Body mass index, waist circumference, and mortality in subjects older than 80 years: a Mendelian randomization study
Affiliations.
- 1 China CDC Key Laboratory of Environment and Population Health, National Institute of Environmental Health, Chinese Center for Disease Control and Prevention, #7 Panjiayuan Nanli, Chaoyang, Beijing 100021, China.
- 2 Department of Bioinformatics and Biostatistics, School of Life Sciences and Biotechnology, Shanghai Jiao Tong University, Shanghai, China.
- 3 Department of Epidemiology and Biostatistics, School of Public Health, Jilin University, Changchun, China.
- 4 Department of Nutrition and Food Hygiene, School of Public Health, Institute of Nutrition, Fudan University, Shanghai, China.
- 5 Department of Big Data in Health Science, School of Public Health, Zhejiang University, Hangzhou, China.
- 6 Center for Global Health, School of Public Health, Nanjing Medical University, Nanjing, China.
- 7 Department of Epidemiology, School of Public Health, Southern Medical University, Guangzhou, China.
- 8 School of Population Medicine and Public Health, Chinese Academy of Medical Sciences & Peking Union Medical College, Beijing, China.
- 9 Department of Occupational Health and Environment Health, School of Public Health, Anhui Medical University, Hefei, China.
- 10 Division of Non-Communicable Disease and Healthy Aging Management, Chinese Center for Disease Control and Prevention, Beijing, China.
- 11 Department of Medicine, Duke Molecular Physiology Institute and Division of Rheumatology, Duke University School of Medicine, Durham, NC, USA.
- 12 Center for Study of Healthy Aging and Development Studies, Peking University, Beijing, China.
- 13 School of Public Health and Social Work, Queensland University of Technology, Brisbane, Australia.
- PMID: 38626306
- DOI: 10.1093/eurheartj/ehae206
Background and aims: Emerging evidence has raised an obesity paradox in observational studies of body mass index (BMI) and health among the oldest-old (aged ≥80 years), as an inverse relationship of BMI with mortality was reported. This study was to investigate the causal associations of BMI, waist circumference (WC), or both with mortality in the oldest-old people in China.
Methods: A total of 5306 community-based oldest-old (mean age 90.6 years) were enrolled in the Chinese Longitudinal Healthy Longevity Survey (CLHLS) between 1998 and 2018. Genetic risk scores were constructed from 58 single-nucleotide polymorphisms (SNPs) associated with BMI and 49 SNPs associated with WC to subsequently derive causal estimates for Mendelian randomization (MR) models. One-sample linear MR along with non-linear MR analyses were performed to explore the associations of genetically predicted BMI, WC, and their joint effect with all-cause mortality, cardiovascular disease (CVD) mortality, and non-CVD mortality.
Results: During 24 337 person-years of follow-up, 3766 deaths were documented. In observational analyses, higher BMI and WC were both associated with decreased mortality risk [hazard ratio (HR) 0.963, 95% confidence interval (CI) 0.955-0.971 for a 1-kg/m2 increment of BMI and HR 0.971 (95% CI 0.950-0.993) for each 5 cm increase of WC]. Linear MR models indicated that each 1 kg/m2 increase in genetically predicted BMI was monotonically associated with a 4.5% decrease in all-cause mortality risk [HR 0.955 (95% CI 0.928-0.983)]. Non-linear curves showed the lowest mortality risk at the BMI of around 28.0 kg/m2, suggesting that optimal BMI for the oldest-old may be around overweight or mild obesity. Positive monotonic causal associations were observed between WC and all-cause mortality [HR 1.108 (95% CI 1.036-1.185) per 5 cm increase], CVD mortality [HR 1.193 (95% CI 1.064-1.337)], and non-CVD mortality [HR 1.110 (95% CI 1.016-1.212)]. The joint effect analyses indicated that the lowest risk was observed among those with higher BMI and lower WC.
Conclusions: Among the oldest-old, opposite causal associations of BMI and WC with mortality were observed, and a body figure with higher BMI and lower WC could substantially decrease the mortality risk. Guidelines for the weight management should be cautiously designed and implemented among the oldest-old people, considering distinct roles of BMI and WC.
Keywords: Body mass index; Causal inference; Mendelian randomization; Mortality; Waist circumference.
© The Author(s) 2024. Published by Oxford University Press on behalf of the European Society of Cardiology. All rights reserved. For commercial re-use, please contact [email protected] for reprints and translation rights for reprints. All other permissions can be obtained through our RightsLink service via the Permissions link on the article page on our site—for further information please contact [email protected].

Grants and funding
- P30-AG028716/NH/NIH HHS/United States
- Search Menu
- Sign in through your institution
- Advance Articles
- Editor's Choice
- Braunwald's Corner
- ESC Guidelines
- EHJ Dialogues
- Issue @ a Glance Podcasts
- CardioPulse
- Weekly Journal Scan
- European Heart Journal Supplements
- Year in Cardiovascular Medicine
- Asia in EHJ
- Most Cited Articles
- ESC Content Collections
- Author Guidelines
- Submission Site
- Why publish with EHJ?
- Open Access Options
- Submit from medRxiv or bioRxiv
- Author Resources
- Self-Archiving Policy
- Read & Publish
- Advertising and Corporate Services
- Advertising
- Reprints and ePrints
- Sponsored Supplements
- Journals Career Network
- About European Heart Journal
- Editorial Board
- About the European Society of Cardiology
- ESC Publications
- War in Ukraine
- ESC Membership
- ESC Journals App
- Developing Countries Initiative
- Dispatch Dates
- Terms and Conditions
- Journals on Oxford Academic
- Books on Oxford Academic
Article Contents
- Introduction
- Conclusions
- Supplementary data
- Declarations
- Data Availability
- Ethical Approval
- Pre-registered Clinical Trial Number
Body mass index, waist circumference, and mortality in subjects older than 80 years: a Mendelian randomization study

Yuebin Lv, Yue Zhang and Xinwei Li contributed equally to the study.
- Article contents
- Figures & tables
- Supplementary Data
Yuebin Lv, Yue Zhang, Xinwei Li, Xiang Gao, Yongyong Ren, Luojia Deng, Lanjing Xu, Jinhui Zhou, Bing Wu, Yuan Wei, Xingyao Cui, Zinan Xu, Yanbo Guo, Yidan Qiu, Lihong Ye, Chen Chen, Jun Wang, Chenfeng Li, Yufei Luo, Zhaoxue Yin, Chen Mao, Qiong Yu, Hui Lu, Virginia Byers Kraus, Yi Zeng, Shilu Tong, Xiaoming Shi, Body mass index, waist circumference, and mortality in subjects older than 80 years: a Mendelian randomization study, European Heart Journal , 2024;, ehae206, https://doi.org/10.1093/eurheartj/ehae206
- Permissions Icon Permissions
Emerging evidence has raised an obesity paradox in observational studies of body mass index (BMI) and health among the oldest-old (aged ≥80 years), as an inverse relationship of BMI with mortality was reported. This study was to investigate the causal associations of BMI, waist circumference (WC), or both with mortality in the oldest-old people in China.
A total of 5306 community-based oldest-old (mean age 90.6 years) were enrolled in the Chinese Longitudinal Healthy Longevity Survey (CLHLS) between 1998 and 2018. Genetic risk scores were constructed from 58 single-nucleotide polymorphisms (SNPs) associated with BMI and 49 SNPs associated with WC to subsequently derive causal estimates for Mendelian randomization (MR) models. One-sample linear MR along with non-linear MR analyses were performed to explore the associations of genetically predicted BMI, WC, and their joint effect with all-cause mortality, cardiovascular disease (CVD) mortality, and non-CVD mortality.
During 24 337 person-years of follow-up, 3766 deaths were documented. In observational analyses, higher BMI and WC were both associated with decreased mortality risk [hazard ratio (HR) 0.963, 95% confidence interval (CI) 0.955–0.971 for a 1-kg/m 2 increment of BMI and HR 0.971 (95% CI 0.950–0.993) for each 5 cm increase of WC]. Linear MR models indicated that each 1 kg/m 2 increase in genetically predicted BMI was monotonically associated with a 4.5% decrease in all-cause mortality risk [HR 0.955 (95% CI 0.928–0.983)]. Non-linear curves showed the lowest mortality risk at the BMI of around 28.0 kg/m 2 , suggesting that optimal BMI for the oldest-old may be around overweight or mild obesity. Positive monotonic causal associations were observed between WC and all-cause mortality [HR 1.108 (95% CI 1.036–1.185) per 5 cm increase], CVD mortality [HR 1.193 (95% CI 1.064–1.337)], and non-CVD mortality [HR 1.110 (95% CI 1.016–1.212)]. The joint effect analyses indicated that the lowest risk was observed among those with higher BMI and lower WC.
Among the oldest-old, opposite causal associations of BMI and WC with mortality were observed, and a body figure with higher BMI and lower WC could substantially decrease the mortality risk. Guidelines for the weight management should be cautiously designed and implemented among the oldest-old people, considering distinct roles of BMI and WC.

Opposite causal associations of body mass index (BMI) and waist circumference (WC) with mortality among Chinese oldest-old based on 1998–2018 Chinese Longitudinal Healthy Longevity Survey (CLHLS). Upper: a scheme for the study design. Left lower: non-linear Mendelian randomization results for the causal association of BMI and WC with mortality. Right lower: joint effect analyses of BMI and WC on mortality. CVD, cardiovascular disease.
- body mass index procedure
- cardiovascular diseases
- waist circumference
- mendelian randomization analysis
Email alerts
More on this topic, related articles in pubmed, citing articles via, looking for your next opportunity, affiliations.
- Online ISSN 1522-9645
- Print ISSN 0195-668X
- Copyright © 2024 European Society of Cardiology
- About Oxford Academic
- Publish journals with us
- University press partners
- What we publish
- New features
- Open access
- Institutional account management
- Rights and permissions
- Get help with access
- Accessibility
- Media enquiries
- Oxford University Press
- Oxford Languages
- University of Oxford
Oxford University Press is a department of the University of Oxford. It furthers the University's objective of excellence in research, scholarship, and education by publishing worldwide
- Copyright © 2024 Oxford University Press
- Cookie settings
- Cookie policy
- Privacy policy
- Legal notice
This Feature Is Available To Subscribers Only
Sign In or Create an Account
This PDF is available to Subscribers Only
For full access to this pdf, sign in to an existing account, or purchase an annual subscription.
- Open access
- Published: 10 May 2024
Lipids as potential mediators linking body mass index to diabetes: evidence from a mediation analysis based on the NAGALA cohort
- Song Lu 1 na1 ,
- Qun Wang 2 na1 ,
- Hengcheng Lu 2 na1 ,
- Maobin Kuang 1 , 2 ,
- Min Zhang 1 , 3 ,
- Guotai Sheng 2 ,
- Yang Zou 2 &
- Xiaoping Peng 1 , 3
BMC Endocrine Disorders volume 24 , Article number: 66 ( 2024 ) Cite this article
202 Accesses
Metrics details
Body mass index (BMI) and lipid disorders are both known to be strongly associated with the development of diabetes, however, the indirect effect of lipid parameters in the BMI-related diabetes risk is currently unknown. This study aimed to investigate the mediating role of lipid parameters in the association of BMI with diabetes risk.
We assessed the association of diabetes risk with BMI, as well as lipid parameters including high-density lipoprotein cholesterol(HDL-C), low-density lipoprotein cholesterol(LDL-C F and LDL-C S ), triglycerides(TG), total cholesterol(TC), remnant cholesterol(RC), non-HDL-C, and combined indices of lipid parameters with HDL-C (RC/HDL-C ratio, TG/HDL-C ratio, TC/HDL-C ratio, non-HDL/HDL-C ratio, LDL/HDL-C ratio) using data from 15,453 subjects in the NAGALA project. Mediation models were used to explore the mediating role of lipid parameters in the association of BMI with diabetes risk, and mediation percentages were calculated for quantifying the strength of the indirect effects. Finally, receiver operating characteristic curve (ROC) analysis was used to compare the accuracy of BMI and BMI combined with lipid parameters in predicting incident diabetes.
Multivariate regression models, adjusted for confounding factors, demonstrated robust associations of lipid parameters, BMI, with diabetes risk, with the exception of TC, LDL-C F , LDL-C S , and non-HDL-C. Mediation analysis showed that lipid parameters except TC, LDL-C F , LDL-C S , and Non-HDL-C were involved in and mediated the association of BMI with diabetes risk, with the largest mediation percentage being the RC/HDL-C ratio, which was as high as 40%; it is worth mentioning that HDL-C and HDL-C-related lipid ratio parameters also play an important mediating role in the association between BMI and diabetes, with the mediator proportion being greater than 30%. Finally, based on the ROC results, we found that the prediction performance of all lipid parameters in the current study except TC was significantly improved when combined with BMI.
Our fresh findings suggested that lipid parameters partially mediated the association of BMI with diabetes risk; this result indicated that in the context of diabetes risk screening and disease management, it is important to not only monitor BMI but also pay attention to lipid parameters, particularly HDL-C and HDL-C-related lipid ratio parameters.
Peer Review reports
Diabetes is a chronic metabolic disorder characterized by disturbances in blood glucose metabolism, leading to systemic involvement of multiple organs and systems [ 1 ]. The latest research forecasting models reported that the global prevalence of diabetes is 529 million in 2021, and driven by the obesity epidemic [ 2 ], a staggering 1.31 billion people are projected to live with diabetes globally by 2050 [ 3 ]. In addition, the incidence of diabetes is gradually showing a trend towards younger age [ 4 ], which poses a new and great challenge to global public health. Therefore, early identification of diabetes risk and early intervention based on risk factors is essential to reduce the incidence of diabetes as well as to slow down the disease progression.
It is well known that obesity is closely associated with the development of diabetes and is a key driver of the diabetes epidemic [ 2 , 5 , 6 ]. BMI is the most classic and simple measure of obesity [ 7 ] and an important obesity index for measuring the risk of developing diabetes [ 8 , 9 , 10 , 11 , 12 , 13 ]. To date, there is still no clear-cut mechanism linking BMI directly to the development of diabetes, and the main arguments around obesity leading to diabetes currently focus on insulin resistance (IR), impaired β-cell function, and metabolic damage from chronic inflammation [ 14 , 15 , 16 ]. Recently, an increasing number of studies have focused on the impact of obesity-related disorders of lipid metabolism on the pathogenesis of diabetes, and researchers have used metabolomics to identify a range of lipid markers of obesity-related diabetic risk, mainly including phospholipids and sphingolipids [ 17 , 18 , 19 , 20 , 21 ]. These findings suggest that lipids may play an important role in obesity-related diabetes risk. Considering the important value of BMI in risk assessment of diabetes and the potential impact of lipid metabolism on diabetes, it is important to further clarify the impact of lipid parameters on the association of BMI with diabetes risk in real-world studies, which could provide an important basis for clarifying the pathogenesis and the daily risk management of diabetes. To clarify the answer to this question, in the current study we used mediation analysis on data from 15,453 subjects of the NAGALA cohort to investigate lipid parameters that potentially mediate the link of BMI with diabetes risk, quantifying their contributions and identifying the most impactful lipids for diabetes risk management.
Data source and study population
To elucidate the role of lipids in BMI-related diabetes risk, we extracted dataset from 20,944 participants in the NAGALA (1994–2016) project. The dataset was collected by Okamura’s team and stored in the Dryad public database (https://datadryad.org/stash/dataset/doi: https://doi.org/10.5061/dryad.8q0p192 ). Based on Dryad’s Data Sharing Terms of Service, researchers can use publicly available data from the database to conduct in-depth analyses to explore new discoveries that will benefit academic progress. Detailed information about the NAGALA cohort study can be found in the previously published research [ 22 ]. According to the new research objectives, we further excluded the subjects with diabetes, impaired fasting glucose, liver disease, excessive drinking, incomplete data, using medicine at baseline, and withdraw survey with unknown reason, and finally, 15,453 subjects were included in the current study (Fig. 1 ). The NAGALA project has been authorized by the Ethics Committee of Murakami Memorial Hospital, and obtained informed consent from all subjects for their data usage [ 22 ]. This study was a secondary analysis of the NAGALA cohort study, which has been approved by the Ethics Committee of Jiangxi Province People’s Hospital. Furthermore, since the publicly available dataset has been anonymized, the Ethics Committee of Jiangxi Provincial People’s Hospital waived the need for informed consent from the subjects.
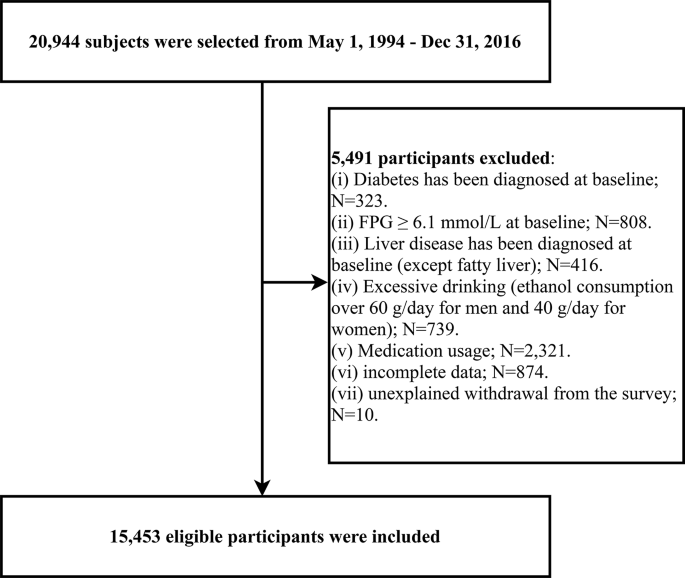
Flow chart of study participants
Data collection, measurement, and calculation
All variables in the current study were contained in the NAGALA dataset [ 22 ], which were measured by the medical personnel using standardized methods and recorded in standardized questionnaires, including systolic/diastolic blood pressure (S/DBP), age, sex, weight, height, waist circumference (WC), TC, fasting plasma glucose (FPG), alanine aminotransferase (ALT), HDL-C, TG, gamma-glutamyl transferase (GGT), glycated hemoglobin (HbA1c), aspartate aminotransferase (AST), drinking status, smoking status, habit of exercise, and fatty liver. Among them, the blood biochemical indicators were measured and recorded by the automatic biochemical analyzer after all subjects fasting at least 8 h. Lifestyle factors were defined as following: (1) Drinking status was grouped according to the alcohol consumption in the past month, including non or little (< 40 g/w), light (40–139 g/w), moderate (140–279 g/w), and heavy (> 280 g/w) [ 23 ]. (2) Smoking status was grouped as non-smoking, former smoking, and current smoking. (3) Having exercise habits required engaging physical activity at least once a week. Moreover, fatty liver was diagnosed by gastroenterology experts based on abdominal ultrasound examination results of the subjects [ 24 ]. Based on baseline parameter information, we further calculated BMI and multiple lipid parameters, with the detailed calculation process presented in Fig. 2 [ 25 , 26 , 27 , 28 , 29 , 30 , 31 , 32 ]. The main results of the study on LDL-C are based on calculations using the Modified Friedewald Formula. To ensure the robustness of the results, we also used the Sampson formula to calculate LDL-C concentrations [ 26 ] (Fig. 2 ). In addition, to differentiate between the two methods of calculation, we referred to LDL-C results from the Modified Friedewald formula as LDL-C F and those from the Sampson formula as LDL-C S .
