A post hoc random-effects network meta-analysis was performed to compare the associations of ticagrelor DAPT, ticagrelor monotherapy, and aspirin with saphenous vein graft failure.
A 2-sided P value of <.05 was considered significant for all tests. There was no adjustment for multiplicity. Because of the potential for type I error due to multiple comparisons, findings for analyses of secondary end points should be interpreted as exploratory. All analyses were performed using SAS version 9.4 (SAS Institute Inc) except for the network meta-analysis, which was performed using R version 4.1.0. 16
The literature search yielded 776 results, of which 557 were screened for eligibility. Four trials meeting the inclusion criteria were included in the analysis: Ticagrelor and Aspirin for the Prevention of Cardiovascular Events after Coronary Artery Bypass Graft Surgery (TAP-CABG), 8 Different Antiplatelet Therapy Strategy after Coronary Artery Bypass Graft Surgery (DACAB), 9 Effect of Ticagrelor on Saphenous Vein Graft Patency in Patients undergoing Coronary Artery Bypass Grafting Surgery (Popular CABG), 10 and Ticagrelor Antiplatelet Therapy to Reduce Graft Events and Thrombosis (TARGET). 11 The PRISMA IPD flow diagram is provided in eFigure 2 in the Supplement .
An overview of the included trials is provided in Table 1 . Two trials 8 , 10 compared ticagrelor DAPT with aspirin, 1 trial 11 compared ticagrelor monotherapy with aspirin, and 1 trial 9 compared ticagrelor DAPT and ticagrelor monotherapy with aspirin. All trials used a 90-mg twice-daily regimen of ticagrelor. A total of 3079 grafts (1668 saphenous vein grafts and 1411 arterial grafts) in 1316 patients were included in the meta-analysis.
The primary analysis included 871 patients from the TAP-CABG, 8 DACAB, 9 and POPular CABG 10 trials. A total of 435 patients (49.9%; 527 saphenous vein grafts) were randomized to ticagrelor DAPT, and 436 patients (50.1%; 537 saphenous vein grafts) were randomized to aspirin ( Table 2 ). The median treatment duration was 365 days (IQR, 307-365 days) for patients in the ticagrelor DAPT group and 364 days (IQR, 315-365 days) for patients in the aspirin group. A total of 394 patients (90.6%) in the ticagrelor DAPT group and 400 patients (91.7%) in the aspirin group underwent protocol-defined imaging (eTables 2-4 in the Supplement ). Protocol-defined imaging was performed by computed tomographic angiography in 789 patients and coronary angiography in 5 patients. The median time from CABG surgery to imaging was 369 days (IQR, 364-375 days) in the ticagrelor DAPT group and 370 days (IQR, 364-376 days) in the aspirin group.
The primary outcome of saphenous vein graft failure occurred in 11.2% (54 of 481) of saphenous vein grafts in the ticagrelor DAPT group and in 20.0% (99 of 494) of saphenous vein grafts in the aspirin group (difference, −8.7% [95% CI, −13.5% to −3.9%], OR, 0.51 [95% CI, 0.35 to 0.74]; P < .001; Figure 1 A).
When assessed per patient, saphenous vein graft failure occurred in 13.2% (52 of 394) of patients in the ticagrelor DAPT group and 23.0% (92 of 400) of patients in the aspirin group (difference, −9.7% [95% CI, −14.9% to −4.4%]; OR, 0.51 [95% CI, 0.35 to 0.74]; P < .001; Figure 1 B).
Ticagrelor DAPT was associated with a significantly higher risk of BARC type 2, 3, or 5 bleeding events compared with aspirin (22.1% vs 8.7%, difference; 13.3% [95% CI, 8.6% to 18.0%]; OR, 2.98 [95% CI, 1.99 to 4.47]; P < .001) ( Figure 2 A; eFigure 3 in the Supplement ). Ticagrelor DAPT was associated with a significantly lower risk of the composite of saphenous vein graft failure or cardiovascular death compared with aspirin (13.9% vs 23.4%: difference, −9.4% [95% CI, −14.7% to −4.1%]; OR, 0.52 [95% CI, 0.36 to 0.76]; P < .001) but was not significantly associated with lower risk of MACCE (6.7% vs 5.5%; difference, 1.2% [95% CI, −2.0% to 4.3%]; HR, 1.21 [95% CI, 0.70 to 2.08]; P = .50; Figure 2 B).
The results for the primary outcome were confirmed in the sensitivity analysis for saphenous vein graft failure per anastomosis (ticagrelor DAPT vs aspirin, 8.6% vs 14.6%; difference, −6.1% [95% CI, −9.9% to −2.4%]; OR, 0.54 [95% CI, 0.37 to 0.80]; P < .001; eFigure 4 in the Supplement ) and in the sensitivity analysis that included only patients with protocol-defined imaging at 1 year (eFigure 5 in the Supplement ). Sensitivity analyses for the primary outcome in the as-treated and per-protocol populations and after imputation of missing data were also consistent with the main analysis (eTable 5 in the Supplement ).
The association of ticagrelor DAPT with the risk of saphenous vein graft failure was consistent across all prespecified subgroups ( Figure 3 ).
Ticagrelor DAPT was associated with a significantly lower risk of saphenous vein graft occlusion than was aspirin (9.6% vs 16.2%; difference, −6.6% [95% CI, −11.0% to −2.2%]; OR, 0.55 [95% CI, 0.37 to 0.82]; P = .003) (eFigure 6 in the Supplement ).
The results of the post hoc analyses for any graft failure were consistent with the main analysis (eTable 6 in the Supplement ). The association of ticagrelor DAPT with any graft failure remained consistent when stratified by graft type and target vessel territory (eFigure 7 in the Supplement ).
The association of ticagrelor DAPT with bleeding events is shown in Figure 2 and eFigure 3 in the Supplement . There were no instances of BARC type 5 bleeding events. The association of ticagrelor DAPT with the risk of BARC type 2, 3, or 5 bleeding events was consistent across important clinical subgroups (eFigure 8 in the Supplement ). Ticagrelor DAPT was not associated with significant differences in the individual components of MACCE or MACE ( Figure 2 ).
Ticagrelor DAPT was associated with a significantly lower risk of net adverse events than was aspirin (17.0% vs 27.8%; difference, −10.6% [95% CI, −16.3% to −4.9%]; OR, 0.53 [95% CI, 0.38 to 0.75]; P < .001) but was not associated with significant differences in net adverse major clinical events or overall net adverse events (eTable 7 in the Supplement ).