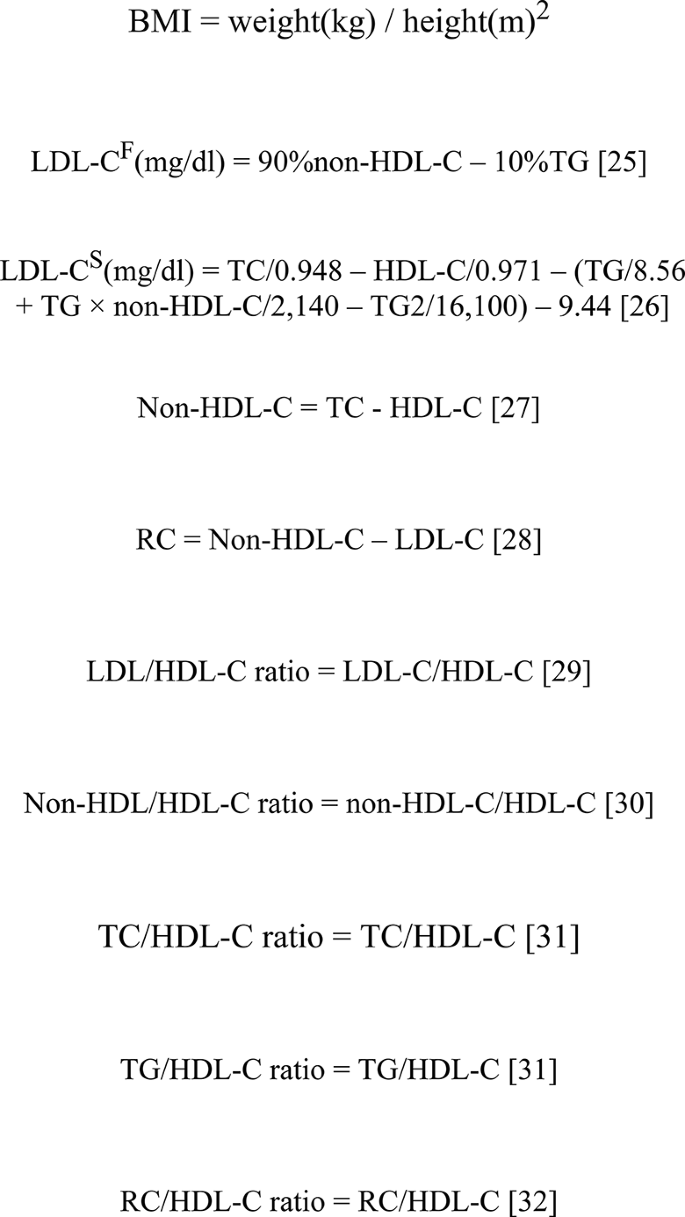
Formulas for calculating BMI and lipid parameters
Diagnosis of diabetes
In the present study, the diagnosis of diabetes followed the criteria set by the American Diabetes Association [ 33 ], as follows: HbA1c ≥ 6.5% or FPG ≥ 7.0 mmol/L, along with self-reported diabetes.
Statistical analysis
Subjects were grouped based on whether incident diabetes was diagnosed during follow-up [ 22 ]. We employed Marginal Structural Models to compute and quantify the magnitude of differences in baseline characteristics between diabetes and non-diabetes groups (prior to quantifying the differences, we performed BOX-COX transformations for skewed-distributed data), and differences greater than 10% were considered statistically significant [ 34 , 35 ].
Prior to validating the mediation effect of lipid parameters in the association of BMI with diabetes risk, we computed the variance inflation factor for lipid parameters, BMI, and each covariate using linear regression equations, and covariates with variance inflation factor > 5 were considered collinear variables [ 36 ]. Based on the results of collinearity screening, we observed collinearity between all lipid parameters and weight and DBP, as well as between BMI and weight, DBP, and WC (Supplementary Tables 1–12); consequently, these variables (weight, DBP, and WC) will be excluded in subsequent multivariable regression models and mediation analyses.
We followed the approach recommended by Professor VanderWeele for conducting the mediation analysis [ 37 , 38 ]. First, we employed a series of multivariable Cox regression models to examine the associations of lipid parameters/BMI with diabetes risk. In these models, we progressively adjusted for important demographic characteristics (height, age, sex), lifestyle factors (habit of exercise, drinking and smoking status), fatty liver, and metabolic factors related to blood pressure, blood glucose, and liver enzymes (SBP, GGT, FPG, ALT, HbA1c, AST) [ 1 , 39 , 40 ]. The strategy and process of progressive adjustment were conducted following the Strengthening the Reporting of Observational Studies in Epidemiology, and these steps were presented in the main analysis. Furthermore, when validating the association of BMI with diabetes risk, we conducted additional adjustments for the mediating variables (11 lipid parameters) and observed whether the association of BMI with diabetes weakened, to assess if the prerequisites for conducting a mediation analysis were met [ 37 , 38 ]. Subsequently, we employed multiple linear regression to examine the association of BMI with lipid parameters, following the adjustment strategy outlined earlier [ 1 , 41 , 42 ]. Finally, we constructed mediation models to determine whether lipid parameters mediated the association of BMI with diabetes risk, and quantified the mediation effects of lipid parameters by calculating the mediation percentage, which is the ratio of the indirect effect to the total effect. To assess the significance of the mediation effects, we employed the Bootstrap sampling method with 1000 iterations. It is worth noting that the triglycerides glucose (TyG) index is calculated from parameters such as blood lipids and blood glucose. Considering that a large number of recent researches have shown that the TyG index is strongly associated with BMI and diabetes, in the current study we also examined the mediating role of the TyG index in the risk of BMI-related diabetes. Finally, ROC analysis was used to calculate the accuracy of BMI and BMI combined with lipid parameters in predicting incident diabetes, and the DeLong test was used to compare the area under the curve (AUC) among the models. All analyses were conducted using R version 3.4.3 and Empower(R) version 4.1. Two-tailed tests were employed, and statistical significance was set at P < 0.05.
Characteristics of study subjects
A total of 15,453 subjects with a mean age of 43.7 ± 8.9 years were included in this study, and a total of 372 were diagnosed with new-onset diabetes. Table 1 shows the differences in the baseline characteristics of the study subjects grouped according to whether or not they were diagnosed with diabetes. We observed significant differences (standardized difference > 10%) in all baseline variables between the diabetes and non-diabetes groups. It is worth mentioning that the most substantial disparity between the two groups lay in the baseline glucose-related measures (FPG and HbA1c), with a standardized difference exceeding 100%. Furthermore, we observed that the BMI of diabetic participants was significantly higher than that of non-diabetic participants, with a standardized difference between the two groups reaching 86%. Lastly, it’s important to mention that in terms of lipid parameters, the standardized difference for the RC/HDL-C ratio was the largest (88%).
Relationship of BMI with diabetes
After thorough adjustment for potential confounders, the current study also confirmed a significant positive correlation of BMI with the risk of diabetes (Table 2 ). In models 1 to 3, we progressively adjusted for all covariates except lipid parameters (mediating variables), and the hazard ratio (HR) for BMI-related diabetes risk was 1.096 in Model 3. Furthermore, we also included the lipid parameters (mediating variables) individually as covariates in the model (Table 2 , models 4–14); the results revealed that except for the models where TC (Model 4: HR = 1.097) and LDL-C F (Model 7: HR = 1.096) were incorporated as covariates for adjustment, other models indicated a weakening of the association of BMI with diabetes risk. These findings suggested that, apart from TC and LDL-C F , other lipid parameters might potentially mediate the association of BMI with diabetes risk.
Relationship of lipid parameters with incident diabetes
We proceeded to run three multivariate Cox regression models to validate the associations of lipid parameters with diabetes risk (Table 3 ). It can be observed that in Model I, all lipid parameters were associated with diabetes risk; among them, apart from HDL-C which exhibited a negative correlation with diabetes, the rest of the lipid parameters showed a positive correlation with diabetes. However, in Models II and III, with further adjustments for demographic characteristics, lifestyle factors, fatty liver, and blood pressure and glucose enzyme metabolism factors, we observed that the associations between TC, LDL-C F , LDL-C S , and non-HDL-C with diabetes risk disappeared, which suggested that TC, LDL-C F , LDL-C S , and non-HDL-C may not be the mediating factors in the association of BMI with diabetes risk. Additionally, it’s important to note that among all lipid parameters, RC and lipid ratios such as RC/HDL-C exhibited the highest level of association with diabetes risk (RC: HR = 2.23; RC/HDL-C ratio: HR = 2.67).
Relationship of BMI with lipid parameters
Table 4 presents the results of the correlation analysis of BMI with lipid parameters. In linear regression, after thorough adjustment for confounding factors, we found significant associations between all lipid parameters and BMI, and except for HDL-C which exhibited a negative correlation with BMI (β=-2.47), the rest of the lipid parameters showed significant positive correlations with BMI. Additionally, it’s worth mentioning that the association of TC/HDL-C ratio with BMI was the strongest (β = 4.19).
Mediating effect of lipid parameters on the association of BMI with incident diabetes
Based on the results of the correlation analysis mentioned above, we proceeded to conduct further mediation analysis. Table 5 ; Fig. 3 present the results of the mediation analysis of lipid parameters in the association of BMI with the risk of diabetes incidence. The results revealed that, excluding TC, LDL-C F , LDL-C S , non-HDL-C, and TyG index the remaining 8 lipid parameters mediated the association of BMI with diabetes, with RC/HDL-C ratio having the most significant impact and a mediation percentage of 40%. Furthermore, it’s worth noting that the non-HDL/HDL-C ratio, TC/HDL-C ratio, LDL/HDL-C ratio, and HDL-C also exhibited mediation percentages exceeding 30%, establishing them as robust mediators in the association of BMI with diabetes risk; interestingly, unconventional lipid parameters tended to display higher mediation percentages compared to conventional ones.
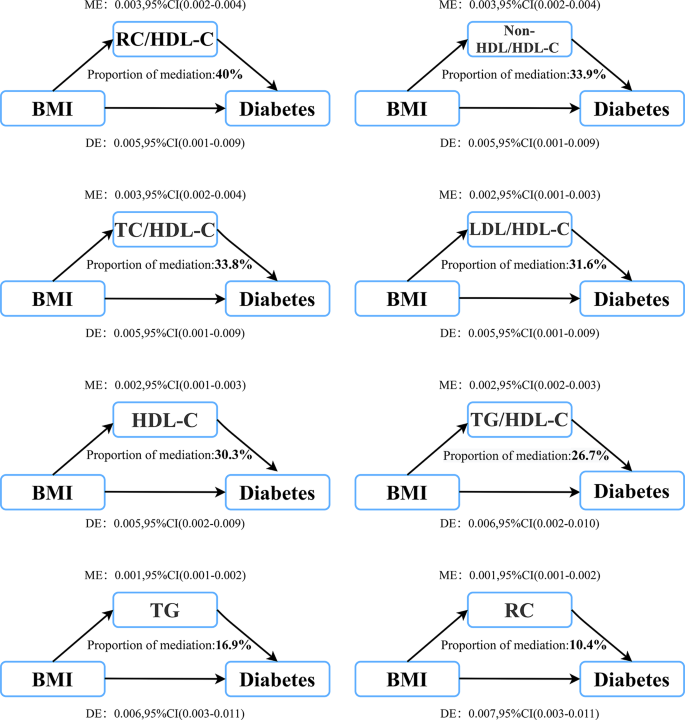
Lipid parameters mediation models of the relationship between BMI and incident diabetes. ME: Mediation effect; DE: Direct effect; BMI: Body mass index
Area under the ROC curve, specificity, sensitivity, PPV, and NPV of BMI and BMI combined lipid parameters to predict incident diabetes
The results in Table 6 demonstrate that when combined with BMI, all lipid parameters resulted in higher AUC values compared to BMI alone. The combination of RC/HDL-C ratio and BMI had the highest AUC value of 0.7748. Moreover, the accuracy of identifying incident diabetes was significantly enhanced by incorporating BMI with all lipid parameters, except for TC + BMI (All DeLong test p < 0.05).
In this study encompassing 15,453 participants, we identified that apart from TC, LDL-C, and non-HDL-C, lipid parameters including RC, HDL-C, TG, TG/HDL-C ratio, TC/HDL-C ratio, LDL/HDL-C ratio, non-HDL/HDL-C ratio and RC/HDL-C ratio played significant mediating roles in the association of BMI with diabetes risk. Among them, the RC/HDL-C ratio made the most substantial contribution to the association of BMI with diabetes risk, accounting for a substantial 40%. Furthermore, the non-HDL/HDL-C ratio, LDL/HDL-C ratio, TC/HDL-C ratio and HDL-C exhibited significant mediation percentages as well, at 33.9%, 31.6%, 33.8% and 30.3%, respectively.
With the advancement of economies and shifts in lifestyle habits, the prevalence of diabetes is sweeping across the globe at an alarming rate, with a notable trend towards affecting younger individuals with lower body weight [ 1 , 2 ]. Abundant epidemiological evidence underscores that both BMI and lipid dysregulation are pivotal risk factors in the onset and progression of diabetes [ 5 , 43 , 44 ]. BMI, serving as a body measurement indicator reflecting overall obesity, is associated with an elevated risk of diabetes and demonstrates a dose-response relationship; in comparison to other obesity indices, BMI might be the optimal predictor of diabetes risk [ 8 , 11 , 45 , 46 ]. The investigation of lipid parameters in relation to the risk of diabetes has emerged as a recent research focus, particularly concerning composite lipid parameters [ 27 , 30 , 31 , 47 , 48 ]. In the present analysis, we assessed the associations between 11 lipid parameters and diabetes, and the findings revealed that a majority of lipid parameters were closely linked to the risk of diabetes, with the RC/HDL-C ratio displaying the strongest association with diabetes incidence risk. Building upon this, our subsequent mediation analysis revealed that the RC/HDL-C ratio serves as the most robust mediating factor in the association of BMI with diabetes risk. It is worth noting that the current study did not find significant associations between TC, LDL-C, and non-HDL-C with diabetes risk, which aligned with some previous reports [ 48 , 49 , 50 , 51 ]. However, it should be mentioned that certain studies have also indicated that TC, LDL-C, and non-HDL-C can indeed reflect diabetes risk [ 52 , 53 ]. Further research is required to elucidate the discrepancies in these study outcomes. In the context of this study, the lack of significant mediation effects of TC, LDL-C, and non-HDL-C in the association of BMI with diabetes risk might largely stem from the absence of an underlying link between TC, LDL-C, and non-HDL-C and diabetes risk, as this did not align with the prerequisite for mediation analysis. Notably, HDL-C and HDL-C-related ratio parameters play a very strong mediating role in BMI-related diabetes risk. In pathological states such as obesity and diabetes, HDL-C function and composition are remodeled, mainly in the form of increased serum glycosylated proteins and oxidized HDL, which in turn reduces the antioxidant and anti-inflammatory effects of HDL [ 54 , 55 ]. Taken together with the current study results, HDL-C may be the most single important lipid parameter in BMI-associated diabetes risk.To our knowledge, this is the first study to dissect the intricate relationship of BMI, lipid parameters, with diabetes risk. The current study demonstrated that the majority of lipid parameters were involved in mediating the association of BMI with the risk of diabetes. Regarding the mechanisms through which lipid parameters mediated the association of BMI with diabetes, several findings based on fundamental research might offer partial explanations: Generally, as BMI increases, serum-free fatty acids tend to rise [ 56 ], and tissues like the liver, skeletal muscle, and pancreas accelerate the uptake and utilization of free fatty acids. However, when these tissues reach a compensatory limit in their uptake and utilization of fatty acids, excess lipids accumulate within cells. On one hand, this lipid toxicity exacerbates lipid accumulation in β-cells, ultimately leading to β-cell apoptosis [ 44 ]; on the other hand, excessive lipid accumulation induces secondary IR in tissues like the liver and skeletal muscle [ 57 ], ultimately culminating in diabetes development. Indeed, the inflammatory response in adipose tissue is also noteworthy. When fatty acid uptake by adipocytes increases, white adipose tissue secretes tumor necrosis factor-alpha (TNF-α), which, through intracellular and extracellular cascades, activates the NF-κB pathway, leading to oxidative stress in adipose tissue, inflammation in β-cells, and hindrance of insulin signal transduction, thereby exacerbating IR [ 57 , 58 , 59 , 60 ]. Furthermore, TNF-α inhibits the expression of the ADIPOQ gene, lowering serum adiponectin levels, which in turn weakens the oxidation process of free fatty acids in skeletal muscle and liver, leading to elevated serum free fatty acid concentrations and exacerbating metabolic disturbances in lipid metabolism [ 61 , 62 ]. Simultaneously, the significant role of adiponectin in exerting anti-apoptotic effects on β-cells should not be overlooked [ 63 ]; decreased adiponectin levels could substantially increase the risk of diabetes. Hence, lipid toxicity, inflammation, and IR likely constitute vital pathological pathways that link lipid parameters with BMI-related diabetes risk.