In the network meta-analysis with aspirin as the reference group, ticagrelor DAPT was associated with a significantly lower risk of saphenous vein graft failure per graft (OR, 0.49 [95% CI, 0.27 to 0.87]) whereas ticagrelor monotherapy was not (OR, 0.94 [95% CI, 0.51 to 1.74]; I 2 = 55.4%; eFigure 9 in the Supplement ).
Ticagrelor monotherapy was not associated with a significant difference in saphenous vein graft failure compared with aspirin per graft (19.3% vs 21.7%; difference, −2.6% [95% CI, −9.1% to 3.9%]; OR, 0.86 [95% CI, 0.58 to 1.27]; P = .44) or per patient (25.2% vs 29.3%; difference, −4.1% [95% CI, −11.9% to 3.7%]; OR, 0.81 [95% CI, 0.55 to 1.20]; P = .30; eTable 8 in the Supplement ). There was no significant difference between ticagrelor monotherapy and aspirin in the association with BARC type 2, 3, or 5 bleeding events (8.9% vs 7.3%; difference, 1.7%; [95% CI, −2.8% to 6.1%]; OR, 1.25 [95% CI, 0.69 to 2.29]; P = .46; eTable 9 in the Supplement ).
In this individual patient data meta-analysis of 4 RCTs including 1316 patients and 1668 saphenous vein grafts, ticagrelor DAPT was associated with a significantly lower risk of saphenous vein graft failure and a significantly higher risk of clinically important bleeding events than was aspirin.
CABG surgery is the treatment of choice for patients with high-complexity coronary artery disease and those with reduced left ventricular ejection fraction. 6 In the US alone approximately 300 000 patients undergo CABG surgery annually. 17 The saphenous vein is used in more than 90% of CABG procedures. 18
RCTs investigating the effect of ticagrelor DAPT vs aspirin on saphenous vein graft failure have reported conflicting results. In the DACAB trial, 9 ticagrelor DAPT significantly increased saphenous vein graft patency 1 year after CABG. In contrast, in the POPular CABG trial, 10 ticagrelor DAPT did not significantly reduce saphenous vein graft occlusion 1 year after CABG surgery. The TAP-CABG trial 8 also did not find a significant difference in the absolute risk of saphenous vein graft occlusion between ticagrelor DAPT and aspirin 3 months after CABG.
In addition, the published RCTs were all individually underpowered to detect even moderate differences in bleeding outcomes. Although they did not individually report an increase in major bleeding events with ticagrelor DAPT, 8 - 10 a solid estimate of the risk to benefit ratio of ticagrelor DAPT after CABG surgery was not possible.
An aggregate network meta-analysis of 20 RCTs that assessed the effects of oral antithrombotic drugs and included 2 RCTs (203 patients) comparing ticagrelor DAPT with aspirin did not show a significant difference in major bleeding events between the groups (OR, 1.93 [95% CI, 0.30-12.4]). 19 In another meta-analysis of 5 RCTs and 3996 patients, there was no significant difference in the risk of bleeding between ticagrelor–based antiplatelet therapy vs aspirin and/or clopidogrel (relative risk, 1.04 [95% CI, 0.95-1.14]; P = .41). 20 However, individual studies used different bleeding definitions and this greatly limits the clinical relevance of the results from trial-level meta-analyses.
In the present work, graft failure and bleeding events were readjudicated using a common definition before pooling, allowing generation of homogeneous pooled estimates of the association of ticagrelor DAPT with saphenous vein graft failure and bleeding events.
This comprehensive synthesis of all RCTs with angiographic follow-up provides solid evidence that ticagrelor DAPT is associated with a significantly lower risk of saphenous vein graft failure 1 year after CABG surgery. The association of ticagrelor DAPT with the risk of saphenous vein graft failure was consistent across subgroups. However, compared with aspirin, ticagrelor DAPT was also associated with a significantly higher risk of BARC type 2 or higher bleeding events. There was also an absolute increase in the risk of all-cause death in the ticagrelor DAPT group, although not statistically significant. Taken together, the present analysis suggests that a patient’s individual risk of graft failure, ischemic events, and bleeding needs to be weighed carefully when deciding whether to add ticagrelor to aspirin after CABG surgery. Longer-term follow-up is required to fully evaluate a potential benefit of ticagrelor DAPT on clinical events. 21
Individual RCTs investigating the effect of ticagrelor monotherapy vs aspirin after CABG surgery have not shown an effect on saphenous vein graft failure but have all been limited by the small sample size. 9 , 11 In this meta-analysis, ticagrelor monotherapy was not associated with a significant difference in the risk of saphenous vein graft failure compared with aspirin, but the pooled estimate was compatible with a potential benefit of ticagrelor monotherapy. Because ticagrelor monotherapy was not associated with a significant difference in the risk of bleeding events compared with aspirin, its role as a treatment option after CABG surgery requires further investigation.
This study has several limitations. First, the pooled analysis is subject to the limitations of the original RCTs, including the open-label treatment allocation in 1 of them, although outcome adjudication was blinded in all RCTs. Second, there was heterogeneity in surgical technique and postoperative management across the included RCTs. Third, the duration of ticagrelor DAPT and timing of follow-up ranged from 3 to 12 months across the included RCTs. Fourth, the analysis was not designed to evaluate differences in clinical outcomes between groups. Lastly, protocol-directed imaging was missing in 9.1% of patients. However, patient characteristics were balanced across randomization groups for patients who underwent imaging, and the results for the primary outcome were consistent after multiple imputation of missing data.
In patients undergoing coronary artery bypass graft surgery, adding ticagrelor to aspirin was associated with a significantly decreased risk of vein graft failure. However, this was accompanied by a significantly increased risk of clinically important bleeding.
Corresponding Author: Mario Gaudino, MD, PhD, Department of Cardiothoracic Surgery, Weill Cornell Medicine, New York Presbyterian Hospital, 525 E 68th St, New York, NY 10065 ( [email protected] ).
Accepted for Publication: June 23,2022.
Author Contributions : Drs Sandner and Redfors had full access to all of the data in the study and take responsibility for the integrity of the data and the accuracy of the data analysis. Drs Sandner and Redfors contributed equally.
Concept and design: Sandner, Fremes, Mehran, Peper, Ruel, Zhao, Zhu, Gaudino.
Acquisition, analysis, or interpretation of data: Sandner, Redfors, Angiolillo, Audisio, Fremes, Janssen, Kulik, Peper, Ruel, Saw, Soletti, Starovoytov, ten Berg, Willemsen, Gaudino.
Drafting of the manuscript: Sandner, Redfors, Kulik, Gaudino.
Critical revision of the manuscript for important intellectual content: All authors.
Statistical analysis: Redfors, Kulik, Gaudino.
Obtained funding: Janssen, Ruel.
Administrative, technical, or material support: Janssen, Ruel, Saw, Soletti, Starovoytov.
Supervision: Angiolillo, Janssen, Soletti, Starovoytov, ten Berg, Willemsen, Gaudino.
Other - protocol review: Willemsen.
Conflict of Interest Disclosures: Dr Sandner reported receiving institutional research grants from Vascular Grafts Solutions outside the submitted work. Dr Angiolillo reported receiving consulting fees or honoraria from Abbott, Amgen, AstraZeneca, Bayer, Biosensors, Boehringer Ingelheim, Bristol Myers Squibb, Chiesi, Daiichi-Sankyo, Eli Lilly, Haemonetics, Janssen, Merck, PhaseBio, PLx Pharma, Pfizer, and Sanofi; and research grants to his institution from Amgen, AstraZeneca, Bayer, Biosensors, CeloNova, CSL Behring, Daiichi-Sankyo, Eisai, Eli Lilly, Gilead, Janssen, Matsutani Chemical Industry Co, Merck, Novartis, Osprey Medical, Renal Guard Solutions, and the Scott R. MacKenzie Foundation. Dr Janssen reported receiving grants from AstraZeneca funding for the POPular CABG trial during the conduct of the study. Dr Kulik reported receiving grants from AstraZeneca during the conduct of the study. Dr Mehran reported receiving research grants from Abbott, Abiomed, Alleviant Medical, AM-Pharma, Applied Therapeutics, Arena, AstraZeneca, Bayer, Beth Israel Deaconess, Biosensors, Biotronik, Bristol Myers Squibb, Boston Scientific Research, CardiaWave Research, CellAegis, CeloNova, CERC, Chiesi, Concept Medical, CSL Behring, Daiichi Sankyo Inc, Duke University, Humacyte, Idorsa Pharmaceuticals, Insel Gruppe AG, Janssen, Medtronic, Novartis, OrbusNeich, Philips, Vivasure, and Zoll, all to her institution; serving as a consultant to the California Institute for Regenerative Medicine; serving on the scientific advisory board of the American Medical Association; serving on the advisory boards of CERC (Biosnsors), Abbott, Arena, Biotronik, CardiaWave, Chiesi, Concept Medical, Humacyte, Magenta, Novartis, and Philips, all to her institution; serving on the board of the American College of Cardiology, and as a committee member of the Women in Innovations of the Society for Cardiovascular Angiography, all to her institution; serving as an unpaid faculty of the Cardiovascular Research Foundation; receiving personal fees from Cine-Med, Janssen, and WebMD; receiving less than 1% of equity in Applied Therapeutics Equity, Elixir Medical, Stel, and ControlRad Equity (spouse) outside the submitted work; and having other financial or nonfinancial interests in Boston Scientific Corp and divested final stock options of less than 1% in Claret Medical. Dr Saw reported receiving grants from AstraZeneca outside the submitted work and serving as the principal investigator for the TAP-CABG study. Dr ten Berg reported receiving speaker fees from AstraZeneca, Bayer, Boehringer Ingelheim, and Celecor and institutional research grants from AstraZeneca and ZonMw. Dr Willemsen reported receiving grants from AstraZeneca funding of the POPular CABG trial during the conduct of the study. Dr Zhao reported receiving grants from AstraZeneca during the conduct of the study and from Chugai Pharma China outside the submitted work. Dr Zhu reported receiving personal fees from Chugai Pharma China outside the submitted work. No other disclosures were reported.
Funding/Support: This study was supported using internal funds from the Department of Cardiothoracic Surgery at Weill Cornell Medicine, New York.
Role of the Funder/Sponsor: The funder had no role in the design and conduct of the study; collection, management, analysis, and interpretation of the data; preparation, review, or approval of the manuscript; and decision to submit the manuscript for publication.
- Register for email alerts with links to free full-text articles
- Access PDFs of free articles
- Manage your interests
- Save searches and receive search alerts
- Future Students
- Current Students
- Faculty/Staff

News and Media
- News & Media Home
- Research Stories
- School's In
- In the Media
You are here
70 years after brown v. board of education, new research shows rise in school segregation.

As the nation prepares to mark the 70th anniversary of the landmark U.S. Supreme Court ruling in Brown v. Board of Education , a new report from researchers at Stanford and USC shows that racial and economic segregation among schools has grown steadily in large school districts over the past three decades — an increase that appears to be driven in part by policies favoring school choice over integration.
Analyzing data from U.S. public schools going back to 1967, the researchers found that segregation between white and Black students has increased by 64 percent since 1988 in the 100 largest districts, and segregation by economic status has increased by about 50 percent since 1991.
The report also provides new evidence about the forces driving recent trends in school segregation, showing that the expansion of charter schools has played a major role.
The findings were released on May 6 with the launch of the Segregation Explorer , a new interactive website from the Educational Opportunity Project at Stanford University. The website provides searchable data on racial and economic school segregation in U.S. states, counties, metropolitan areas, and school districts from 1991 to 2022.
“School segregation levels are not at pre- Brown levels, but they are high and have been rising steadily since the late 1980s,” said Sean Reardon , the Professor of Poverty and Inequality in Education at Stanford Graduate School of Education and faculty director of the Educational Opportunity Project. “In most large districts, school segregation has increased while residential segregation and racial economic inequality have declined, and our findings indicate that policy choices – not demographic changes – are driving the increase.”
“There’s a tendency to attribute segregation in schools to segregation in neighborhoods,” said Ann Owens , a professor of sociology and public policy at USC. “But we’re finding that the story is more complicated than that.”
Assessing the rise
In the Brown v. Board decision issued on May 17, 1954, the U.S. Supreme Court ruled that racially segregated public schools violated the Equal Protection Clause of the Fourteenth Amendment and established that “separate but equal” schools were not only inherently unequal but unconstitutional. The ruling paved the way for future decisions that led to rapid school desegregation in many school districts in the late 1960s and early 1970s.
Though segregation in most school districts is much lower than it was 60 years ago, the researchers found that over the past three decades, both racial and economic segregation in large districts increased. Much of the increase in economic segregation since 1991, measured by segregation between students eligible and ineligible for free lunch, occurred in the last 15 years.
White-Hispanic and white-Asian segregation, while lower on average than white-Black segregation, have both more than doubled in large school districts since the 1980s.
Racial-economic segregation – specifically the difference in the proportion of free-lunch-eligible students between the average white and Black or Hispanic student’s schools – has increased by 70 percent since 1991.
School segregation is strongly associated with achievement gaps between racial and ethnic groups, especially the rate at which achievement gaps widen during school, the researchers said.