The present study assessed the mediating role of lipid parameters in the association of BMI with diabetes risk, further ROC analyses showed that lipid parameters combined with HDL-C were significantly improved not only in predicting incident diabetes but also in mediating BMI-related diabetes risk. It is noteworthy that in the current analysis, we identified the RC/HDL-C ratio as the most valuable lipid parameter for assessing diabetes risk and mediating the association of BMI with diabetes risk; therefore, we recommend placing emphasis on the simple yet efficient indicator of RC/HDL-C ratio in diabetes risk screening. Additionally, based on the results of the mediation analysis, it’s essential to emphasize that incorporating lipid levels into the management of diabetes risk, alongside weight management, could be an effective measure to reduce the incidence of diabetes. Existing evidence also suggested that addressing a single risk factor may prove inadequate for achieving favorable clinical outcomes in the context of complex metabolic disorders [ 63 , 64 , 65 , 66 ]. Therefore, implementing multifactorial management targeting the risk factors for diabetes could be a more effective approach, and this viewpoint is supported by a range of completed and ongoing clinical studies. Previous studies have shown that intensive interventions targeting multiple factors, including blood glucose, lipid levels, and blood pressure, among high-risk individuals, have resulted in a significant reduction in the risk of diabetes and related complications [ 66 , 67 , 68 , 69 ]. Among them, the Steno-2 cohort study demonstrated that for diabetic patients, the importance of controlling blood lipids might even surpass that of controlling blood glucose [ 69 ], which underscored the significance of lipid interventions in comprehensive, multifactorial management of diabetes. Dietary intervention is also a crucial measure in comprehensive diabetes management. Researches indicated that daily intake of 15–35 g of fiber-rich foods and consumption of polyunsaturated fatty acids (omega-3 fatty acids) can significantly improve blood glucose and lipid metabolism in diabetic patients and high-risk individuals, while also aiding in weight reduction [ 70 , 71 ]. These findings offer valuable insights for diabetes prevention and management. Monitoring diabetes risk factors and relying on metabolic control to reduce diabetes risk and incidence rates might be a more efficient approach to curbing the diabetes epidemic.
Study strengths and limitations
Our strength lies in quantifying the effects of 8 lipid parameters in the association of BMI with diabetes risk through mediation analysis, thus introducing novel perspectives into the biological mechanisms underlying the link between BMI and diabetes risk. Furthermore, these newfound discoveries offer fresh evidence and viewpoints for diabetes prevention and management, holding significant clinical implications.
Similarly, there are certain limitations of our study that should be acknowledged. (1) Our study found that lipid parameters only partially mediated the association of BMI with diabetes risk, indicating the existence of several unknown potential mediators that necessitate further research for exploration; furthermore, the specific mechanisms by which lipid parameters mediate the association of BMI with diabetes risk also require additional foundational research for validation. (2) Despite controlling for multiple covariates during our study, there remain unmeasured confounding factors that could potentially lead to residual confounding. (3) Some studies have indicated that the use of lipid-lowering drugs (statins) might increase the risk of diabetes [ 72 ], and they can also influence cholesterol and lipid metabolism. However, since the original study excluded subjects using such medications [ 22 ], we couldn’t assess the impact of lipid-lowering drugs on our study results and hope that future research could address this issue. (4) As our participants were solely drawn from the general population in Japan, further validation is required to assess the applicability of the current study’s findings to other ethnic groups.
This study discovered that the majority of lipid parameters mediated the association of BMI with diabetes risk. It is noteworthy that the impact of the RC/HDL-C ratio was the most significant, mediating 40% of the BMI-related diabetes risk. These findings offered new insights into the prevention and treatment of diabetes, and focusing on HDL-C and HDL-C-related lipid ratio parameters may be an essential measure in the comprehensive management of BMI-related diabetes.
Data availability
The datasets analysed during the current study are available in the Dryad repository. [ https://datadryad.org/stash/dataset/doi:10.5061/dryad.8q0p192 ].
Abbreviations
- Body mass index
gamma-glutamyl transferase
tumor necrosis factor-alpha
high-density lipoprotein cholesterol
hazard ratio
diastolic blood pressure
fasting plasma glucose
alanine aminotransferase
low-density lipoprotein cholesterol
insulin resistance
systolic blood pressure
aspartate aminotransferase
waist circumference
triglyceride
glycated hemoglobin A1c
remnant cholesterol
total cholesterol
Chan JC, Malik V, Jia W, Kadowaki T, Yajnik CS, Yoon KH, et al. Diabetes in Asia: epidemiology, risk factors, and pathophysiology. JAMA. 2009;301:2129–40. https://doi.org/10.1001/jama.2009.726 .
Article CAS PubMed Google Scholar
Hu FB. Globalization of diabetes: the role of diet, lifestyle, and genes. Diabetes Care. 2011;34:1249–57. https://doi.org/10.2337/dc11-0442 .
Article PubMed PubMed Central Google Scholar
GBD 2021 Diabetes Collaborators. Global, regional, and national burden of diabetes from 1990 to 2021, with projections of prevalence to 2050: a systematic analysis for the global burden of Disease Study 2021. Lancet. 2023;402:203–34. https://doi.org/10.1016/S0140-6736(23)01301-6 .
Article Google Scholar
Di Cesare M, Sorić M, Bovet P, Miranda JJ, Bhutta Z, Stevens GA, et al. The epidemiological burden of obesity in childhood: a worldwide epidemic requiring urgent action. BMC Med. 2019;17:212. https://doi.org/10.1186/s12916-019-1449-8 .
Prospective Studies Collaboration, Whitlock G, Lewington S, Sherliker P, Clarke R, Emberson J, et al. Body-mass index and cause-specific mortality in 900 000 adults: collaborative analyses of 57 prospective studies. Lancet. 2009;373:1083–96. https://doi.org/10.1016/S0140-6736(09)60318-4 .
Article PubMed Central Google Scholar
Klein S, Gastaldelli A, Yki-Järvinen H, Scherer PE. Why does obesity cause diabetes? Cell Metab. 2022;34:11–20. https://doi.org/10.1016/j.cmet.2021.12.012 .
Article CAS PubMed PubMed Central Google Scholar
Rao G, Powell-Wiley TM, Ancheta I, Hairston K, Kirley K, Lear SA, et al. Identification of obesity and Cardiovascular Risk in ethnically and racially diverse populations: A Scientific Statement from the American Heart Association. Circulation. 2015;132:457–72. https://doi.org/10.1161/CIR.0000000000000223 .
Article PubMed Google Scholar
Li S, Wang Y, Ying Y, Gong Q, Lou G, Liu Y, et al. Independent and Joint associations of BMI and Waist Circumference with the onset of type 2 diabetes Mellitus in Chinese adults: prospective data linkage study. JMIR Public Health Surveill. 2023;9:e39459. https://doi.org/10.2196/39459 .
Zhang FL, Ren JX, Zhang P, Jin H, Qu Y, Yu Y, et al. Strong Association of Waist Circumference (WC), body Mass Index (BMI), Waist-to-height ratio (WHtR), and Waist-to-hip ratio (WHR) with diabetes: a Population-based cross-sectional study in Jilin Province, China. J Diabetes Res. 2021;2021:8812431. https://doi.org/10.1155/2021/8812431 .
Sheng G, Qiu J, Kuang M, Peng N, Xie G, Chen Y, et al. Assessing temporal differences of baseline body mass index, waist circumference, and waist-height ratio in predicting future diabetes. Front Endocrinol (Lausanne). 2023;13:1020253. https://doi.org/10.3389/fendo.2022.1020253 .
Ohno R, Kaneko H, Ueno K, Aoki H, Okada A, Kamiya K, et al. Association of Body Mass Index and its change with Incident Diabetes Mellitus. J Clin Endocrinol Metab. 2023;dgad374. https://doi.org/10.1210/clinem/dgad374 .
Nano J, Dhana K, Asllanaj E, Sijbrands E, Ikram MA, Dehghan A, et al. Trajectories of BMI before diagnosis of type 2 diabetes: the Rotterdam Study. Obes (Silver Spring). 2020;28:1149–56. https://doi.org/10.1002/oby.22802 .
Article CAS Google Scholar
Chiu CJ, Li SL, Wu CH, Du YF. BMI trajectories as a harbinger of pre-diabetes or underdiagnosed diabetes: an 18-Year retrospective cohort study in Taiwan. J Gen Intern Med. 2016;31:1156–63. https://doi.org/10.1007/s11606-016-3750-y .
Chehade JM, Gladysz M, Mooradian AD. Dyslipidemia in type 2 diabetes: prevalence, pathophysiology, and management. Drugs. 2013;73:327–39. https://doi.org/10.1007/s40265-013-0023-5 .
Szukiewicz D. Molecular mechanisms for the vicious cycle between Insulin Resistance and the inflammatory response in obesity. Int J Mol Sci. 2023;24:9818. https://doi.org/10.3390/ijms24129818 .
Dong Q, Sidra S, Gieger C, Wang-Sattler R, Rathmann W, Prehn C, et al. Metabolic signatures elucidate the Effect of Body Mass Index on type 2 diabetes. Metabolites. 2023;13:227. https://doi.org/10.3390/metabo13020227 .
Xuan Q, Hu C, Zhang Y, Wang Q, Zhao X, Liu X, et al. Serum lipidomics profiles reveal potential lipid markers for prediabetes and type 2 diabetes in patients from multiple communities. Front Endocrinol (Lausanne). 2022;13:966823. https://doi.org/10.3389/fendo.2022.966823 .
Kim YJ, Lee HS, Kim YK, Park S, Kim JM, Yun JH, et al. Association of Metabolites with obesity and type 2 diabetes based on FTO Genotype. PLoS ONE. 2016;11:e0156612. https://doi.org/10.1371/journal.pone.0156612 .
Stevens VL, Carter BD, McCullough ML, Campbell PT, Wang Y. Metabolomic Profiles Associated with BMI, Waist circumference, and diabetes and inflammation biomarkers in women. Obes (Silver Spring). 2020;28:187–96. https://doi.org/10.1002/oby.22670 .
Wang SM, Yang RY, Wang M, Ji FS, Li HX, Tang YM, et al. Identification of serum metabolites associated with obesity and traditional risk factors for metabolic disease in Chinese adults. Nutr Metab Cardiovasc Dis. 2018;28:112–8. https://doi.org/10.1016/j.numecd.2017.09.009 .
Wang Y, Wu P, Huang Y, Ye Y, Yang X, Sun F, et al. BMI and lipidomic biomarkers with risk of gestational diabetes in pregnant women. Obes (Silver Spring). 2022;30:2044–54. https://doi.org/10.1002/oby.23517 .
Okamura T, Hashimoto Y, Hamaguchi M, Obora A, Kojima T, Fukui M. Ectopic fat obesity presents the greatest risk for incident type 2 diabetes: a population-based longitudinal study. Int J Obes (Lond). 2019;43:139–48. https://doi.org/10.1038/s41366-018-0076-3 .
Hashimoto Y, Hamaguchi M, Kojima T, Ohshima Y, Ohbora A, Kato T, et al. Modest alcohol consumption reduces the incidence of fatty liver in men: a population-based large-scale cohort study. J Gastroenterol Hepatol. 2015;30:546–52. https://doi.org/10.1111/jgh.12786 .
Hamaguchi M, Kojima T, Itoh Y, Harano Y, Fujii K, Nakajima T, et al. The severity of ultrasonographic findings in nonalcoholic fatty liver disease reflects the metabolic syndrome and visceral fat accumulation. Am J Gastroenterol. 2007;102:2708–15. https://doi.org/10.1111/j.1572-0241.2007.01526.x .
Chen Y, Zhang X, Pan B, Jin X, Yao H, Chen B, et al. A modified formula for calculating low-density lipoprotein cholesterol values. Lipids Health Dis. 2010;9:52. https://doi.org/10.1186/1476-511X-9-52 .
Sampson M, Ling C, Sun Q, Harb R, Ashmaig M, Warnick R, et al. A New equation for calculation of Low-Density Lipoprotein Cholesterol in patients with normolipidemia and/or hypertriglyceridemia. JAMA Cardiol. 2020;5:540–8. https://doi.org/10.1001/jamacardio.2020.0013 .
Kachekouche Y, Dali-Sahi M, Bendaoud R, Dennouni-Medjati N, Abderahim M. Predictive value of non-HDL cholesterol for cardiovascular disease in a population in far western Algeria with type 2 diabetes. Diabetes Metab Syndr. 2019;13:826–829. https://doi.org/10.1016/j.dsx.2018.12.002 .
Xie G, Zhong Y, Yang S, Zou Y. Remnant Cholesterol is an Independent Predictor of New-Onset Diabetes: A Single-Center Cohort Study. Diabetes Metab Syndr Obes. 2021;14:4735–4745. https://doi.org/10.2147/DMSO.S341285 .
Hong M, Ling Y, Lu Z, Liu Y, Gu P, Shao J, et al. Contribution and interaction of the low-density lipoprotein cholesterol to high-density lipoprotein cholesterol ratio and triglyceride to diabetes in hypertensive patients: a cross-sectional study. J Diabetes Investig. 2019;10:131–8. https://doi.org/10.1111/jdi.12856 .
Zhang N, Hu X, Zhang Q, Bai P, Cai M, Zeng TS, et al. Non-high-density lipoprotein cholesterol: high-density lipoprotein cholesterol ratio is an independent risk factor for diabetes mellitus: results from a population-based cohort study. J Diabetes. 2018;10:708–14. https://doi.org/10.1111/1753-0407.12650 .
Khaloo P, Hasheminia M, Tohidi M, Abdi H, Mansournia MA, Azizi F, et al. Impact of 3-year changes in lipid parameters and their ratios on incident type 2 diabetes: Tehran lipid and glucose study. Nutr Metab (Lond). 2018;15:50. https://doi.org/10.1186/s12986-018-0287-6 .
Zou Y, Hu C, Kuang M, Chai Y. Remnant cholesterol/high-density lipoprotein cholesterol ratio is a new powerful tool for identifying non-alcoholic fatty liver disease. BMC Gastroenterol. 2022;22:134. https://doi.org/10.1186/s12876-022-02216-x .
American Diabetes Association. Standards of medical care in diabetes–2011. Diabetes Care. 2011;34(Suppl 1):S11–61. https://doi.org/10.2337/dc11-S011 .
Article CAS PubMed Central Google Scholar
Sato T, Matsuyama Y. Marginal structural models as a tool for standardization. Epidemiology. 2003;14:680–6. https://doi.org/10.1097/01.EDE.0000081989.82616.7d .
Muanda FT, Weir MA, Bathini L, Blake PG, Chauvin K, Dixon SN, et al. Association of Baclofen with Encephalopathy in patients with chronic kidney disease. JAMA. 2019;322:1987–95. https://doi.org/10.1001/jama.2019.17725 .
Kim JH. Multicollinearity and misleading statistical results. Korean J Anesthesiol. 2019;72:558–69. https://doi.org/10.4097/kja.19087 .
Lee H, Cashin AG, Lamb SE, Hopewell S, Vansteelandt S, VanderWeele TJ, et al. A Guideline for reporting mediation analyses of randomized trials and observational studies: the AGReMA Statement. JAMA. 2021;326:1045–56. https://doi.org/10.1001/jama.2021.14075 .
VanderWeele TJ. Mediation analysis: a practitioner’s guide. Annu Rev Public Health. 2016;37:17–32. https://doi.org/10.1146/annurev-publhealth-032315-021402 .
Campagna D, Alamo A, Di Pino A, Russo C, Calogero AE, Purrello F, et al. Smoking and diabetes: dangerous liaisons and confusing relationships. Diabetol Metab Syndr. 2019;11:85. https://doi.org/10.1186/s13098-019-0482-2 .
Li MJ, Ren J, Zhang WS, Jiang CQ, Jin YL, Lam TH, et al. Association of alcohol drinking with incident type 2 diabetes and pre-diabetes: the Guangzhou Biobank Cohort Study. Diabetes Metab Res Rev. 2022;38:e3548. https://doi.org/10.1002/dmrr.3548 .
Oosterveen E, Tzelepis F, Ashton L, Hutchesson MJ. A systematic review of eHealth behavioral interventions targeting smoking, nutrition, alcohol, physical activity and/or obesity for young adults. Prev Med. 2017;99:197–206. https://doi.org/10.1016/j.ypmed.2017.01.009 .
Vekic J, Zeljkovic A, Stefanovic A, Jelic-Ivanovic Z, Spasojevic-Kalimanovska V. Obesity and dyslipidemia. Metabolism. 2019;92:71–81. https://doi.org/10.1016/j.metabol.2018.11.005 .
Hill MJ, Metcalfe D, McTernan PG. Obesity and diabetes: lipids, ‘nowhere to run to’. Clin Sci (Lond). 2009;116:113–23. https://doi.org/10.1042/CS20080050 .
Shimabukuro M, Zhou YT, Levi M, Unger RH. Fatty acid-induced beta cell apoptosis: a link between obesity and diabetes. Proc Natl Acad Sci U S A. 1998;95:2498–502. https://doi.org/10.1073/pnas.95.5.2498 .
Odegaard AO, Koh WP, Vazquez G, Arakawa K, Lee HP, Yu MC, et al. BMI and diabetes risk in Singaporean Chinese. Diabetes Care. 2009;32:1104–6. https://doi.org/10.2337/dc08-1674 .
Yang J, Wang F, Wang J, Han X, Hu H, Yu C, et al. Using different anthropometric indices to assess prediction ability of type 2 diabetes in elderly population: a 5 year prospective study. BMC Geriatr. 2018;18:218. https://doi.org/10.1186/s12877-018-0912-2 .
Huang J, Lin H, Wang S, Li M, Wang T, Zhao Z, et al. Association between serum LDL-C concentrations and risk of diabetes: a prospective cohort study. J Diabetes. 2023. https://doi.org/10.1111/1753-0407.13440 .
Sheng G, Kuang M, Yang R, Zhong Y, Zhang S, Zou Y. Evaluation of the value of conventional and unconventional lipid parameters for predicting the risk of diabetes in a non-diabetic population. J Transl Med. 2022;20:266. https://doi.org/10.1186/s12967-022-03470-z .
Hadaegh F, Hatami M, Tohidi M, Sarbakhsh P, Saadat N, Azizi F. Lipid ratios and appropriate cut off values for prediction of diabetes: a cohort of Iranian men and women. Lipids Health Dis. 2010;9:85. https://doi.org/10.1186/1476-511X-9-85 .
Feskens EJ, Kromhout D. Cardiovascular risk factors and the 25-year incidence of diabetes mellitus in middle-aged men. The Zutphen Study. Am J Epidemiol. 1989;130:1101–8. https://doi.org/10.1093/oxfordjournals.aje.a115437 .
Wilson PW, Meigs JB, Sullivan L, Fox CS, Nathan DM, D’Agostino RB, Sr. Prediction of incident diabetes mellitus in middle-aged adults: the Framingham offspring study. Arch Intern Med. 2007;167:1068–74. https://doi.org/10.1001/archinte.167.10.1068 .
Zhu XW, Deng FY, Lei SF. Meta-analysis of Atherogenic Index of Plasma and other lipid parameters in relation to risk of type 2 diabetes mellitus. Prim Care Diabetes. 2015;9:60–7. https://doi.org/10.1016/j.pcd.2014.03.007 .
Liu L, Li Q, Yuan Z, Zhao M, Zhang X, Zhang H, et al. Non-high-density lipoprotein cholesterol is more informative than traditional cholesterol indices in predicting diabetes risk for women with normal glucose tolerance. J Diabetes Investig. 2018;9:1304–11. https://doi.org/10.1111/jdi.12837 .
Kashyap SR, Osme A, Ilchenko S, Golizeh M, Lee K, Wang S, et al. Glycation reduces the Stability of ApoAI and increases HDL dysfunction in Diet-controlled type 2 diabetes. J Clin Endocrinol Metab. 2018;103:388–96. https://doi.org/10.1210/jc.2017-01551 .
Zhang Y, McGillicuddy FC, Hinkle CC, O’Neill S, Glick JM, Rothblat GH, et al. Adipocyte modulation of high-density lipoprotein cholesterol. Circulation. 2010;121:1347–55. https://doi.org/10.1161/CIRCULATIONAHA.109.897330 .
Unger RH. Lipotoxicity in the pathogenesis of obesity-dependent NIDDM. Genetic and clinical implications. Diabetes. 1995;44:863–70. https://doi.org/10.2337/diab.44.8.863 .
Sethi JK, Vidal-Puig AJ. Thematic review series: adipocyte biology. Adipose tissue function and plasticity orchestrate nutritional adaptation. J Lipid Res. 2007;48:1253–62. https://doi.org/10.1194/jlr.R700005-JLR200 .
Baker RG, Hayden MS, Ghosh S. NF-κB, inflammation, and metabolic disease. Cell Metab. 2011;13:11–22. https://doi.org/10.1016/j.cmet.2010.12.008 .
Rehman K, Akash MS. Mechanisms of inflammatory responses and development of insulin resistance: how are they interlinked? J Biomed Sci. 2016;23:87. https://doi.org/10.1186/s12929-016-0303-y .
Kern PA, Di Gregorio GB, Lu T, Rassouli N, Ranganathan G. Adiponectin expression from human adipose tissue: relation to obesity, insulin resistance, and tumor necrosis factor-alpha expression. Diabetes. 2003;52:1779–85. https://doi.org/10.2337/diabetes.52.7.1779 .
Chandran M, Phillips SA, Ciaraldi T, Henry RR. Adiponectin: more than just another fat cell hormone? Diabetes Care. 2003;26:2442–50. https://doi.org/10.2337/diacare.26.8.2442 .
Díez JJ, Iglesias P. The role of the novel adipocyte-derived hormone adiponectin in human disease. Eur J Endocrinol. 2003;148:293–300. https://doi.org/10.1530/eje.0.1480293 .
Turer AT, Scherer PE. Adiponectin: mechanistic insights and clinical implications. Diabetologia. 2012;55:2319–26. https://doi.org/10.1007/s00125-012-2598-x .
Douketis JD, Macie C, Thabane L, Williamson DF. Systematic review of long-term weight loss studies in obese adults: clinical significance and applicability to clinical practice. Int J Obes (Lond). 2005;29:1153–67. https://doi.org/10.1038/sj.ijo.0802982 .
Gray LJ, Cooper N, Dunkley A, Warren FC, Ara R, Abrams K, et al. A systematic review and mixed treatment comparison of pharmacological interventions for the treatment of obesity. Obes Rev. 2012;13:483–98. https://doi.org/10.1111/j.1467-789X.2011.00981.x .
Rucker D, Padwal R, Li SK, Curioni C, Lau DC. Long term pharmacotherapy for obesity and overweight: updated meta-analysis. BMJ. 2007;335:1194–9. https://doi.org/10.1136/bmj.39385.413113.25 .
Sasako T, Yamauchi T, Ueki K. Intensified multifactorial intervention in patients with type 2 diabetes Mellitus. Diabetes Metab J. 2023;47:185–97. https://doi.org/10.4093/dmj.2022.0325 .
Wang Y, Guan Q, Hou X, Zhang X, Zhang H, Xu C, et al. Multifactorial intervention on type 2 diabetes (MIDiab) study: a multicenter, open-label, randomized, parallel controlled, community trial. J Diabetes. 2020;12:862–4. https://doi.org/10.1111/1753-0407.13081 .
Gaede P, Pedersen O. Intensive integrated therapy of type 2 diabetes: implications for long-term prognosis. Diabetes. 2004;53(Suppl 3):S39–47. https://doi.org/10.2337/diabetes.53.suppl_3.s39 .
Reynolds AN, Akerman AP, Mann J. Dietary fibre and whole grains in diabetes management: systematic review and meta-analyses. PLoS Med. 2020;17:e1003053. https://doi.org/10.1371/journal.pmed.1003053 .
Mori TA, Bao DQ, Burke V, Puddey IB, Watts GF, Beilin LJ. Dietary fish as a major component of a weight-loss diet: effect on serum lipids, glucose, and insulin metabolism in overweight hypertensive subjects. Am J Clin Nutr. 1999;70:817–25. https://doi.org/10.1093/ajcn/70.5.817 .
Casula M, Mozzanica F, Scotti L, Tragni E, Pirillo A, Corrao G, et al. Statin use and risk of new-onset diabetes: a meta-analysis of observational studies. Nutr Metab Cardiovasc Dis. 2017;27:396–406. https://doi.org/10.1016/j.numecd.2017.03.001 .
Download references
Acknowledgements
Thanks to Professor Okamura’s team for their great efforts in data collection and collation.
This work was supported by the National Natural Science Foundation of China [82060067, 81660359], the Natural Science Foundation of Jiangxi Province [20232BAB216004, 20203BBGL73233], Traditional Chinese Medicine Science and Technology Project of Jiangxi province [2020B0109, 2023B1218], and the First Affiliated Hospital of Nanchang University Young Talents Research and Cultivation Project [YFYPY202122].
Author information
Song Lu, Qun Wang and Hengcheng Lu contributed equally to this work.
Authors and Affiliations
Department of Cardiology, The First Affiliated Hospital of Nanchang University, Jiangxi Medical College, Nanchang University, Nanchang, 330006, China
Song Lu, Maobin Kuang, Min Zhang & Xiaoping Peng
Jiangxi Cardiovascular Research Institute, Jiangxi Provincial People’s Hospital, The First Affiliated Hospital of Nanchang Medical College, Nanchang, 330006, China
Qun Wang, Hengcheng Lu, Maobin Kuang, Guotai Sheng & Yang Zou
Jiangxi Hypertension Research Institute, Nanchang, 330006, China
Min Zhang & Xiaoping Peng
You can also search for this author in PubMed Google Scholar
Contributions
YZ and XP-P: Conceptualization, supervision and project administration.YZ and GT-S: Methodology.SL, QW and HC-L: Writing-original draft preparation.YZ, GT-S and XP-P: Writing-reviewing and editing.SL, QW, HC-L and MB-K: Software.SL, MZ and MB-K: Formal analysis and validation.SL, QW, HC-L, MZ and MB-K: Data curation.All authors read and approved the final manuscript.
Corresponding authors
Correspondence to Yang Zou or Xiaoping Peng .
Ethics declarations
Ethics approval and consent to participate.
The NAGALA project has been authorized by the Ethics Committee of Murakami Memorial Hospital, and obtained informed consent from all subjects for their data usage. This study was a secondary analysis of the NAGALA cohort study, which has been approved by the Ethics Committee of Jiangxi Province People’s Hospital. Furthermore, since the publicly available dataset has been anonymized, the Ethics Committee of Jiangxi Provincial People’s Hospital waived the need for informed consent from the subjects.
Consent for publication
Not applicable.
Competing interests
The authors declare no competing interests.
Additional information
Publisher’s note.
Springer Nature remains neutral with regard to jurisdictional claims in published maps and institutional affiliations.
Electronic supplementary material
Below is the link to the electronic supplementary material.
Supplementary Material 1
Rights and permissions.
Open Access This article is licensed under a Creative Commons Attribution 4.0 International License, which permits use, sharing, adaptation, distribution and reproduction in any medium or format, as long as you give appropriate credit to the original author(s) and the source, provide a link to the Creative Commons licence, and indicate if changes were made. The images or other third party material in this article are included in the article’s Creative Commons licence, unless indicated otherwise in a credit line to the material. If material is not included in the article’s Creative Commons licence and your intended use is not permitted by statutory regulation or exceeds the permitted use, you will need to obtain permission directly from the copyright holder. To view a copy of this licence, visit http://creativecommons.org/licenses/by/4.0/ . The Creative Commons Public Domain Dedication waiver ( http://creativecommons.org/publicdomain/zero/1.0/ ) applies to the data made available in this article, unless otherwise stated in a credit line to the data.
Reprints and permissions
About this article
Cite this article.
Lu, S., Wang, Q., Lu, H. et al. Lipids as potential mediators linking body mass index to diabetes: evidence from a mediation analysis based on the NAGALA cohort. BMC Endocr Disord 24 , 66 (2024). https://doi.org/10.1186/s12902-024-01594-5
Download citation
Received : 06 March 2024
Accepted : 30 April 2024
Published : 10 May 2024
DOI : https://doi.org/10.1186/s12902-024-01594-5
Share this article
Anyone you share the following link with will be able to read this content:
Sorry, a shareable link is not currently available for this article.
Provided by the Springer Nature SharedIt content-sharing initiative
- Lipid parameters
- Indirect effect
- Mediation analyses
BMC Endocrine Disorders
ISSN: 1472-6823
- General enquiries: [email protected]
Open Access is an initiative that aims to make scientific research freely available to all. To date our community has made over 100 million downloads. It’s based on principles of collaboration, unobstructed discovery, and, most importantly, scientific progression. As PhD students, we found it difficult to access the research we needed, so we decided to create a new Open Access publisher that levels the playing field for scientists across the world. How? By making research easy to access, and puts the academic needs of the researchers before the business interests of publishers.
We are a community of more than 103,000 authors and editors from 3,291 institutions spanning 160 countries, including Nobel Prize winners and some of the world’s most-cited researchers. Publishing on IntechOpen allows authors to earn citations and find new collaborators, meaning more people see your work not only from your own field of study, but from other related fields too.
Brief introduction to this section that descibes Open Access especially from an IntechOpen perspective
Want to get in touch? Contact our London head office or media team here
Our team is growing all the time, so we’re always on the lookout for smart people who want to help us reshape the world of scientific publishing.
Home > Books > Biomedicine
Body-mass Index and Health

Book metrics overview
7,276 Chapter Downloads
Impact of this book and its chapters
Total Chapter Downloads on intechopen.com

Total Chapter Views on intechopen.com
Overall attention for this book and its chapters
Book Citations
Total Chapter Citations
Academic Editor
Istanbul University , Turkey
Published 13 February 2019
Doi 10.5772/intechopen.73958
ISBN 978-1-78985-006-2
Print ISBN 978-1-78985-005-5
eBook (PDF) ISBN 978-1-83962-016-4
Copyright year 2019
Number of pages 110
The body mass index has an important place in weight control. Attention should be paid to the regularization of anthropometric measures and to physical activity to protect from increasing obesity that is associated with chronic noncommunicable conditions, such as diabetes mellitus, cancers and cardiovascular diseases. Also, attention should be paid to the countries that are developing. The daily i...
The body mass index has an important place in weight control. Attention should be paid to the regularization of anthropometric measures and to physical activity to protect from increasing obesity that is associated with chronic noncommunicable conditions, such as diabetes mellitus, cancers and cardiovascular diseases. Also, attention should be paid to the countries that are developing. The daily intake of calories, carbohydrates, oils and proteins, fibers, vitamins and minerals and clean water is essential for all individuals, especially for children and for pregnant women.
By submitting the form you agree to IntechOpen using your personal information in order to fulfil your library recommendation. In line with our privacy policy we won’t share your details with any third parties and will discard any personal information provided immediately after the recommended institution details are received. For further information on how we protect and process your personal information, please refer to our privacy policy .
Cite this book
There are two ways to cite this book:
Edited Volume and chapters are indexed in
Table of contents.
By Ayşe Emel Önal
By Niranjan Bhattacharya and Priyodarshi Sengupta
By Mariana Petrova Genova, Bisera Dimitrova Atanasova and Katya Nikolova Todorova-Ananieva
By Li Liu, Hui Zou and Ya-Huan Li
By Nuri Faruk Aykan, Mehmet Artac and Tahsin Özatli
IMPACT OF THIS BOOK AND ITS CHAPTERS
7,276 Total Chapter Downloads
1,203 Total Chapter Views
5 Crossref Citations
9 Dimensions Citations
2 Altmetric Score
Order a print copy of this book
Available on

Delivered by
£119 (ex. VAT)*
Hardcover | Printed Full Colour
FREE SHIPPING WORLDWIDE
* Residents of European Union countries need to add a Book Value-Added Tax Rate based on their country of residence. Institutions and companies, registered as VAT taxable entities in their own EU member state, will not pay VAT by providing IntechOpen with their VAT registration number. This is made possible by the EU reverse charge method.
As an IntechOpen contributor, you can buy this book for an Exclusive Author price with discounts from 30% to 50% on retail price.
Log in to your Author Panel to purchase a book at the discounted price.
For any assistance during ordering process, contact us at [email protected]
Related books
Tertiary care.
Edited by Ayşe Emel Önal
Healthcare Access
Air quality and health, primary health care, biofeedback.
Edited by Mark Schwartz
Frontiers in Clinical Trials
Edited by Xianli Lv
Infrared Spectroscopy
Edited by Theophile Theophanides
Frontiers in Guided Wave Optics and Optoelectronics
Edited by Bishnu Pal
Abiotic Stress in Plants
Edited by Arun Shanker
Anopheles mosquitoes
Edited by Sylvie Manguin
Call for authors
Submit your work to intechopen.

Project 1 : Body-Mass Index
Cite this chapter.
4474 Accesses
It’s project time! Body-mass index (BMI) is a measurement model health-care professionals use to determine whether a person is overweight, and if so, by how much. To compute BMI, you need a person’s weight in kilograms and height in meters. The BMI is simply weight/ height 2 , converted to a unitless value.
This is a preview of subscription content, log in via an institution to check access.
Access this chapter
- Available as PDF
- Read on any device
- Instant download
- Own it forever
- Compact, lightweight edition
- Dispatched in 3 to 5 business days
- Free shipping worldwide - see info
Tax calculation will be finalised at checkout
Purchases are for personal use only
Institutional subscriptions

Rights and permissions
Reprints and permissions
Copyright information
© 2009 Ray Lischner
About this chapter
(2009). Project 1 : Body-Mass Index. In: Exploring C++. Apress. https://doi.org/10.1007/978-1-4302-0352-0_26
Download citation
DOI : https://doi.org/10.1007/978-1-4302-0352-0_26
Publisher Name : Apress
Print ISBN : 978-1-59059-749-1
Online ISBN : 978-1-4302-0352-0
eBook Packages : Professional and Applied Computing Professional and Applied Computing (R0) Apress Access Books
Share this chapter
Anyone you share the following link with will be able to read this content:
Sorry, a shareable link is not currently available for this article.
Provided by the Springer Nature SharedIt content-sharing initiative
- Publish with us
Policies and ethics
- Find a journal
- Track your research
- Introduction
- Methodology
- Conclusions
- Article Information
Linear regression models were fitted and adjusted for maternal education level, maternal body mass index (BMI), total minutes of physical activity per week, breastfeeding, center size, and NOVA classification system groups 2 and 3. HDL indicates high-density lipoprotein; HOMA-IR, homeostasis model assessment for insulin resistance; LDL, low-density lipoprotein.
a For HDL cholesterol, a positive β coefficient signifies low risk.
eAppendix. Additional Study Assessments
eTable 1. Distribution of FFQ Items Into 4 Groups According to the Degree of Their Processing Established by NOVA Classification System
eFigure 1. Flow Chart of the Children Included in the Analysis
eTable 2. Association Between Energy-Adjusted Ultraprocessed Food Consumption in Tertiles (in g/day) and CVD Risk Factors at Baseline
eFigure 2. Linear Regression Models Replacing 100 g of UPF With 100 g of Unprocessed/Minimally Processed Foods
eTable 3. Maternal Socioprofessional Stratified Regression Association Between 1-SD Increment of Energy-Adjusted Ultraprocessed Food Consumption (in g/day) and CVD Risk Factors at Baseline
eTable 4. Maternal Education Level Stratified Regression Association Between 1-SD Increment of Energy-Adjusted Ultraprocessed Food Consumption (in g/day) and CVD Risk Factors at Baseline
Data Sharing Statement
See More About
Sign up for emails based on your interests, select your interests.
Customize your JAMA Network experience by selecting one or more topics from the list below.