“Segregation appears to shape educational outcomes because it concentrates Black and Hispanic students in higher-poverty schools, which results in unequal learning opportunities,” said Reardon, who is also a senior fellow at the Stanford Institute for Economic Policy Research and a faculty affiliate of the Stanford Accelerator for Learning .
Policies shaping recent trends
The recent rise in school segregation appears to be the direct result of educational policy and legal decisions, the researchers said.
Both residential segregation and racial disparities in income declined between 1990 and 2020 in most large school districts. “Had nothing else changed, that trend would have led to lower school segregation,” said Owens.
But since 1991, roughly two-thirds of districts that were under court-ordered desegregation have been released from court oversight. Meanwhile, since 1998, the charter sector – a form of expanded school choice – has grown.
Expanding school choice could influence segregation levels in different ways: If families sought schools that were more diverse than the ones available in their neighborhood, it could reduce segregation. But the researchers found that in districts where the charter sector expanded most rapidly in the 2000s and 2010s, segregation grew the most.
The researchers’ analysis also quantified the extent to which the release from court orders accounted for the rise in school segregation. They found that, together, the release from court oversight and the expansion of choice accounted entirely for the rise in school segregation from 2000 to 2019.
The researchers noted enrollment policies that school districts can implement to mitigate segregation, such as voluntary integration programs, socioeconomic-based student assignment policies, and school choice policies that affirmatively promote integration.
“School segregation levels are high, troubling, and rising in large districts,” said Reardon. “These findings should sound an alarm for educators and policymakers.”
Additional collaborators on the project include Demetra Kalogrides, Thalia Tom, and Heewon Jang. This research, including the development of the Segregation Explorer data and website, was supported by the Russell Sage Foundation, the Robert Wood Johnson Foundation, and the Bill and Melinda Gates Foundation.
More Stories
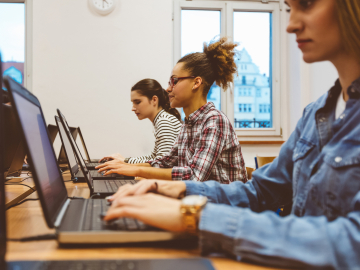
⟵ Go to all Research Stories
Get the Educator
Subscribe to our monthly newsletter.
Stanford Graduate School of Education
482 Galvez Mall Stanford, CA 94305-3096 Tel: (650) 723-2109
- Contact Admissions
- GSE Leadership
- Site Feedback
- Web Accessibility
- Career Resources
- Faculty Open Positions
- Explore Courses
- Academic Calendar
- Office of the Registrar
- Cubberley Library
- StanfordWho
- StanfordYou
Improving lives through learning

- Stanford Home
- Maps & Directions
- Search Stanford
- Emergency Info
- Terms of Use
- Non-Discrimination
- Accessibility
© Stanford University , Stanford , California 94305 .
Cookies on GOV.UK
We use some essential cookies to make this website work.
We’d like to set additional cookies to understand how you use GOV.UK, remember your settings and improve government services.
We also use cookies set by other sites to help us deliver content from their services.
You have accepted additional cookies. You can change your cookie settings at any time.
You have rejected additional cookies. You can change your cookie settings at any time.

- Entering and staying in the UK
- Visas and entry clearance
Analysis of migrants use of the Graduate route
- Home Office
Published 14 May 2024
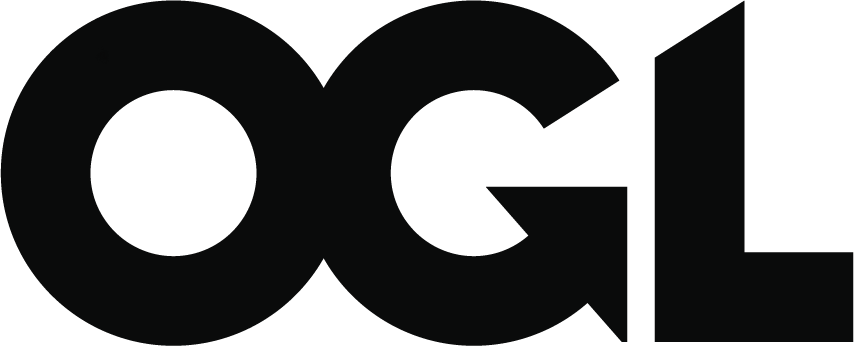
© Crown copyright 2024
This publication is licensed under the terms of the Open Government Licence v3.0 except where otherwise stated. To view this licence, visit nationalarchives.gov.uk/doc/open-government-licence/version/3 or write to the Information Policy Team, The National Archives, Kew, London TW9 4DU, or email: [email protected] .
Where we have identified any third party copyright information you will need to obtain permission from the copyright holders concerned.
This publication is available at https://www.gov.uk/government/statistics/analysis-of-migrants-use-of-the-graduate-route/analysis-of-migrants-use-of-the-graduate-route
1. Introduction
This report looks at the journeys of foreign nationals who came to study in the UK, with a particular focus on those entering and leaving the Graduate route. It looks at who is entering the Graduate route, what they do after their Graduate leave expires, and if they extended into other leave. Additionally, it includes early insights on their earnings and employment by linking Home Office visa records to HMRC earnings data.
This report uses data that underpins the Home Office migrant journey statistical publications. The next full update of the Migrant journey covering 2023 is due to be published on 23 May 2024.
The report seeks to complement the Migration Advisory Committee’s (MAC’s) rapid review of the Graduate route published on 14 May 2024.
The report defines a migrant journey as a series of grants of leave to an individual where each grant is no more than 12 months after the expiry of the previous grant. As such, the number of new journeys in a given year within the report will not match the total number of overseas visa grants in the same period as published in the Immigration system statistics.
Overview of the Graduate route
The Graduate route was introduced in July 2021 and allows foreign students who have successfully completed a UK bachelor’s degree, postgraduate degree or other eligible course to stay in the UK for at least 2 years. Graduate visa holders can work or look for work during this period and may switch to another immigration route at any point.
It is too early to say whether the behaviours of the early adopters of the scheme will be indicative of the behaviours of later cohorts. This also applies when looking at Graduate visa holders’ earnings.
2. The Graduate Journey
What are study visa holders doing after their studies?
The proportion of students granted further leave to remain in the UK following their studies more than tripled between 2019 and 2023, from 18% to 56%.
Over half (56%) the number of students who came to the end of their studies in 2023 had further leave to remain in the UK, mostly on the Graduate route (32%) and other work routes (18%).
Figure 1: Students who came to the end of their studies by subsequent leave category
Source: Migrant journey underlying datasets, Home Office
- ‘Other work’ includes all work routes excluding the Graduate route. For example ‘Skilled Worker’ and ‘Skilled Worker - Health and Care’ visas.
- ‘Other leave’ includes all non-work routes.
- The numbers at the top of the bars are the total number of people leaving the student route in each year. This includes people whose leave came to end, and people who switched onto another route before their leave expired.
Figure 1 shows the proportion of students who remained in the UK after their studies by switching to another type of leave was between 15% and 20% in 2019 and 2020. This proportion has since increased reaching 52% in 2022 and 56% in 2023. In addition, the number of people leaving the student route increased in each year since 2019 (from 160,104 in 2019 to 350,365 in 2023), so both the number and proportion of students remaining in the UK beyond their studies has increased. While more students appear to be extending their stay by making use of the Graduate visa and other routes, we do not yet know if they will remain in the UK permanently, or whether they are just staying longer.
Before 2021, between 80% and 85% of students held no valid leave to remain in the UK following the expiry of their student leave. This proportion has fallen since 2020, reaching 44% in 2023. This period saw a number of different factors which may have affected the proportion of foreign students remaining in the UK, including the COVID-19 pandemic, the introduction of the Graduate route, and the ending of freedom of movement for EU nationals. It is difficult to unpick the extent to which these factors are impacting recent trends, however 2022 was the first year on record where more than half of student leavers were granted further leave.
Who is on the Graduate route?
The latest Immigration system statistics extensions data shows that 213,250 main applicants and 45,836 dependants were granted Graduate visas between its launch in July 2021 and the end of 2023.
Figure 2: Graduate route visas granted by year and applicant type
Source: Immigration system statistics; Extensions detailed datasets, table Exe_D01
The top 5 nationalities account for almost three-quarters (74%) of Graduate visas issued to main applicants with Indian nationals accounting for 42%.
Table 1: Top 5 nationalities granted Graduate visas (main applicants)
Table 1 shows that Indian (42%), Nigerian (11%) and Chinese (10%) nationals accounted for two-thirds of those entering the Graduate route in 2023. Indian students were proportionally more likely to switch to the Graduate route, accounting for 42% of Graduate visa grants but only 23% of Student visa expiries between 2021 and 2023; while Chinese students were proportionally less likely, accounting for only 10% of Graduate visa grants but 30% of Student visa expiries.
Those entering the Graduate route tend to be in their mid-to-late 20s, with more than half (58%) being between the ages of 24 and 29. Slightly more (52%) of the 2023 graduate entrants were male than female (48%).
113,105 students switched to the Graduate route in 2023; 69% of these students had been studying for one year or less.
Figure 3: Graduate route entrants by length of study leave between 2021 and 2023
Source: Migrant Journey underlying datasets, Home Office
- Length of study leave is the number of complete years of valid study leave held before switching to the Graduate route.
- The numbers at the top of the bars are the total number of Graduate route entrants in each year.
Figure 3 shows that more than two-thirds (69%) of people taking up the Graduate route in 2023 had been studying for one year or less, compared with 56% in 2022. This is slightly higher than for those extending onto non-Graduate routes, where 60% had studied for one year or less. This broadly aligns with the length of time students are studying in the UK more generally, with 66% of all students who came to the end of their studies in 2023 having studied for one year or less, up from 58% in 2022.
The increase in people switching within one year follows an increase in the number of one-year study visas being issued, which has more than doubled from 137,885 in 2019 to 298,383 in 2023.
For students who started their studies between 2011 and 2018, two-thirds (66%) held no leave to remain in the UK after 3 years. This fell to 61% for 2019, and 44% for the 2020 cohort who are the latest 3-year cohort. The numbers who hold no leave to remain are indicative of the proportion who should have left the UK at the point of analysis.
What do people do once their Graduate route leave ends?
25,469 people’s Graduate visas had expired by the end of 2023, with 63% switching to other routes.
The chart below presents what foreign students who had switched to the Graduate route did before their Graduate leave expired, indicated by their latest visa. However, it is too early to say whether the behaviours of early adopters of the scheme will be indicative of the behaviours of later cohorts.
Figure 4: Graduate visa expiries by subsequent category
Figure 4 shows that 63% of the 25,469 people whose Graduate visas had expired by the end of 2023 had switched to another route. Just under half (46%) had switched to a work route (33% extending into Skilled Worker, 9% into Skilled Worker - Health and Care, and 4% into other work routes). Smaller proportions had returned to study (7%) or switched to family (6%) or other routes (5%).
An additional 17,080 people had extended out of the Graduate route despite still holding valid leave at the end of 2023 with the majority (12,549) switching to work routes (with 8,485 into Skilled Worker, and 3,245 into Skilled Worker - Health and Care).
3. Graduate visa holder earnings
This section presents early findings on the earnings and employment of Graduate visa holders by linking Home Office visa records to HMRC’s Pay As You Earn ( PAYE ) Real Time Information ( RTI ) data.
Data is available from July 2021 (when the Graduate route was introduced) to March 2023. This means insights can be provided over one entire financial year, from April 2022 to March 2023. Findings over the whole period are provided where relevant and stated as such.
All figures and tables in this section relate to the PAYE reported gross earnings of main applicant Graduate visa holders who were aged between 18 and 65, whose visa was granted before the start of the period being looked at (and who had not switched onto any other visa type during this period).
Of the approximately 131,000 unique Graduate visa records extracted from Home Office case working systems that were granted between July 2021 and March 2023, 101,000 (77%) yielded a robust match to HMRC’s Migrant Worker Scan ( MWS ) database, allowing for the linking of these records to HMRC PAYE RTI data. A further 9,000 Graduate visas (7%) were partially matched but have been excluded from all analysis as their match was not deemed robust. Most of those who were not successfully matched to MWS are assumed to have never worked in the UK given that the reason they did not match is likely due to them not applying for a National Insurance number. However, there may be a small number of records that did not match due to differences in the information held by the Home Office and HMRC. These unmatched Graduate visa records are counted as unemployed within all figures reporting on proportions of Graduate visa holders in employment but are excluded from all analyses on earnings. See ‘ About the data ’ section for further details on methodology.
How many Graduate visa holders were in employment?
Of all Graduate visa holders in scope to earn across the whole financial year ending 2023, 73% of Graduate visa holders were in employment at some point during financial year ending 2023; however, of this 73%, the majority (63%) were not in employment for the full year.
Figure 5: Number of months Graduate visa holders worked during financial year ending 2023
Figure 5 shows the number of months in which Graduate visa holders worked during this period varied. This generally leaned towards longer spans, with 61% in employment for at least half of the financial year, and a further 27% consistently working throughout this period. Only 12% worked for less than 6 (but more than 0 months) out of the full 12 months, while 27% did not work at all. While figure 5 includes all Graduate visa holders in scope to earn across financial year ending 2023, this includes those who may have only recently graduated before this period and therefore had less time to seek employment.