- Academic Medicine
- Acid Base, Electrolytes, Fluids
- Allergy and Clinical Immunology
- American Indian or Alaska Natives
- Anesthesiology
- Anticoagulation
- Art and Images in Psychiatry
- Artificial Intelligence
- Assisted Reproduction
- Bleeding and Transfusion
- Caring for the Critically Ill Patient
- Challenges in Clinical Electrocardiography
- Climate and Health
- Climate Change
- Clinical Challenge
- Clinical Decision Support
- Clinical Implications of Basic Neuroscience
- Clinical Pharmacy and Pharmacology
- Complementary and Alternative Medicine
- Consensus Statements
- Coronavirus (COVID-19)
- Critical Care Medicine
- Cultural Competency
- Dental Medicine
- Dermatology
- Diabetes and Endocrinology
- Diagnostic Test Interpretation
- Drug Development
- Electronic Health Records
- Emergency Medicine
- End of Life, Hospice, Palliative Care
- Environmental Health
- Equity, Diversity, and Inclusion
- Facial Plastic Surgery
- Gastroenterology and Hepatology
- Genetics and Genomics
- Genomics and Precision Health
- Global Health
- Guide to Statistics and Methods
- Hair Disorders
- Health Care Delivery Models
- Health Care Economics, Insurance, Payment
- Health Care Quality
- Health Care Reform
- Health Care Safety
- Health Care Workforce
- Health Disparities
- Health Inequities
- Health Policy
- Health Systems Science
- History of Medicine
- Hypertension
- Images in Neurology
- Implementation Science
- Infectious Diseases
- Innovations in Health Care Delivery
- JAMA Infographic
- Law and Medicine
- Leading Change
- Less is More
- LGBTQIA Medicine
- Lifestyle Behaviors
- Medical Coding
- Medical Devices and Equipment
- Medical Education
- Medical Education and Training
- Medical Journals and Publishing
- Mobile Health and Telemedicine
- Narrative Medicine
- Neuroscience and Psychiatry
- Notable Notes
- Nutrition, Obesity, Exercise
- Obstetrics and Gynecology
- Occupational Health
- Ophthalmology
- Orthopedics
- Otolaryngology
- Pain Medicine
- Palliative Care
- Pathology and Laboratory Medicine
- Patient Care
- Patient Information
- Performance Improvement
- Performance Measures
- Perioperative Care and Consultation
- Pharmacoeconomics
- Pharmacoepidemiology
- Pharmacogenetics
- Pharmacy and Clinical Pharmacology
- Physical Medicine and Rehabilitation
- Physical Therapy
- Physician Leadership
- Population Health
- Primary Care
- Professional Well-being
- Professionalism
- Psychiatry and Behavioral Health
- Public Health
- Pulmonary Medicine
- Regulatory Agencies
- Reproductive Health
- Research, Methods, Statistics
- Resuscitation
- Rheumatology
- Risk Management
- Scientific Discovery and the Future of Medicine
- Shared Decision Making and Communication
- Sleep Medicine
- Sports Medicine
- Stem Cell Transplantation
- Substance Use and Addiction Medicine
- Surgical Innovation
- Surgical Pearls
- Teachable Moment
- Technology and Finance
- The Art of JAMA
- The Arts and Medicine
- The Rational Clinical Examination
- Tobacco and e-Cigarettes
- Translational Medicine
- Trauma and Injury
- Treatment Adherence
- Ultrasonography
- Users' Guide to the Medical Literature
- Vaccination
- Venous Thromboembolism
- Veterans Health
- Women's Health
- Workflow and Process
- Wound Care, Infection, Healing
Get the latest research based on your areas of interest.
Others also liked.
- Download PDF
- X Facebook More LinkedIn
Khoury N , Martínez MÁ , Garcidueñas-Fimbres TE, et al. Ultraprocessed Food Consumption and Cardiometabolic Risk Factors in Children. JAMA Netw Open. 2024;7(5):e2411852. doi:10.1001/jamanetworkopen.2024.11852
Manage citations:
© 2024
- Permissions
Ultraprocessed Food Consumption and Cardiometabolic Risk Factors in Children
- 1 Universitat Rovira i Virgili Departament de Bioquímica i Biotecnologia, Unitat de Nutrició Humana, ANUT-DSM group, Spain
- 2 Institut d’Investigació Sanitària Pere Virgili, Reus, Spain
- 3 Consorcio Centro de Investigación Biomédica en Red, M. P. Fisiopatología de la Obesidad y Nutrición, Instituto de Salud Carlos III, Madrid, Spain
- 4 Metabolism and Investigation Unit, Maimónides Institute of Biomedicine Research of Córdoba, Reina Sofia University Hospital, University of Córdoba, Córdoba, Spain
- 5 Primary Care Interventions to Prevent Maternal and Child Chronic Diseases of Perinatal and Developmental Origin, Instituto de Salud Carlos III, Madrid, Spain
- 6 Unit of Pediatric Gastroenterology, Hepatology and Nutrition, Pediatric Service, Hospital Clínico Universitario de Santiago, Santiago de Compostela, Spain
- 7 Pediatric Nutrition Research Group, Health Research Institute of Santiago de Compostela, Unit of Investigation in Nutrition, Growth and Human Development of Galicia-Universidad de Santiago de Compostela, Santiago de Compostela, Spain
- 8 Growth, Exercise, Nutrition and Development Research Group, University of Zaragoza, Spain
- 9 Instituto Agroalimentario de Aragón, University of Zaragoza, Spain
- 10 Instituto de Investigación Sanitaria de Aragón, Zaragoza, Spain
- 11 Center for Nutrition Research, Faculty of Pharmacy and Nutrition, Department of Nutrition, Food Science and Physiology, University of Navarra, Pamplona, Spain
- 12 Navarra Medical Research Institute, Pamplona, Spain
- 13 Department of Preventive Medicine and Public Health, University of Valencia, Spain
- 14 Hospital del Mar Medical Research Institute, Barcelona, Spain
- 15 Centre d’Atenció Primària, Institut Català de la Salut, Reus, Spain
- 16 Pediatrics, Nutrition, and Development Research Unit, Universitat Rovira I Virgili, Reus, Spain
Question What is the association of consuming ultraprocessed foods (UPFs) with cardiometabolic risk factors in children?
Findings In this cross-sectional study of 1426 children, higher consumption of UPFs was positively associated with body mass index, waist circumference, fat mass index, and fasting plasma glucose and negatively associated with high-density lipoprotein cholesterol concentrations.
Meaning These findings highlight the need for public health initiatives to promote the replacement of UPFs with unprocessed or minimally processed foods.
Importance High intake of ultraprocessed foods (UPFs) has been associated with higher cardiometabolic risk in adults; however, the evidence in children is limited.
Objective To investigate the association between UPF consumption and cardiometabolic risk factors in the Childhood Obesity Risk Assessment Longitudinal Study (CORALS).
Design, Setting, and Participants This baseline cross-sectional analysis was conducted using the data of CORALS participants recruited between March 22, 2019, and June 30, 2022. Preschool children (aged 3-6 years) were recruited from schools and centers in 7 cities in Spain. Inclusion criteria included informed consent signed by parents or caregivers and having a completed a set of questionnaires about the child’s prenatal history at home. Exclusion criteria included low command of Spanish or unstable residence.
Exposure Energy-adjusted UPF consumption (in grams per day) from food frequency questionnaires and based on the NOVA food classification system.
Main Outcomes and Measures Age- and sex-specific z scores of adiposity parameters (body mass index [BMI], fat mass index, waist-to-height ratio, and waist circumference) and cardiometabolic parameters (diastolic and systolic blood pressure, fasting plasma glucose, homeostasis model assessment for insulin resistance, high-density and low-density lipoprotein cholesterol, and triglycerides) were estimated using linear regression models.
Results Of 1509 enrolled CORALS participants, 1426 (mean [SD] age, 5.8 [1.1] years; 698 boys [49.0%]) were included in this study. Mothers of children with high UPF consumption were younger, had a higher BMI, were more likely to have overweight or obesity, and had lower education levels and employment rates. Compared with participants in the lowest tertile of energy-adjusted UPF consumption, those in the highest tertile showed higher z scores of BMI (β coefficient, 0.20; 95% CI, 0.05-0.35), waist circumference (β coefficient, 0.20; 95% CI, 0.05-0.35), fat mass index (β coefficient, 0.17; 95% CI, 0.00-0.32), and fasting plasma glucose (β coefficient, 0.22; 95% CI, 0.06-0.37) and lower z scores for HDL cholesterol (β coefficient, −0.19; 95% CI, −0.36 to −0.02). One-SD increments in energy-adjusted UPF consumption were associated with higher z scores for BMI (β coefficient, 0.11; 95% CI, 0.05-0.17), waist circumference (β coefficient, 0.09; 95% CI, 0.02-0.15), fat mass index (β coefficient, 0.11; 95% CI, 0.04-1.18), and fasting plasma glucose (β coefficient, 0.10; 95% CI, 0.03-0.17) and lower HDL cholesterol (β coefficient, −0.07; 95% CI, −0.15 to −0.00). Substituting 100 g of UPFs with 100 g of unprocessed or minimally processed foods was associated with lower z scores of BMI (β coefficient, −0.03; 95% CI, −0.06 to −0.01), fat mass index (β coefficient, −0.03; 95% CI, −0.06 to 0.00), and fasting plasma glucose (β coefficient, −0.04; 95% CI, −0.07 to −0.01).
Conclusions and Relevance These findings suggest that high UPF consumption in young children is associated with adiposity and other cardiometabolic risk factors, highlighting the need for public health initiatives to promote the replacement of UPFs with unprocessed or minimally processed foods.
The presence of abnormal cardiometabolic risk factors often begins in childhood, highlighting the importance of identifying and controlling them early to delay or prevent cardiovascular disease (CVD) in the future. 1 Modifiable risk factors (eg, diet and physical activity) may contribute to the development of recognized cardiometabolic risk factors. 2 - 4 Emerging studies have shed light on the potential role of ultraprocessed foods (UPFs) in determining the risk of chronic diseases, 5 - 7 independent of their nutritional profiles. 8
Commonly, UPFs represent a category of food products that undergo extensive industrial processing, often containing multiple ingredients, additives, and preservatives to make them not only convenient (ready to eat) but also palatable and appealing. This approach has been used to create the most widely used UPF classification, the NOVA Food Classification system. 9 , 10 Ultraprocessed foods are typically rich in saturated fats, sugars, sodium, and other substances (eg, additives) and lower in essential nutrients, all of which are associated with cardiometabolic health. 11 , 12 Due to their high availability and affordability and wide marketing to children, UPFs have become increasingly frequent in modern diets, particularly among children, adolescents, 13 - 15 and their families, and especially among individuals and families with low socioeconomic status and educational levels in which obesity is more prevalent. 16 Additionally, the habits established during early childhood often track to later ages 17 and carry into adulthood, compounding the risk of CVD and other noncommunicable diseases. 18 - 20
Previous observational studies in adults have reported positive associations between UPF consumption and obesity, 21 type 2 diabetes, 22 CVD, 23 and all-cause mortality 24 ; however, the epidemiologic evidence in children remains limited and controversial. 25 While the majority of studies have reported unfavorable associations with body mass index (BMI), others did not find this association, and few have focused on cardiometabolic risk factors. 25
Given the public health burden of CVDs and the increasing availability of UPFs, having a better understanding of potential associations between UPF consumption and cardiometabolic risk factors in children is essential. Therefore, the aim of this study was to examine the associations between UPF consumption and cardiometabolic risk factors in a population of Spanish preschool children (aged 3-6 years).
This cross-sectional study was conducted using data from the Childhood Obesity Risk Assessment Longitudinal Study (CORALS), which followed the Strengthening the Reporting of Observational Studies in Epidemiology ( STROBE ) reporting guideline. The ethics committee of each of recruitment center approved the study protocol, which was conducted following the standards of the Declaration of Helsinki. 26 Parents or caregivers provided written informed consent.
CORALS is an ongoing prospective multicenter study conducted in 7 Spanish centers aiming to identify potential risk factors for childhood obesity over a 10-year follow-up period. A detailed description of the CORALS is published elsewhere. 27 Between March 22, 2019, and June 30, 2022, eligible participants aged 3 to 6 years at enrollment were recruited from schools across 7 cities in Spain. To be enrolled in the study, parents or caregivers had to sign a consent form, attend the inclusion face-to-face visit and complete several questionnaires at home for data collection on leisure time physical activity, 3-day food consumption, and sociodemographics. The exclusion criteria included belonging to a family with difficulty collaborating due to low command of Spanish or unstable residence.
To estimate the dietary intake of UPFs, trained dietitians (B.P.-V., S.d.L.H.D., M.L.M.-B., K.A.P.-V., and R.V.-C.) used the validated, semiquantitative, 125-item food and beverage frequency COME-Kids questionnaire. 28 Participants with energy intake below the first percentile or above the 99th percentile were excluded to minimize misreporting (details are provided in the eAppendix in Supplement 1 ). The NOVA Food Classification system was used to determine the consumption of food depending on its degree of processing 27 (details are provided in the eAppendix and eTable 1 in Supplement 1 ).
Adiposity measurements and cardiometabolic risk factor assessments were conducted in health care centers. Weight and body fat mass were measured using a precision scale and an octopolar multifrequency bioelectrical impedance device (MC780MAS; Tanita). Height was measured using a portable seca 213 stadiometer according to standard procedures. Body mass index was calculated and categorized as underweight or normal weight or as overweight or obesity based on pediatric cutoffs. 29 Waist circumference was determined using a flexible, nonextensible measuring tape. The fat mass index was estimated by dividing body fat mass in kilograms by height in meters squared. 30 Waist-to-height ratio was estimated by dividing waist circumference in centimeters by height in centimeters. 31
Blood pressure was measured in the nondominant arm 3 times, with a 5-minute gap between each measurement, using an automatic oscillometer (M3 Intelligence HEM-75051-EV; OMRON Healthcare) equipped with a child-sized cuff. Eight-hour fasting blood samples were collected from participants, and serum total cholesterol, high-density lipoprotein (HDL) cholesterol, low-density lipoprotein (LDL) cholesterol, triglycerides, plasma glucose, and insulin concentrations were measured using standard procedures. Homeostasis model assessment for insulin resistance (HOMA-IR) was calculated as fasting insulin (μIU/mL) × fasting glucose (mmol/L) / 22.5.
Parents or caregivers were provided with a set of questionnaires to complete at home, gathering information on early life factors, maternal characteristics, and lifestyle patterns. To assess physical activity, the total time (in hours) of engagement in sports and physical activities per week was estimated using a previously validated questionnaire. 32 An 18-item questionnaire for children was used to assess adherence to the Mediterranean diet, an indicator of diet quality. 27
The current analysis was conducted using the CORALS database updated through January 20, 2023. Analysis of descriptive baseline characteristics are reported as mean (SD) or median (IQR) for continuous variables and as numbers with percentages for categorical variables using one-way analysis of variance and χ 2 test, respectively.
Consumption of UPFs (in grams per day) was adjusted for total energy intake using the residual regression method. 33 Intake of UPFs in grams per day was calculated instead of energy percentage to account for foods with no energy content (eg, artificially sweetened beverages) and for nonnutritional concerns associated with food processing (eg, food additives). Participants were categorized by tertiles of energy-adjusted UPF consumption, ranging from tertile 1 for the lowest intake to tertile 3 for the highest intake.
Age- and sex-specific z scores of each outcome were estimated from standardized residuals conducted using linear regression models. Missing data of less than 5% for covariates were imputed to the mean and to the highest frequency category for quantitative and qualitative confounders, respectively. 34 Multivariable linear regression models were fitted to assess the associations (β coefficient and 95% CI) between tertiles of energy-adjusted UPFs and z scores of cardiometabolic risk factors. The first tertile (lowest intake) was considered the reference. Models were adjusted for maternal education level (primary or lower, secondary or university), maternal BMI (underweight, normal overweight, obesity), physical activity (minutes per week), exclusive breastfeeding (yes or no), recruitment center size (<200, 200-400, >400 participants), and NOVA group 1, 2, or 3 (as detailed in eTable 1 in Supplement 1 ). To assess the linear trend, the median value of each tertile of UPF consumption was modeled as a continuous variable. The analysis was also conducted in a continuous form, with a 1-SD increment and using the same confounders.
Additionally, a simulation model was fitted to substitute 100 g of consumed UPFs with 100 g of unprocessed or minimally processed food to examine the association of healthier food consumption with the outcomes. The theoretical impact of substituting 1 food group for another was assessed by introducing both variables simultaneously as continuous variables into the model. Differences in the β coefficients, variances, and covariance were used to estimate the β coefficients and 95% CIs for the substitution association. Sensitivity analyses were conducted to assess associations according diet quality, maternal education, and socioprofessional level (details provided in the eAppendix in Supplement 1 ).
Data were analyzed using Stata, version 14 software (StataCorp LLC). All statistical tests were 2-sided, and P < .05 was deemed statistically significant.
A total of 1426 participants (mean [SD] age, of 5.8 [1.1] years; 698 boys [49.0%] and 728 girls [51.0%]) were included in this study after excluding 54 participants lacking the food and beverage frequency questionnaire and 29 with missing data or implausible reported energy intake (eFigure 1 in Supplement 1 ). The characteristics of the study population across tertiles of energy-adjusted UPF consumption are shown in Table 1 . Children in the third tertile (highest UPF consumption) had a higher BMI z score, waist-to-height ratio, fat mass index, systolic blood pressure, and overweight or obesity prevalence and lower HDL and LDL cholesterol. Mothers whose children were categorized in the highest tertile of energy-adjusted UPF consumption were younger, had a higher BMI, were more prone to be living with overweight or obesity, were less likely to have exclusively breastfed their children, and had lower educational achievement and employment rates.
General dietary characteristics of participants are shown in Table 2 . Children in the top tertile were more likely to consume higher amounts of total energy, carbohydrates, yogurt, other dairy products, sugar and candy, and sugary beverages and lower amounts of protein, fat, monounsaturated and polyunsaturated fatty acids, fiber, milk, cheese, white meat, unprocessed red meat, eggs, fish, seafood, vegetables, fruits, nuts, legumes, whole and refined cereals, and oils and fat.