How long did it take for Graduate visa holders to start earning?
Of all Graduate visa holders in scope to earn across the whole financial year ending 2023 who were in employment at some point in the financial year, 62% of Graduate visa holders were earning in the first month following their visa being granted.
One in ten (10%) started earning in the second month following their visa being granted and this proportion continues to decrease over subsequent months.
Figure 6: Month in which Graduate visa holders started earning following their visa being granted
- A small number of Graduate visa holders first earned in months that were more than 12 months following the grant of their visa. These proportions each rounded to zero and have therefore not been shown in figure 6.
How did Graduate visa holder employment differ by demographic characteristics?
Of the top 5 nationalities granted Graduate visas, Nigerian nationals were most likely to have worked at least one month (86%).
Chinese nationals were least likely of the top 5 nationalities to have worked at least one month (60%) and for the full year (18%).
Graduate visa holders aged between 25 to 34 and 35 to 49 were more likely to work at least one month compared to those aged 18 to 24 (both 76% compared to 68%). There was no notable difference in the proportion of Graduate visa holders in employment across gender.
Table 2: Proportion of Graduate visa holders in employment during financial year ending 2023 by nationality
- Proportions relate to the proportion of records where nationality was available in the data (excluding ‘all nationalities’ which relates to all available records).
How much did Graduate visa holders earn in each month?
Since the launch of the Graduate route in July 2021, the median monthly pay gradually rose from £1,227 to £1,937 in March 2023.
Figure 7: Median pay in each month from August 2021 to March 2023, where Graduate visa holders earned in the month
- August 2021 represents the first full month of earnings for those granted the Graduate visa in July 2021. This time series looks at each month in isolation and does not track the earnings of the same cohort over time.
While not directly comparable to UK labour market statistics (see ‘ About the data ’ section for further information), this reflects the wider general trend of monthly median earning for the general UK population aged between 18 and 65 across the same period ( Earnings and employment from Pay As You Earn Real Time Information ). Graduate visa holders tended to earn slightly less compared to the general UK population (approximately £300 less as of March 23). However, caution is required when comparing due to variations in cohort composition relative to the general UK population (including factors such as age, region of employment and other characteristics).
How much did Graduate visa holders earn in financial year ending 2023?
The median annual earning for the 73% of Graduate visa holders who were in employment for at least one month in financial year ending 2023 was £17,815. Whereas, for the 27% who were in employment across the entire year, this was £26,460.
Figure 8: Annual Graduate visa holder employment earnings for financial year ending 2023 by earning band
Figure 8 shows that 41% of Graduate visa holders who earned in at least one month in financial year ending 2023 earned less than £15,000. 9% of those who earned for the full year earned less than £15,000. For those in employment across the entire year, just under half (46%) earned between £20,000 and £29,999.
How did annual Graduate visa holder earnings differ by demographic characteristics?
Of the top 5 nationalities granted Graduate visas, USA nationals who worked at least one month had a noticeably higher median annual earning during financial year ending 2023 (£21,135).
In comparison, those from Pakistan had a lower median annual earning (£14,402), as did those from China (£15,762). The differences between nationalities amongst those who were employed over the full year are less stark, although the pattern is broadly similar; median earnings for USA nationals sat above the overall level at £28,000, with earnings for Pakistan nationals sitting below the overall level at £24,955.
Graduate visa holders aged between 35 to 49 who worked at least one month had a higher median annual earning (£19,328) compared to those aged between 18 to 24 and 25 to 34 (£17,701 and £17,746 respectively). Men earned a median of £17,792 (£26,879 where employed over the full year) and women earned £17,856 (£25,988 where employed over the full year).
Table 3: Median annual earnings during financial year ending 2023 by nationality
- Calculations relate to the records where nationality was available in the data set (excluding ‘all nationalities’ which relates to all available records).
What sectors do Graduate visa holders tend to work in?
Graduate visa holders were most likely to be employed within the administrative and support services sector (25%) followed by health and social work and professional, scientific and technical activities (16% and 14% respectively).
Of all Graduate visa holders in scope to earn across the whole financial year ending 2023 who were in employment at some point in the financial year (As categorised using UK Standard Industrial Classification ( SIC ) codes as defined by the Office for National Statistics ( ONS )).
Figure 9: Proportion of Graduate visa holders in employment during financial year ending 2023, by sector
- Sectors are based on the UK SIC codes, as defined by the ONS . These codes have been determined from both the Inter-Departmental Business Register ( IDBR ) and data from Companies House for each PAYE enterprise. Sector information is included where available in the data. ‘Other sectors’ contains remaining sectors with below 175 Graduate visa holders earning at some point in financial year ending 2023. Graduate visa holders may have worked in multiple sectors either concurrently across the financial year or even simultaneously.
Graduate visa holders were least likely to be employed in real estate (1%) and transportation and storage sectors (1%). A smaller proportion of Graduate visa holders worked in the administrative and support services sector for the full financial year (19%) compared to the proportion who were in employment in this sector for at least one month (25%). Compared to the other sectors, this relative proportion was notably larger, suggesting Graduate visa holders are more likely to have worked in this sector for a short period compared to other sectors.
Of the 5 largest sectors of Graduate visa holder employment, Nigerian nationals were the most likely to be working in the health and social work sector. 41% of Nigerian nationals were in employment in this sector for at least one month compared to 14% of Indian nationals, 11% of Pakistani nationals, 10% of USA nationals and 3% of Chinese nationals. Pakistani and Indian nationals were most likely to be employed in the administrative and support service activities sector (38% and 33% respectively). Chinese and USA nationals were most likely to be employed in professional, scientific and technical activities (21% of both).
How much do Graduate visa holders working in different sectors earn?
The sector with the highest annual earning for financial year ending 2023 was finance and insurance. The median earning was £34,846 for those who earned for the entire year and £27,879 for who earned in at least one month.
These annual earnings are substantially above the median annual earning for the full cohort (£26,460).
Figure 10: Median annual Graduate visa holder employment earnings for financial year ending 2023, by sector
- Sectors are based on the UK SIC codes, as defined by the ONS . These codes have been determined from both the IDBR and data from Companies House for each PAYE enterprise. Sector information is included where available in the data. ‘Other sectors’ contains remaining sectors with below 175 Graduate visa holders earning at some point in financial year ending 2023. Graduate visa holders may have worked in multiple sectors either concurrently across the financial year or even simultaneously.