Cross-sectional associations between energy-adjusted UPF consumption across tertiles and by 1-SD increment (in grams per day) and cardiometabolic risk factors are shown in Table 3 . Compared with participants in the lowest tertile, those in the top tertile had higher z scores of waist circumference (β coefficient, 0.20; 95% CI, 0.05-0.35), BMI (β coefficient, 0.20; 95% CI, 0.05-0.35), fat mass index (β coefficient, 0.17; 95% CI, 0.00-0.32), and fasting plasma glucose (β coefficient, 0.22; 95% CI, 0.06-0.37). Additionally, participants in the highest tertile had a lower z score of HDL cholesterol (β coefficient, −0.19; 95% CI, −0.36 to −0.02). After adjusting for the Mediterranean diet score (12 points), the associations were maintained for the z scores of fasting plasma glucose (β coefficient, 0.17; 95% CI, 0.03-0.31) and HDL cholesterol (β coefficient, −0.20; 95% CI, −0.36 to −0.05) (eTable 2 in Supplement 1 ). Positive associations were also observed between 1-SD increments of UPF consumption and z scores of waist circumference (β coefficient, 0.09; 95% CI, 0.02-0.15), fat mass index (β coefficient, 0.11; 95% CI, 0.04-1.18), BMI (β coefficient, 0.11; 95% CI, 0.05-0.17), and fasting plasma glucose (β coefficient, 0.10; 95% CI, 0.03-0.17) and negatively associated with HDL cholesterol (β coefficient, −0.07; 95% CI, −0.15 to 0.00) ( Table 3 ). Likewise, after further adjusting for the Mediterranean diet score, the associations between 1-SD increments remained significant for the z scores of fasting plasma glucose (β coefficient, 0.08; 95% CI, 0.02-0.13) and HDL cholesterol (β coefficient, −0.07; 95% CI, −0.13 to −0.01) (eTable 2 in Supplement 1 ).
Similar positive associations among fat mass index, BMI, and plasma glucose were observed, irrespective of the animal or vegetable origin of the UPFs consumed. Substitution of 100 g of UPFs with 100 g of unprocessed or minimally processed foods was associated with a decrease in z scores of fat mass index (β coefficient, −0.03; 95% CI, −0.06 to 0.00) and BMI (β coefficient, −0.03; 95% CI, −0.06 to −0.01), and fasting plasma glucose (β coefficient, −0.04; 95% CI, −0.07 to −0.01) ( Figure ). The same models adjusted for the Mediterranean diet score showed positive associations for z scores of fasting plasma glucose (β coefficient, −0.04; 95% CI, −0.06 to −0.01) and inverse association for z score of HDL cholesterol (β coefficient, 0.03; 95% CI, 0.00-0.07) (eFigure 2 in Supplement 1 ). No associations were shown for the other outcomes.
In children whose mothers were unemployed, a positive association was found between energy-adjusted UPF consumption and z scores of waist circumference (β coefficient, 0.26; 95% CI, 0.14-0.39), fat mass index (β coefficient, 0.20; 95% CI, 0.07-0.34), waist-to-height ratio (β coefficient, 0.21; 95% CI, 0.09-0.34), BMI (β coefficient, 0.18; 95% CI, 0.04-0.31), fasting plasma glucose (β coefficient, 0.14; 95% CI, 0.03-0.25), and diastolic blood pressure (β coefficient, 0.14; 95% CI, 0.0-0.27). In children with employed mothers, a positive association was observed between 1-SD increments in energy-adjusted UPF consumption and z scores of fasting plasma glucose (β coefficient, 0.09; 95% CI, 0.01-0.17) and a negative association in case of the HDL cholesterol (β coefficient, −0.09; 95% CI, −0.18 to −0.01) (eTable 3 in Supplement 1 ).
Children whose mothers had a low education level had higher z scores of waist circumference (β coefficient, 0.14; 95% CI, 0.05-0.23), fat mass index (β coefficient, 0.15; 95% CI, 0.06-0.25), BMI (β coefficient, 0.15; 95% CI, 0.06-0.24), and fasting plasma glucose (β coefficient, 0.11; 95% CI, 0.02-0.19). Children whose mothers had a high education level had a lower HDL cholesterol z score (β coefficient, −0.15; 95% CI, −0.26 to −0.03) (eTable 4 in Supplement 1 ).
To our knowledge, this study is the first to assess the associations between UPF consumption and various cardiometabolic risk factors in young children. In this large cross-sectional study, UPF consumption was positively associated with z scores of BMI, waist circumference, fat mass index, and fasting plasma glucose concentration and inversely associated with HDL cholesterol concentration.
Our findings are in line with those of previous studies. 35 - 37 Consumption of UPFs at age 4 years was associated with increased BMI z scores at age 10 years in the Generation XXI cohort, while no association was found at age 7 years. 35 Another study showed that high UPF consumption in children aged 7 to 13 years was associated with increased BMI growth trajectories. 36 Similarly, lower UPF intake in Spanish children aged 4 to 7 years was associated with lower BMI z scores at age 7 years, though this association became nonsignificant after adjusting for maternal factors. 37
A global study by Neri et al 38 revealed that increased UPF consumption was associated with higher dietary energy density and intake of free sugars, alongside decreased total fiber intake, potentially contributing to childhood obesity. Additionally, findings from the Avon Longitudinal Study of Parents and Children showed that high UPF consumption was associated with unfavorable fat mass index trajectories from age 7 to 24 years. 36 Similarly, in a Brazilian cohort, UPF consumption during preschool years was associated with increases in waist circumference from preschool to school age. 39 Other studies found no significant association between UPF consumption and HDL cholesterol and fasting plasma glucose concentrations. 37 , 40 Therefore, to our knowledge, our study is the first in children to find significant associations with the aforementioned risk factors and is in line with other studies assessing adult populations. 41
Our results provide new insight into the association between UPF consumption and health and the importance of recognizing that early dietary habits in childhood might have future implications on cardiometabolic health. While the magnitude of the associations reported in our study may be considered of limited clinical relevance, it is important to note that our study consisted of young children. Therefore, if such minimal differences can reveal a significant association, they may serve as an early warning of future cardiometabolic conditions.
Our results are in line with previous studies showing that the main UPF products consumed are pastries, sweet beverages, cookies, and candies. 42 - 44 In addition, our results support the findings of other European studies that have shown that children of mothers with lower education or with lower socioeconomic status are more likely to consume UPFs. These findings suggest that educational and socioeconomic factors may contribute to the purchase of low-cost and unhealthy foods, such as UPFs, increasing the risk of health disorders. 37 , 45 , 46
Several possible mechanisms could explain our results. First, UPFs contain higher amounts of sodium, energy, fat, and sugar and lower amounts of fiber, which are well recognized as contributors to cardiometabolic risk factors. 47 In addition, some UPFs may be linked to a higher glycemic response, and it has been shown that high consumption of sugar-sweetened beverages may delay the internal satiety signal, leading to excessive calorie intake and higher glycemic load. 48 - 50 Moreover, excessive consumption of energy, saturated fat, and sugar contributes to weight gain and a higher risk of obesity, which is an important risk factor in CVD. 51 Furthermore, our study showed that children who consumed high amounts of UPFs tended to have lower intakes of fruits and vegetables, which, along with a healthy dietary pattern, are known to be beneficial for cardiometabolic health. 52
Most of the associations were maintained in our study after further adjusting the models to Mediterranean diet adherence, suggesting that other intrinsic UPF factors may play an important role in determining these associations (eg, additives). Animal and cellular studies revealed potential cardiovascular risks from authorized additives such as sulfites, monosodium glutamate, and emulsifiers. 53 - 58 Food processing generates contaminants such as acrylamide and acrolein, which have been linked, respectively, to increased odds and risk of cardiovascular disease. 59 , 60 Ultraprocessed foods may contain chemicals such as bisphenols and perfluoroalkyl substances that have been associated with a higher risk of cardiometabolic outcomes in children. 61 , 62
The NOVA Food Classification system has sparked debate among researchers due to disagreements over UPF definitions, bias concerns, and the system’s contribution to dietary guidelines. 63 , 64 The NOVA system itself has some limitations, as it does not consider that certain minimal processing could improve the final product (eg, fermentation in milk) and adopts a vague definition of what is considered a cosmetic additive, which has led to considering carotenoids as an additive that increases the potential harmfulness of a product. 65 Despite these limitations, NOVA categories have consistently shown associations with cardiometabolic health in adults.
This study has several strengths. Most importantly, the study was conducted in a large sample size from 7 different geographic areas of Spain. The study also assessed cardiometabolic risk factors not considered in other similar studies. 21 - 24
Our study also has several limitations. First, because the study is observational, we cannot draw conclusions on cause and effect. Second, our study involved preschool children from Spain, which means that the generalization of our findings to different populations is not appropriate. Third, some grade of misclassification could be present in our study since UPF consumption was estimated from a food and beverage frequency questionnaire that was not specifically developed to assess this type of food, which could result in either an overestimation or underestimation of consumption within various NOVA categories. Additionally, imprecise estimations could also arise from the use of a food and beverage frequency questionnaire, which may be influenced by social desirability bias. Finally, we cannot dismiss that associations may be due to residual confounding or that undetected cardiometabolic disorders in our study population may exist due to age.
In this large cross-sectional study, UPFs consumption was positively associated with fasting plasma glucose levels, BMI, waist circumference, and fat mass index and inversely associated with HDL cholesterol concentration. These findings highlight the importance of promoting unprocessed or minimally processed foods and reducing UPF consumption, particularly starting from early ages. However, further prospective studies are warranted to validate our findings.
Accepted for Publication: March 16, 2024.
Published: May 17, 2024. doi:10.1001/jamanetworkopen.2024.11852
Open Access: This is an open access article distributed under the terms of the CC-BY License . © 2024 Khoury N et al. JAMA Network Open .
Corresponding Author: Nancy Babio, PhD, Unitat de Nutrició Humana, Departament de Bioquímica i Biotecnología, Universitat Rovira i Virgili, c/Sant Llorenç 21, 43201 Reus, Spain ( [email protected] ).
Author Contributions: Ms Khoury and Dr Babio had full access to all of the data in the study and take responsibility for the integrity of the data and the accuracy of the data analysis. Ms Khoury and Dr M. Á. Martínez contributed equally to this work as co–first authors. Prof Salas-Salvadó and Dr Babio contributed equally to this work as co–senior authors.
Concept and design: Khoury, M. Á. Martínez, Miguel-Berges, Navas-Carretero, Portoles, Leis, J. F. Martínez, Flores-Rojas, Moreno Aznar, Gil-Campos, Salas-Salvadó, Babio.
Acquisition, analysis, or interpretation of data: Khoury, M. Á. Martínez, Garcidueñas-Fimbres, Pastor-Villaescusa, Leis, Pérez-Vega, Jurado-Castro, Vázquez-Cobela, Andía Horno, J. A. Martínez, Picáns-Leis, Luque, Moreno Aznar, Castro-Collado, Gil-Campos, Salas-Salvadó, Babio.
Drafting of the manuscript: Khoury, Leis, de las Heras Delgado, Vázquez-Cobela, Andía Horno, J. A. Martínez, Flores-Rojas, Salas-Salvadó, Babio.
Critical review of the manuscript for important intellectual content: Khoury, M. Á. Martínez, Garcidueñas-Fimbres, Pastor-Villaescusa, Leis, Miguel-Berges, Navas-Carretero, Portoles, Pérez-Vega, Jurado-Castro, Vázquez-Cobela, J. A. Martínez, Picáns-Leis, Luque, Moreno Aznar, Castro-Collado, Gil-Campos, Salas-Salvadó, Babio.
Statistical analysis: Khoury, Garcidueñas-Fimbres, de las Heras Delgado, Vázquez-Cobela.
Obtained funding: Khoury, Navas-Carretero, J. A. Martínez, Flores-Rojas, Moreno Aznar, Gil-Campos, Salas-Salvadó, Babio.
Administrative, technical, or material support: Leis, Pérez-Vega, Vázquez-Cobela, Andía Horno, J. A. Martínez, Picáns-Leis, Luque, Gil-Campos, Salas-Salvadó, Babio.
Supervision: M. Á. Martínez, Leis, Portoles, Vázquez-Cobela, J. A. Martínez, Picáns-Leis, Luque, Moreno Aznar, Gil-Campos, Babio.
Conflict of Interest Disclosures: Prof Salas-Salvadó reported being a nonpaid member of the scientific boards of the International Nut and Dried Fruit Foundation, Danone Institute International, and Fundación Eroski; receiving institutional grants from the International Nut and Dried Fruit Foundation; and receiving personal fees from Danone Institute Spain. No other disclosures were reported.
Funding/Support: The establishment of the Childhood Obesity Risk Assessment Longitudinal Study (CORALS) cohort in the first year of the study (2019) was supported by an agreement between Consorcio Centro de Investigación Biomédica en Red, M. P. Fisiopatología de la Obesidad y Nutrición and Danone Institute Spain. This study was supported by grants 2021FI B 00145 from the Agència de Gestió d’Ajuts Universitaris de Recerca (Ms Khoury) and CD21/00045 from Sara Borrell (Dr M. Á. Martínez).
Role of the Funder/Sponsor: The funders had no role in the design and conduct of the study; collection, management, analysis, and interpretation of the data; preparation, review, or approval of the manuscript; and decision to submit the manuscript for publication.
Data Sharing Statement: See Supplement 2 .
Additional Contributions: The authors thank all the CORALS participants and their parents or caregivers as well as the health centers and primary schools for their collaboration, the CORALS personnel for outstanding support, and the staff of all associated primary care centers for exceptional work. The authors also acknowledge the Institut d’Investigació Sanitària Pere Virgili Biobank (PT20/00197), which is integrated in the Instituto de Salud Carlos III Platform for Biobanks and Biomodels.
- Register for email alerts with links to free full-text articles
- Access PDFs of free articles
- Manage your interests
- Save searches and receive search alerts
- Open access
- Published: 23 May 2024
Correlation between triglyceride glucose-body mass index and hypertension risk: evidence from a cross-sectional study with 60,283 adults in eastern China
- Yijia Chen 1 na1 ,
- Jinling Du 2 na1 ,
- Nan Zhou 1 , 3 ,
- Yingqian Song 4 ,
- Weiwei Wang 1 &
- Xin Hong 1 , 3
BMC Cardiovascular Disorders volume 24 , Article number: 270 ( 2024 ) Cite this article
20 Accesses
Metrics details
Insulin resistance (IR) and obesity are established risk factors for hypertension, with triglyceride-glucose (TyG) serving as a recognized surrogate marker for IR. The aim of this study was to investigate the association between TyG-BMI and hypertension in the general population.
A total of 60,283 adults aged ≥18 years who underwent face-to-face questionnaires, anthropometric measurements, and laboratory examination were included in this study. Multivariable logistic regression models and receiver operating characteristic curve (ROC) were used to determine the association between TyG-BMI and hypertension. The restricted cubic spline model was used for the dose-response analysis.
After fully adjusting for confounding variables, multivariate logistic regression model showed a stable positive association between TyG-BMI and hypertension (OR: 1.61 per SD increase; 95% CI: 1.55–1.67; P-trend < 0.001). The multivariate adjusted OR and 95% CI for the highest TyG-BMI quartile compared with the lowest quartile were 2.52 (95% CI 2.28–2.78). Dose-response analysis using restricted cubic spline confirmed that the association between TyG-BMI index and hypertension was linear. Subgroup analyses showed that stronger associations between TyG-BMI index and hypertension were detected in young and middle-aged individuals (P for interaction < 0.05). ROC analysis showed that TyG-BMI index could better predict the risk of hypertension than other parameters (TyG-BMI cut-off value: 207.105, AUC: 0.719, sensitivity 65.5%, specificity 66.8%), particularly among young and middle-aged people.
The TyG-BMI index was independently associated with hypertension in the study population. Further studies are required to confirm this relationship.
Peer Review reports
Introduction
Hypertension, a major risk factor for cardiovascular disease (CVD) and premature death, has been recognized as a worldwide public health challenge [ 1 , 2 ]. A recent national survey found that the crude prevalence of hypertension among Chinese residents aged ≥ 18 years was 27.9% (weighted rate of 23.2%) [ 3 ]. The direct economic burden due to hypertension accounted for 6.6% of total health costs in China, imposing a significant burden of disease on the country [ 4 ]. Given the large number of people suffering from hypertension and the heavy burden of the disease, it would be meaningful to identify at-risk individuals prone to hypertension at an early stage through a simple but effective diagnostic tool.
Insulin resistance (IR) is a common pathological state in which insulin-dependent cells, such as adipocytes and cardiomyocytes, are impaired in their ability to respond to insulin [ 5 ]. Several studies have demonstrated that IR is the key mechanism in many metabolic diseases, such as diabetes [ 6 ], obesity [ 7 ] and metabolic syndrome [ 8 ]. In addition, a meta-analysis involving 11 studies showed that IR is independently associated with hypertension and plays a crucial role in the development of hypertension [ 9 ]. Several studies [ 10 , 11 ] have shown that IR causes elevated blood pressure by causing renal sodium retention, activating the sympathetic nervous system, increasing peripheral and renal vascular resistance, and damaging the vascular endothelium. Therefore, early and accurate recognition of IR is clinically important for the implementation of preventive and management measures for hypertension. The hyperinsulinemic-euglycemic clamp (HEC) technique is currently the gold standard for the diagnosis of insulin resistance [ 12 ]. However, this method of assessing IR is costly, complex, time-consuming and ethically restricted.
In recent years, the triglyceride glucose (TyG) index, calculated from the combination of fasting glucose (FPG) and triglycerides (TG), has been widely used as a simple and effective surrogate marker for the early identification of IR [ 13 , 14 , 15 , 16 ]. Currently, it has been shown that the product of TyG index combined with body mass index (BMI) has a stronger diagnostic value in identifying IR [ 17 , 18 ]. Previous studies have highlighted the robust predictive capability of TyG-BMI for major chronic diseases with substantial burden, such as diabetes [ 19 ], and cardiovascular diseases, primarily including ischemic stroke, heart failure, and hypertension [ 20 , 21 , 22 , 23 , 24 , 25 ]. Furthermore, it has shown a significant ability to predict mortality risk [ 26 ]. A cross-sectional study [ 23 ] involving 2,124 subjects aged 18 years and older in Romania showed an association between TyG-BMI index and hypertension (OR: 2.12, 95% CI: 1.62–2.78). Another study [ 24 ] involving 4,352 Chinese people aged ≥ 65 years also showed that TyG-BMI index was significantly associated with hypertension (OR: 3.56, 95% CI: 2.70–4.70). However, the sample sizes of these studies were relatively small and their study subjects were not representative of the eastern Chinese population aged ≥ 18 years.