Figure 10 shows that the lowest median annual earning for those Graduate visa holders employed in in at least one month of the financial year ending 2023 was for the accommodation and food service activities sector (£12,805). The administrative and support services sector had the second lowest median annual earning of £14,438 for those who earned in at least one month. The health and social work sector (the second most common sector for Graduate visa holder employment as shown in figure 9) ranked comparatively higher (£16,559). However, those in the administrative and support services sector who worked for the full financial year still earned more than those in the health and social work sector who did the same (£25,550 compared to £24,242).
The gap between the median annual earnings of those working for the full year, compared to those who worked in at least one month, was notably wider for the accommodation and food service activities (£12,805 vs £21,852) and the administrative and support services sectors (£14,438 vs £25,550) compared to the health and social work sector (£16,559 vs £24,242). This suggests that those in these 2 sectors were more likely to be in part-year or seasonal employment compared to those in the health and social work sector.
4. About the data
Migrant journey data
The ‘Migrant journey: user guide’ provides further details on this topic including definitions used, how figures are compiled, data quality and issues arising from figures based on data sourced from an administrative database.
The analysis in this report is based on an earlier data extract than the one which will be used in the upcoming Migrant journey 2023 report. As extracts are taken from a live data-matching system, there may be differences between numbers included in this report and in the upcoming Migrant journey report.
Unless stated otherwise, figures refer to the number of people (main applicants only) whose sponsored study visas and Graduate route extension visas have been successfully matched. Therefore, these figures may not match visa totals published in the Immigration system statistics .
Earnings data
Earnings data used for this report comes from a range of Home Office case working systems. Data is extracted from the Initial Status Analysis ( ISA ) system comprising data from the Case Information Database (CID), the Central Reference System (CRS) and Atlas. This includes data on grant of entry clearance (visas issues) and extensions of stay within the UK.
This data is then matched against HMRC Real Time Information ( RTI ) for Pay As You Earn ( PAYE ) data using the available common identifiers.
Where a visa record had a National Insurance number ( NINo ), this was verified against the Migrant Worker Scan ( MWS ) – a list of individuals who successfully applied for a NINo through the Department for Work and Pensions (DWP) post-16 registration process, or have had a NINo allocated to them as part of their visa granted by the Home Office – before linking to RTI . A record was deemed robust if it matched on at least 5 out of 6 of the following identifiers:
- date of birth
- nationality
Where the visa record had no NINo , fuzzy matching to MWS to assign a NINo was done using the same variables. Two primary matching methods are employed:
- precision matching
- Levenshtein edit distance ( LED )
Precision matching assesses specific variables from visa data against HMRC tax records. LED is used to match visa applicants with minor input errors in key fields, employing substitution, deletion, and replacement to compare strings. Matches where a NINo was not available were deemed robust if the record matched an MWS record on date of birth, surname, forename and at least 2 out of 3 of the following:
The visa records extracted for the purpose of matching to HMRC records in time to produce analyses for this report were extracted in a bespoke way for this data set. This means the number of records extracted may not necessarily reflect total figures presented in other published statistics for the immigration system.
These data and all figures produced from it are classified as ‘Official Statistics in Development’. This means statistics remain subject to further development and will have a wider degree of uncertainty. Please see ‘Official Statistics in Development’ . New methods are being tested to improve quality and provide better coverage across wider visa routes. Limitations of the data is explained in further detail below.
Further information about the data set used for this analysis
The data set contains all unique Graduate visa records that were successfully extracted from Home Office’ case working information databases and securely shared with HMRC.
The data set only contains main applicant Graduate visas granted to those aged 18 and over. It does not contain information on their dependants.
The data set only includes PAYE employment earnings in the UK and does not contain self-employed earnings or earnings from any other sources. This has meant that a small number of Graduate visa holders counted as unemployed might in fact have been self employed, however, the number of self employed Graduate visa holders is very small.
All figures and tables in this section relate to Graduate visa holders whose visa was granted before the start of the period being looked at and who had not switched onto any other visa type during the period.
We currently are unable to identify hours worked or whether employment is part-time or full-time.
By ‘in employment’ for the full financial year, at least some part of it or in a single month, this refers to the monthly employment level gross pay for this period being greater than £0.
Percentages are rounded to the nearest per cent. Figures are rounded to the nearest £. Where percentages are rounded, they may not total 100% because they have been rounded independently.
Age is calculated as of 5 April 2022 for analyses focusing on financial year ending March 2023 and as of the start of the month for analysis focusing on earnings within each month. All other demographic characteristics are recorded as of the time of the Graduate visa application.
A small number of Graduate visa records were missing an expiry date. All held a grant date. Where an expiry date was missing, one was imputed by adding 2 years to the grant date. This will have likely been the correct expiry date for most of these records where an expiry date was missing, however, if the Graduate visa holder was granted the 3-year visa after completing a PhD or had obtained a Graduate visa under a different expiry date, this imputation may be incorrect.
Sectors are based on the UK SIC codes, as defined by the ONS . These codes have been determined from both the IDBR and data from Companies House for each PAYE enterprise. Sector information is included where available in the data. Graduate visa holders may have worked in multiple sectors either concurrently across the financial year or even simultaneously.
Limitations of the data
While figures are derived from HMRC matched data, figures are calculated using a separate methodology to the UK labour market statistics, jointly produced by HMRC and the ONS , and cannot be directly compared to these statistics. Caution is advised when comparing to any other similar data sources of graduate or UK population earning statistics.
As with all administrative data, there will be a small number of cases where data is missing or has been inputted incorrectly. Some information submitted by employers for RTI is late, missing or incorrect.
Data cleaning was performed prior to analysis to allow for optimal matching outcomes. Duplicated visa data was also removed prior to analysis; however, some may remain in the data. We are exploring the use of an alternative data extraction method for visa records to minimise data processing errors and better reflect other published sources.
While standards for a ‘robust’ match to HMRC data have been set high, matches may still not be 100% accurate. Individuals with near identical personal details may be incorrectly identified as the same person.
Unmatched Graduate visa records are counted as unemployed within all figures reporting on proportions of Graduate visa holders in employment. Some Graduate visa holders may have been in employment but were not successfully matched due to discrepancies in the personal identifier information held in either Home Office or HMRC data used for matching. There may be instances where the likelihood of being matched differs by certain demographics or other characteristics
Is this page useful?
- Yes this page is useful
- No this page is not useful
Help us improve GOV.UK
Don’t include personal or financial information like your National Insurance number or credit card details.
To help us improve GOV.UK, we’d like to know more about your visit today. Please fill in this survey (opens in a new tab) .

IMAGES
VIDEO
COMMENTS
Score 94% Score 94%. 4.4 Writing the Data Analysis Chapter (s): Results and Evidence. Unlike the introduction, literature review and methodology chapter (s), your results chapter (s) will need to be written for the first time as you draft your thesis even if you submitted a proposal, though this part of your thesis will certainly build upon the ...