Therefore, this study aimed to investigate the association and dose-response relationship between TyG-BMI and hypertension among people aged ≥ 18 years in eastern China.
Study population and sampling
Data were obtained from the Chronic Disease and Risk Factor Surveillance in Nanjing, the capital of Jiangsu Province in eastern China. This survey was a population-based cross-sectional study designed to determine the prevalence of chronic diseases and associated risk factors from January 2017 to June 2018. Detailed information on the study design, sampling methods, participant characteristics have been previously published [ 27 ]. Briefly, a representative sample of permanent residents aged ≥ 18 years that had lived in the local village/community for at least 6 months was obtained in this study using a multi-stage stratified random cluster sampling method. A total of 62,000 study subjects were recruited and 61,098 subjects agreed to participate in the survey, with a response rate of 98.5%. Eight hundred and fifteen study subjects were excluded due to missing data from face-to-face questionnaires, anthropometric measurements, and blood samples, 60,283 participants were included in the final analyses.
Data collection and measurement
Data from face-to-face questionnaires, anthropometric measurements, and blood samples were collected by trained medical professionals. The questionnaire included basic demographic characteristics (e.g., age, gender), behavioral risk factors (e.g., smoking and drinking status), personal and family medical history of chronic diseases (e.g., diabetes, hypertension, dyslipidemia). Anthropometric measurements included height, body weight, waist circumference (WC), and blood pressure (BP). All participants were asked to rest for at least 5 min before BP measurements. BP was measured three times in a seated position by a trained staff using an automatic sphygmomanometer (Omron HBP-1300, Japan). Three measurements were taken at 2-min intervals, and the average of the last two measurements was taken as the final blood pressure value. Blood samples were drawn after a fasting overnight of at least 10 h. Fasting plasma glucose (FPG) was assessed with a glucose oxidase method; total cholesterol (TC), triglycerides (TG), high-density lipoprotein cholesterol (HDL-C), and low-density lipoprotein cholesterol (LDL-C) were analyzed enzymatically with commercially available reagents. The method and study design have been described previously [ 27 ].
Definition of TyG-BMI index
TyG-BMI index = TyG index × BMI, where BMI = weight (kg) / height (m) 2 , and the TyG index = Ln [FPG (mg/dL) × TG (mg/dL) /2] [ 28 ].
Diagnosis of hypertension
According to Chinese guidelines on the prevention and treatment of hypertension in adults (2018 Revised Edition) [ 29 ], hypertension was defined as self-reported current treatment with antihypertensive medication in the past two weeks, and/or an average systolic blood pressure (SBP) ≥ 140 mmHg and/or an average diastolic blood pressure (DBP) ≥ 90 mmHg.
According to Chinese guidelines on the prevention and treatment of dyslipidemia in adults (2016 Revised Edition) [ 30 ], abnormal TC was defined as TC ≥ 6.2mmol/L, abnormal TG was defined as TG ≥ 2.3mmol/L, abnormal LDL-C was defined as LDL-C ≥ 4.1mmol/L, abnormal HDL-C was defined as HDL-C < 1.0mmol/L. Dyslipidemia was defined as self-reported history of dyslipidemia and/or the use of antilipemic medication, and/or having at least one of the above abnormal serum lipids. According to Chinese guidelines on the prevention and treatment of type 2 diabetes in adults (2017) [ 31 ], Diabetes was defined as self-reported current treatment with anti-diabetes medication (insulin or oral hypoglycemic agents), and/or FPG ≥ 7.0 mmol/L. Regular exercise was defined as exercising 2 or more days per week [ 32 , 33 ]. Current smoking was defined as participants who have smoked at least 100 cigarettes in their lifetime and currently smoke cigarettes [ 34 ]. Current drinking was defined as consuming at least 1 alcoholic beverage per week in the past month [ 35 ]. Overweight and obesity were defined as BMI between 24.0 and 27.9 kg/m 2 and of ≥ 28.0 kg/m 2 , respectively [ 36 ]. Central obesity was defined as a WC ≥ 90 cm in men and ≥ 85 cm in women [ 37 ].
Statistical analysis
Standardized values (means, prevalence) were calculated using the weight coefficients to represent the total Nanjing adult population aged ≥ 18 years. Weight coefficients accommodated the sampling scheme for unequal probabilities of sample selection, as well as the post-stratification weights, which harmonized the standard population of the 2009 Nanjing Sixth National Population Census by two genders and 12 age groups (5-year intervals) [ 38 ]. Quantitative data were presented as means±SD, and qualitative data as proportions. Differences in quantitative and qualitative variables were compared by One-way ANOVA and Chi-square test, respectively.
Multivariate logistic regression model was used to analyze the association between TyG-BMI index and hypertension. Before building the multiple regression models, the collinearity between the variables was checked and the variance inflation factor (VIF) was calculated for each variable. Variables with VIF > 5 were considered as collinear variables and could not be included in the multiple regression model [ 39 ]. The results showed that height, weight, BMI, and TG were not included in the model (Supplementary Table 1 ). As a categorical variable classified by quartile, or a continuous variable using the standard deviation transformed, the level of TyG-BMI index was included in the regression model analysis separately. Four models were used, with the crude model being unadjusted. Model 1 adjusted for sex and age; model 2 adjusted for model 1 plus area, education, current smoking, current drinking, regular exercise, family history of hypertension; model 3 adjusted for age, sex, area, education, current smoking, current drinking, regular exercise, family history of hypertension, WC, TG, LDL-C, HDL-C, FPG. In addition, stratified analysis and interaction test were used to explore whether the correlation between TyG-BMI and hypertension differed between subgroups.
Meanwhile, we calculated the area under the receiver-operating characteristics (ROC) curves (AUC) and other parameters to examine the relationship between TyG-BMI and hypertension. Additionally, we used restricted cubic splines model to analyze the dose-response relationship between TyG-BMI and hypertension [ 40 ].
To validate the stability of the results, we conducted sensitivity analysis. Firstly, the participants were classified into three groups based on blood pressure values: ideal blood pressure, pre-hypertension, and hypertension. Using those with ideal blood pressure as the reference group, we analyzed the relationship between TyG-BMI index and pre-hypertension as well as hypertension. Additionally, a multiple linear regression model was employed to analyze the relationship between TyG-BMI index with SBP and DBP.
All statistical analyses were conducted by the R software 3.6.3 ( https://www.R-project.org , The R Foundation) or SPSS software (version 20; IBM, Armonk, NY, USA). All P -values were two-tailed with a significant level of < 0.05.
Baseline characteristics
A total of 60,283 participants (29,848 male and 30,435 female) aged 18 years and above were included in our study. The mean age was 46.86 ± 17.30 years (male mean age was 46.67 ± 17.21 years and female mean age was 47.04 ± 17.38 years). The average TyG-BMI level was 204.86 ± 35.28. There were 15,686 hypertension patients with a weighted prevalence rate of 29.8%. (Supplemental Table 2 ). The weighted prevalence rate of hypertension is higher among males, the elderly, residents in rural areas, individuals with a primary education or below, current smokers, current drinkers, participants with no regular exercise, and individuals who are overweight or obese, as well as those who have comorbidities of diabetes and dyslipidemia. (All P < 0.05) (Supplemental Table 3 ).
Baseline characteristics according to the TyG-BMI levels were shown in Table 1 . Age, Height, Weight, BMI, WC, SBP, DBP, FPG, TC, TG, and LDL-C levels increased with increasing levels of TyG-BMI, while the level of HDL-C decreased with increasing levels of TyG-BMI (All P < 0.001). Moreover, participants with higher levels of TyG-BMI had a significantly higher prevalence of obesity/overweight, central obesity, diabetes, dyslipidemia and hypertension (All P < 0.05). Subjects with higher TyG-BMI levels had a significantly higher proportion of current smoking, current drinking and family history of hypertension (All P < 0.05).
Association between TyG-BMI and hypertension
The association between TyG-BMI and hypertension was estimated by a multivariate logistic regression model (Table 2 ). When the standard deviation-transformed TyG-BMI was analyzed as a continuous variable, per SD increase in TyG-BMI led to higher odds of hypertension in the univariate logistic regression model (OR: 2.24, 95% CI: 2.19–2.28). The association remained statistically significant in all multivariate logistic regression models after adjusting for several covariates including age, sex, area, education, current smoking, current drinking, regular exercise, family history of hypertension, WC, TG, LDL-C, HDL-C, FPG. (Model 1: OR: 1.89, 95% CI: 1.85–1.94; Model 2: OR: 1.82, 95% CI: 1.77–1.87; Model 3: OR:1.61, 95% CI: 1.55–1.67). When the TyG-BMI index was treated as a categorical variable based on quartiles, compared with participants in the lowest quartile, those in the highest quartile of the TyG-BMI index had a higher association with hypertension in all four models (unadjusted model: OR: 8.85, 95% CI: 8.30–9.43; Model 1: OR: 4.67, 95% CI: 4.35–5.02; Model 2: OR:4.16, 95% CI: 3.84–4.51; Model 3: OR: 2.52, 95% CI: 2.28–2.78; all P for trend < 0.001). The dose-response analysis with a restricted cubic spline model showed a linear relationship between the TyG-BMI index and hypertension (P for non-linearity test = 0.006) (Fig. 1 ). The results of the sensitivity analysis revealed that, when using individuals with ideal blood pressure as the reference group, the multivariate adjusted OR and 95% CI for the highest quartile of TyG-BMI compared to the lowest quartile were 1.88 (95% CI 2.72–2.06) for pre-hypertension and 4.11 (95% CI 3.59–4.70) for hypertension (Supplemental Fig. 1 ). Additionally, after adjusting for Model 3, a positive association was observed between the TyG-BMI index with SBP and DBP (All P < 0.05) (Supplemental Table 4 ). In addition, an ROC analysis was conducted to evaluate the value of TyG-BMI in relation to hypertension. Table 3 presented the AUC and optimal thresholds of TyG-BMI and other parameters in association with hypertension. In this dataset, TyG-BMI demonstrated the highest AUC (0.719), indicating a moderate level of performance in relation to hypertension.
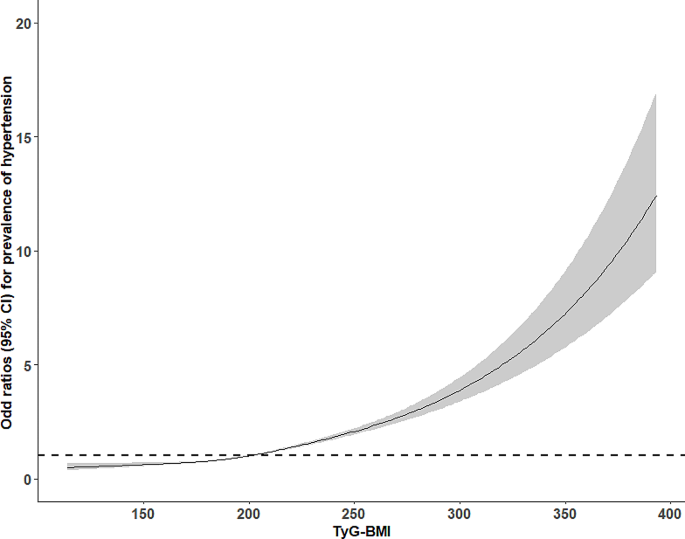
Dose-response relationship between TyG-BMI and hypertension by the restricted cubic spline. Adjusted for age, sex, area, education, current smoking, current drinking, regular exercise, family history of hypertension, WC, TG, LDL-C, HDL-C, FPG
Subgroup analysis
Stratified analysis assessed the the effect of TyG-BMI (per 1 SD increase) on hypertension in different subgroups (Fig. 2 ). The associations between TyG-BMI and hypertension were positive in the following subgroups: gender (male vs. female; P-interaction = 0.705), current smoking (no vs. yes; P-interaction = 0.437), current drinking (no vs. yes; P-interaction = 0.136), regular exercise (no vs. yes; P-interaction = 0.497), family history of hypertension (no vs. yes; P-interaction = 0.280), and BMI (< 24 kg/m 2 vs. 24-<28 kg/m 2 vs. ≥28 kg/m 2 ; P-interaction = 0.741).
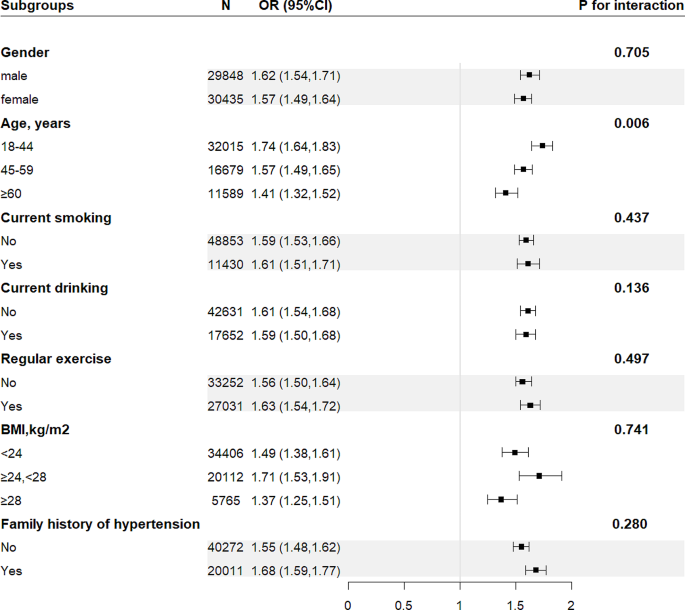
Subgroup analyses of the effect of TyG-BMI index on the prevalence of hypertension. Each subgroup analysis adjusted for age, sex, area, education, current smoking, current drinking, regular exercise, family history of hypertension, WC, TG, LDL-C, HDL-C, FPG, appropriately. Abbreviations as in Table 1
Moreover, there was a significant interaction between TyG-BMI and age on hypertension (P-interaction < 0.05). In the age stratification, the correlation between TyG-BMI and hypertension was significantly higher in young and middle-aged people than in older people. Considering the significant differences in age stratification in the subgroup analysis. ROC analysis was conducted to further evaluate the association between TyG-BMI and hypertension across different age groups. The results showed that TyG-BMI exhibited a higher AUC value middle-aged and younger individuals with hypertension (Fig. 3 , Supplementary Table 5 ).
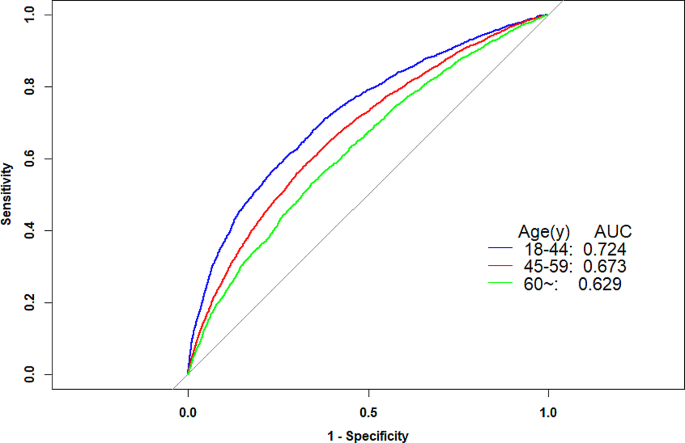
Receiver operating characteristic curve analyses of TyG-BMI by age. All P < 0.05, compare with the group of ≥ 60 years
In this study, we analyzed the association between TyG-BMI index and hypertension using a large and representative sample. Our results showed that after adjusting for confounders, there was always a significant positive association with hypertension regardless of whether TyG-BMI index was used as a continuous or a categorical variable, showing a nearly linear dose-response relationship. The results of the stratified analysis showed that the direction of the relationships between TyG-BMI and hypertension among different subgroups were consistent with that of the overall study population. The association between TyG-BMI and hypertension was higher in young and middle-aged people than in elderly people. The ROC analysis demonstrated that the relationship between TyG-BMI and hypertension was stronger compared to TyG and BMI.
TyG-BMI index is being extensively studied as a simple and effective new indicator for the diagnosis of insulin resistance. Two cohort studies examined the ability of TyG-BMI to predict prediabetes as well as diabetes, respectively. Their results indicated that the risk of prediabetes and diabetes increased with increasing TyG-BMI. In addition, the ability of TyG-BMI to predict prediabetes and diabetes was higher than that of TyG and BMI [ 19 , 25 , 41 ]. In our study, the association between TyG-BMI index and hypertension was similar to previous studies. Bala C et al. [ 23 ] explored the correlation between six surrogate of insulin resistance indexes and hypertension, and their results showed that TyG-BMI was independently associated with hypertension after adjusting for confounders. Li YX et al. studied the association of different insulin resistance surrogates with hypertension and hyperuria, and the results showed that the TyG-BMI index had a stronger correlation with hypertension than TyG index [ 24 ]. Moreover, a study [ 42 ] involving 105,070 lean adults in China showed that TyG-BMI was significantly associated with pre-hypertension in both men and women. Consistent with previous research findings [ 16 ], the present study conducted a further analysis on the positive correlation between TyG-BMI and individuals with pre-hypertension These results suggest that TyG-BMI could serve as an early indicator for identifying pre-hypertension cases. Moreover, ROC analysis in our study demonstrated that the AUC of TyG-BMI surpassed that of TyG and BMI, aligning with prior research outcomes [ 23 , 24 ]. These findings underscore the predictive potential of TyG-BMI for hypertension.
The results of our subgroup analysis showed significant differences in the association between TyG-BMI and hypertension by age. In the age stratification, the correlation between TyG-BMI and hypertension was significantly higher in young and middle-aged people than in older people. This phenomenon is thought to be the effect of rapid social development and changes in behavioral lifestyles. On the one hand, young and middle-aged people are under tremendous social pressure due to the increasing aging of the population and the decreasing labor force [ 43 , 44 , 45 ]; on the other hand, with the accelerated development of urbanization and modernization, young people are increasingly exposed to unhealthy lifestyle habits, such as overeating and lack of physical activity, which lead to the premature appearance of various metabolic problems [ 46 , 47 , 48 ]. Previous studies have shown that TyG-BMI index in normal weight individuals was more strongly associated with diabetes [ 19 ], prediabetes [ 41 ], and NAFLD [ 49 ] than overweight and obese individuals. However, this phenomenon did not exist in our study. This can be explained by the inconsistency of the study population and study outcomes. In addition, the diagnosis of obesity in our study was based on BMI. With changes in people’s lifestyle and dietary habits, some subtle changes have taken place in the structure of the human body, especially the significant increase in fat storage [ 50 , 51 ]. Relying on body mass index alone to distinguish obesity does not reflect this information.