Interpreting the data, using illustrative examples Conclusion Concise summary of the main findings Task 4.2: Introduction to a data chapter In this session we will be discussing a sample data chapter extract (in Appendix A on pages 42-53). It comes from a study of ways in which international students and a British teacher
The results chapter (also referred to as the findings or analysis chapter) is one of the most important chapters of your dissertation or thesis because it shows the reader what you've found in terms of the quantitative data you've collected. It presents the data using a clear text narrative, supported by tables, graphs and charts.
Writing up your PhD (Qualitative Research) Independent Study version ... About the course i-iii . 1 Structure and Introduction 1-13 . 2 The Literature Review 14-28 . 3 The Methodology Chapter 29-37 . 4 The Data Chapters 38-54 . 5 The Final Chapter 55-73 ... the sense of argument develops through the whole process of data collection, analysis and
Step 4: Acknowledge the limitations of your study. The fourth step in writing up your discussion chapter is to acknowledge the limitations of the study. These limitations can cover any part of your study, from the scope or theoretical basis to the analysis method (s) or sample.
The Method chapter typically contains the following three subsections: Subjects or Participants, Instrumentation or Measures, and Procedures. In addition, the Method chapter of a dissertation proposal often contains a Statistical Analysis or Data Analysis section, in which procedures for approaching the data are outlined.
The results chapter in a dissertation or thesis (or any formal academic research piece) is where you objectively and neutrally present the findings of your qualitative analysis (or analyses if you used multiple qualitative analysis methods ). This chapter can sometimes be combined with the discussion chapter (where you interpret the data and ...
An understanding of the data analysis that you will carry out on your data can also be an expected component of the Research Strategy chapter of your dissertation write-up (i.e., usually Chapter Three: Research Strategy). Therefore, it is a good time to think about the data analysis process if you plan to start writing up this chapter at this ...
Abstract. Descriptive and analytic studies will need to be performed as part of most PhD theses. The initial step is to identify whether the data you have gathered follows a normal or a skewed distribution pattern. In normal distribution data, parametric tests need to be used (e.g. mean and student t -test), while in skewed data, non-parametric ...
The data analysis chapter is a crucial section of a research dissertation that involves the examination, interpretation, and synthesis of collected data. ... Be it master thesis data analysis, an undergraduate one or for PhD scholars, the steps remain almost the same as we have discussed in this guide. The primary focus is to be connected with ...
analysis. At the end of this chapter, some new trends and developments in the field will be outlined. Here, new types of data, a trend ... (or PhD dissertation) but treated as illustrations. Another way of describing (and doing) qualitative data anal- ... Qualitative data analysis is the classification and interpretation of linguistic (or ...
The process of data analysis is a detailed one. It starts from managing the data in the right format to final interpretation of it for arriving at the conclusions. Data must be cleaned, i.e ...
And place questionnaires, copies of focus groups and interviews, and data sheets in the appendix. On the other hand, one must put the statistical analysis and sayings quoted by interviewees within the dissertation. 8. Thoroughness of Data. It is a common misconception that the data presented is self-explanatory.
3.6 Organisation of data 3.6.1 Transcription 3.6.2 Categories of interaction Task 3.4 The first student's Research Methods chapter was 34 pages long; the second student's Research Methodology and Method chapter was 47 pages. Compare their coverage with what you have drafted, or plan to include, in your Methodology chapter.
WRITING CHAPTER 4: DATA ANALYSIS AND FINDINGSWriting chapter 4 (Data Analysis and Findings) of a PhD/DBA Dissertation can be tideous and erroneous. Especiall...
if you write a scientific dissertation, or anyway using quantitative methods, you will have some objective results that you will present in the Results chapter. You will then interpret the results in the Discussion chapter. B) More common for qualitative methods. - Analysis chapter. This can have more descriptive/thematic subheadings.
The method you choose will depend on your research objectives and questions. These are the most common qualitative data analysis methods to help you complete your dissertation: 2. Content analysis: This method is used to analyze documented information from texts, email, media and tangible items.
Fast-Track Your Data Analysis, Today. Enter your details below, pop us an email, or book an introductory consultation. If you are a human seeing this field, please leave it empty. Get 1-on-1 help analysing and interpreting your qualitative or quantitative dissertation or thesis data from the experts at Grad Coach. Book online now.
5. Analysis and synthesis 6. Conclusions and recommendations Chapter 1: Introduction This chapter makes a case for the signifi-cance of the problem, contextualizes the study, and provides an introduction to its basic components. It should be informative and able to stand alone as a document. • Introduction: The introduction includes an
CHAPTER 6: DATA ANALYSIS AND INTERPRETATION 357 The results of qualitative data analysis guide subsequent data collection, and analysis is thus a less-distinct final stage of the research process than quantitative analysis, where data analysis does not begin until all data have been collected and condensed into numbers;
CHAPTER FOUR. DATA ANALYSIS AND PRESENTATION OF RES EARCH FINDINGS 4.1 Introduction. The chapter contains presentation, analysis and dis cussion of the data collected by the researcher. during the ...
PhD Statistical Analysis Help. Statistical data analysis is at the heart of your research, as all your findings are based on the way you analyze your raw data. Thus, it can be costly to make mistakes at this stage of research. If you do not use the right statistical tests, tools and techniques, it can be disastrous for your entire research.
4.1 INTRODUCTION. In this chapter, I describe the qualitative analysis of the data, including the practical steps involved in the analysis. A quantitative analysis of the data follows in Chapter 5. In the qualitative phase, I analyzed the data into generative themes, which will be described individually. I describe how the themes overlap.
Previous seminars in our series have attended to divisions, but also possibilities, engendered by data along various fault lines and contexts (from 19th-century statistical thinking to biases in archives, from the challenges of quantification to the history of data governance). With this seminar on 'Ancient Data' we focus on what happens to ancient sources—textual, material and visual ...
In this individual patient data meta-analysis of 4 RCTs including 1316 patients and 1668 saphenous vein grafts, ticagrelor DAPT was associated with a significantly lower risk of saphenous vein graft failure and a significantly higher risk of clinically important bleeding events than was aspirin. ... Corresponding Author: Mario Gaudino, MD, PhD ...
As the nation prepares to mark the 70th anniversary of the landmark U.S. Supreme Court ruling in Brown v. Board of Education, a new report from researchers at Stanford and USC shows that racial and economic segregation among schools has grown steadily in large school districts over the past three decades — an increase that appears to be driven in part by policies favoring
Further information about the data set used for this analysis. The data set contains all unique Graduate visa records that were successfully extracted from Home Office' case working information ...