Although the underlying mechanisms of the relationship between TyG-BMI and hypertension are unclear, it may be related to insulin resistance. Some reviews have shown that insulin resistance is the mechanism of the development and progression of hypertension [ 52 , 53 ]. Although the stimulatory effect of insulin on glucose uptake by adipocytes is severely diminished by insulin resistance, the effect of insulin on salt reabsorption in the proximal tubules of the kidney is preserved. Thus, insulin-resistant individuals promote renal tubular salt absorption due to compensatory hyperinsulinemia, leading to a state of salt overload and hypertension [ 54 ]. Moreover, insulin resistance mediates the effect of hyperuricemia on the risk of hypertension, and the combined or synergistic role of hyperuricemia and insulin resistance in the development of cardiovascular disease has been reported documented [ 55 ]. Uric acid and IR may affect blood pressure through the following mechanisms: (1) activation of the renin-angiotensin-aldosterone system, (2) direct effects of uric acid and insulin on stimulation of renal sodium reabsorption [ 56 , 57 ].
The hyperinsulinemic-euglycemic clamp (HEC) technique, the gold standard for the diagnosis of insulin resistance, is not suitable for routine clinical work because of its high cost, time consuming and complicated procedures. Although homeostasis model assessment of IR (HOMA-IR) has a wide range of clinical application, it does have the disadvantage of relatively high cost and low reproducibility of measuring plasma insulin [ 58 ]. The relationship between TyG-BMI and HOMA-IR has been proven [ 17 ]. Unlike HEC and HOMA-IR, TyG-BMI does not require insulin but only FPG, TG, and BMI, and is a simple and effective indicator for the diagnosis of insulin resistance. This study further revealed that the ROC value of TyG-BMI for hypertension surpassed that of individual markers including TyG or BMI. Consequently, we have reason to believe that TyG-BMI holds promise in identifying high-risk individuals with hypertension. It is recommended that future prospective studies delve deeper into exploring the predictive value of TyG-BMI for hypertension.
This study has several advantages worth mentioning. (1) The study included 60,283 study subjects with a large and representative sample size. (2) After adjusting for confounders, a linear dose-response relationship between TyG-BMI and hypertension was confirmed. Subgroup analysis identified high-risk groups. With these reliable statistical analyses, the conclusions of this study can be considered quite reliable.
Some disadvantages of this research cannot be ignored. (1) This study is a cross-sectional study and can only confirm the association between TyG-BMI and hypertension, but not the causal relationship between TyG-BMI and hypertension. (2) Some study subjects may already know their blood pressure levels and thus have changed their lifestyles at the time of the survey, which may lead to Ney-man bias. This bias may misestimate the association between influencing factors and disease. (3) Given the cross-sectional design of this study, it presents a challenge to determine the temporal relationship between TyG-BMI and the onset of hypertension in the absence of longitudinal data. Consequently, the accurate assessment of TyG-BMI’s predictive value for hypertension is limited. To better understand the temporal association between these variables, longitudinal studies are warranted for further investigation. (4) Due to limitations of the survey, we were unable to compare the TyG-BMI with the hyperinsulinemic-euglycemic clamp (HEC) technique, the gold standard for the diagnosis of insulin resistance.
This cross-sectional study of a Chinese population showed an independent association between TyG-BMI and hypertension after adjusting for confounders, and this association was more pronounced in young and middle-aged populations. Furthermore, the AUC value of TyG-BMI for hypertension was higher than that of individual markers such as TyG or BMI, suggesting that TyG-BMI may serve as a novel target for the prevention and management of hypertension. Lifestyle modifications, encompassing physical exercise and adopting healthy dietary practices for weight management, play a crucial role in ameliorating IR and mitigating the risk of hypertension.
Data availability
The datasets used and/or analyzed during the current study are available from the corresponding author upon reasonable request.
Abbreviations
triglyceride glucose- body mass index
waist circumference
body mass index
systolic blood pressure
diastolic blood pressure
fasting plasma glucose
total cholesterol
triglyceride
high density lipoprotein cholesterol
low density lipoprotein cholesterol
standard deviation
Balakumar P, Maung-U K, Jagadeesh G. Prevalence and prevention of cardiovascular disease and diabetes mellitus. Pharmacol Res. 2016(Pt A);113:600–9.
Article PubMed Google Scholar
Peters SAE, Muntner P, Woodward M. Sex differences in the prevalence of, and trends in, cardiovascular risk factors, treatment, and control in the United States, 2001 to 2016. Circulation. 2019;139(8):1025–35.
Article Google Scholar
Wang ZW, Chen Z, Zhang LF, Wang X, Hao G, Zhang ZG, et al. Status of hypertension in China: results from the China hypertension survey, 2012–2015. Circulation. 2018;137(22):2344–56.
The writing committee of the report on cardiovascular health. Diseases in China. Report on cardiovascular health and diseases in China: an updated summary of 2020. Chin Cir J. 2021;36(8):521–45. Chinese.
Google Scholar
Mastrototaro L, Roden M. Insulin resistance and insulin sensitizing agents. Metabolism. 2021;125:154892.
Article CAS PubMed Google Scholar
Kahn SE, Cooper ME, Del Prato S. Pathophysiology and treatment of type 2 diabetes: perspectives on the past, present, and future. Lancet. 2014;383(9922):1068–83.
Wu HZ, Ballantyne CM. Metabolic inflammation and insulin resistance in obesity. Circ Res. 2020;126(11):1549–64.
McCracken E, Monaghan M, Sreenivasan S. Pathophysiology of the metabolic syndrome. Clin Dermatol. 2018;36(1):14–20.
Wang F, Han L, Hu D. Fasting insulin, insulin resistance and risk of hypertension in the general population: a meta-analysis. Clin Chim Acta. 2017;464:57–63.
Liu XZ, Fan J, Pan SJ. METS-IR, a novel simple insulin resistance indexes, is associated with hypertension in normal-weight Chinese adults. J Clin Hypertens (Greenwich). 2019;21(8):1075–81.
Ben-Dov IZ, Bursztyn M. Associations of arterial stiffness indices with measures of insulin resistance and renal sodium reabsorption. Am J Hypertens. 2019;32(9):810–2.
DeFronzo RA, Tobin JD, Andres R. Glucose clamp technique: a method for quantifying insulin secretion and resistance. Am J Physiol. 1979;237(3):E214–223.
Simental-Mendía LE, Rodríguez-Morán M, Guerrero-Romero F. The product of fasting glucose and triglycerides as surrogate for identifying insulin resistance in apparently healthy subjects. Metab Syndr Relat Disord. 2008;6(4):299–304.
Zheng RJ, Mao YS. Triglyceride and glucose (TyG) index as a predictor of incident hypertension: a 9-year longitudinal population-based study. Lipids Health Dis. 2017;16(1):175.
Zhu BR, Wang J, Chen K, Yan WH, Wang AP, Wang WQ, et al. A high triglyceride glucose index is more closely associated with hypertension than lipid or glycemic parameters in elderly individuals: a cross-sectional survey from the reaction study. Cardiovasc Diabetol. 2020;19(1):112.
Chen L, He L, Zheng W, Liu Q, Ren Y, Kong W, et al. High triglyceride glucose-body mass index correlates with prehypertension and hypertension in east Asian populations: a population-based retrospective study. Front Cardiovasc Med. 2023;25(10):1139842.
Er LK, Wu S, Chou HH, Hsu LA, Teng MS, Sun YC, et al. Triglyceride glucose-body mass index is a simple and clinically useful surrogate marker for insulin resistance in nondiabetic individuals. PLoS ONE. 2016;11(3):e0149731.
Lim JS, Kim JY, Koo SH, Kwon GC. Comparison of triglyceride glucose index, and related parameters to predict insulin resistance in Korean adults: an analysis of the 2007–2010 Korean national health and nutrition examination survey. PLoS ONE. 2019;14(3):e0212963.
Wang XY, Liu JD, Cheng ZY, Zhong YJ, Chen XH, Song W. Triglyceride glucose-body mass index and the risk of diabetes: a general population-based cohort study. Lipids Health Dis. 2021(1);20:99.
Du Z, Xing LY, Lin M, Sun YX. Estimate of prevalent ischemic stroke from triglyceride glucose-body mass index in the general population. BMC Cardiovasc Disord. 2020;20(1):483.
Wang R, Chen C, Xu G, Jin Z. Association of triglyceride glucose-body mass index and hemoglobin glycation index with heart failure prevalence in hypertensive populations: a study across different glucose metabolism status. Lipids Health Dis. 2024;23(1):53.
Khamseh ME, Malek M, Abbasi R, Taheri H, Lahouti M, Alaei-Shahmiri F. Triglyceride glucose index and related parameters (triglyceride glucose-body mass index and triglyceride glucose-waist circumference) identify nonalcoholic fatty liver and liver fibrosis in individuals with overweight/obesity. Metab Syndr Relat Disord. 2021;19(3):167–73.
Bala C, Gheorghe-Fronea O, Pop D, Pop C, Caloian B, Comsa H, et al. The association between six surrogate insulin resistance indexes and hypertension: a population-based study. Metab Syndr Relat Disord. 2019;17(6):328–33.
Li YX, You AJ, Tomlinson B, Yue LF, Zhao KJ, Fan HM, et al. Insulin resistance surrogates predict hypertension plus hyperuricemia. J Diabetes Investig. 2021;12(11):2046–53.
Nikbakht HR, Najafi F, Shakiba E, Darbandi M, Navabi J, Pasdar Y. Triglyceride glucose-body mass index and hypertension risk in iranian adults: a population-based study. BMC Endocr Disord. 2023; 21;23:156.
D’Elia L, Masulli M, Virdis A, Casiglia E, Tikhonoff V, Angeli F, et al. Triglyceride-glucose index and mortality in a large Regional-based Italian database (Urrah Project). J Clin Endocrinol Metab. 2024;14:dgae170.
Du JL, Chen YJ, Zhou N, Song YQ, Wang WW, Hong X. Associations between self-reported sleep duration and abnormal serum lipids in eastern China: a population-based cross-sectional survey. Sleep Med. 2022;95:1–8.
Ramdas Nayak VK, Nayak KR, Vidyasagar S. Predictive performance of traditional and novel lipid combined anthropometric indices to identify prediabetes. Diabetes Metab Syndr. 2020;14(5):1265–72.
Writing Group of 2018 Chinese Guidelines for the Management of Hypertension, Chinese Hypertension League, Chinese Society of Cardiology, Chinese Medical Doctor Association Hypertension Committee. Chinese guidelines for the management of hypertension (2018 revised Edition). Chin J Cardiovasc Med. 2019;24(1):24–56.
Writing Group of Chinese Guidelines for the Management of Dyslipidemia in Adults. Chinese guidelines for the management of dyslipidaemias in adults (2016 revised Edition). Chin Circ. 2016;31(10):937–50.
Chinese Society of Diabetes. Chinese guidelines for the management of type 2 diabetes (2017 Edition). Chin J Diabetes Mellitus. 2018;10(1):4–67.
Lin PMD, Chang KT, Lin YA, Tzeng IS, Chuang HH, Chen JY. Association between self-reported sleep duration and serum lipid profile in a middle-aged and elderly population in Taiwan: a community-based, cross-sectional study. BMJ Open. 2017(10);7:e015964.
Zhou L, Yu K, Yang LL, Wang H, Xiao Y, Qiu GK, et al. Sleep duration, midday napping, and sleep quality and incident stroke: the Dongfeng-Tongji cohort. Neurology. 2020;94(4):345–56.
Sanford NN, Sher DJ, Butler S, Xu X, Ahn C, D'Amico AV, et al. Cancer screening patterns among current, former, and never smokers in the United States, 2010–2015. JAMA Netw open. 2019;2:e193759.
Ma GS, Zhu DH, Hu XQ, Luan DC, Kong LZ. Yang XG. The drinking practice of people in China. Acta Nutrimenta Sinica. 2005;27:362–65. Chinese.
Xia YT, Wu YL, Wang LM, Zhang YL, Yan HM, Liu SB, et al. Underestimation of weight status among overweight and obese adults in China in 2013. Chin J Dis Control Prev. 2019;23(8):938–44.
Hu LH, Huang X, You CJ, Li JX, Hong K, Li P, et al. Prevalence of overweight, obesity, abdominal obesity and obesity-related risk factors in southern China. PLoS ONE. 2017;12(9):e0183934.
Hu N, Jiang Y, Li YC, Chen YD. Weighting method of china chronic disease surveillance data in 2010. Chin J Health Stats. 2012;29(3):424–26.
Sheng GT, Peng N, Hu C, Zhong L, Zhong MC, Zou Y. The albumin-to-alkaline phosphatase ratio as an independent predictor of future non-alcoholic fatty liver disease in a 5-year longitudinal cohort study of a non-obese Chinese population. Lipids Health Dis. 2021;20(1):50.
Desquilbet L, Mariotti F. Dose-response analyses using restricted cubic spline functions in public health research. Stat Med. 2010;29(9):1037–57.
Jiang CY, Yang RJ, Kuang MB, Yu M, Zhong MC, Zou Y. Triglyceride glucose-body mass index in identifying high-risk groups of pre-diabetes. Lipids Health Dis. 2021(1);20:161.
Zeng ZY, Liu SX, Xu H, Xu X, Liu XZ, Zhao XX. Association of triglyceride glucose index and its combination of obesity indices with prehypertension in lean individuals: a cross-sectional study of Chinese adults. J Clin Hypertens (Greenwich). 2020;22(6):1025–32.
Fang EF, Xie C, Schenkel JA, Wu C, Long Q, Cui H, et al. A research agenda for ageing in China in the 21st century (2nd edition): Focusing on basic and translational research, long-term care, policy and social networks. Ageing Res Rev. 2020; 64: 101174.
Peltzer K, Pengpid S, Sychareun V, Ferrer AJG, Low WY, Huu TN, et al. Prehypertension and psychosocial risk factors among university students in ASEAN countries. BMC Cardiovasc Disord. 2017(1);17:230.
Infurna FJ, Gerstorf D, Lachman ME. Midlife in the 2020s: opportunities and challenges. Am Psychol. 2020;75(4):470–85.
Sogari G, Velez-Argumedo C, Gómez MI, Mora C. College students and eating habits: a study using an ecological model for healthy behavior. Nutrients. 2018(12);10:1823.
American College of Obstetricians and Gynecologists’ Committee on Adolescent Health Care. Committee opinion 653 summary: concerns regarding social media and health issues in adolescents and young adults. Obstet Gynecol. 2016;127(2):414.
Waterhouse J, Bailey L, Tomlinson F, Edwards B, Atkinson G, Reilly T. Food intake in healthy young adults: effects of time pressure and social factors. Chronobiol Int. 2005;22(6):1069–92.
Wang R, Dai L, Zhong Y, Xie G. Usefulness of the triglyceride glucose-body mass index in evaluating nonalcoholic fatty liver disease: insights from a general population. Lipids Health Dis. 2021;20(1):77.
Jaacks LM, Vandevijvere S, Pan A, McGowan CJ, Wallace C, Imamura F, et al. The obesity transition: stages of the global epidemic. Lancet Diabetes Endocrinol. 2019;7(3):231–40.
Neeland IJ, Ross R, Després JP, Matsuzawa Y, Yamashita S, Shai I, et al. Visceral and ectopic fat, atherosclerosis, and cardiometabolic disease: a position statement. Lancet Diabetes Endocrinol. 2019(9);7:715–25.
Reaven G. Insulin resistance, hypertension, and coronary heart disease. J Clin Hypertens (Greenwich). 2003;5(4):269–74.
Reaven GM. Relationship between insulin resistance and hypertension. Diabetes Care. 1991;14Suppl:33–8.
Soleimani M. Insulin resistance and hypertension: new insights. Kidney Int. 2015;87(3):497–9.
Desai RV, Ahmed MI, Fonarow GC, Filippatos GS, White M, Aban IB, et al. Effect of serum insulin on the association between hyperuricemia and incident heart failure. Am J Cardiol. 2010;106(8):1134–8.
Horita S, Seki G, Yamada H, Suzuki M, Koike K, Fujita T. Insulin resistance, obesity, hypertension, and renal sodium transport. Int J Hypertens. 2011;2011:391762.
Article PubMed PubMed Central Google Scholar
Han TS, Lan L, Qu RG, Xu Q, Jiang RY, Na LX, et al. Temporal relationship between hyperuricemia and insulin resistance and its impact on future risk of hypertension. Hypertension. 2017;70(4):703–11.
Matthews DR, Hosker JP, Rudenski AS, Naylor BA, Treacher DF, Turner RC. Homeostasis model assessment: insulin resistance and beta-cell function from fasting plasma glucose and insulin concentrations in man. Diabetologia. 1985;28(7):412–9.
Download references
Acknowledgements
The authors thank all the participants, staffs, and investigators for the Chronic Disease and Risk Factor Surveillance in Nanjing.
Study funded by Nanjing Municipal Medical Science and Technique Development Foundation, China (ZKX18049, ZKX21054) and Medical Research Project of Jiangsu Provincial Health Commission in 2022 (M2022028) and Scientific Research Innovation Project of Nanjing Institute of Public Health, Nanjing Medical University in 2023(NCX2301).
Author information
Yijia Chen and Jinling Du contributed equally to this work.
Authors and Affiliations
Department of Chronic and Noncommunicable Disease Prevention, Nanjing Medical University Affiliated Nanjing Municipal Center for Disease Control and Prevention, Nanjing, 210003, China
Yijia Chen, Nan Zhou, Weiwei Wang & Xin Hong
Guangzhou Liwan Center for Disease Control and Prevention, Guangzhou, 510176, China
Department of Epidemiology and Biostatistics, School of Public Health, Nanjing Medical University, Nanjing, 211166, China
Nan Zhou & Xin Hong
Nanjing Gulou Center for Disease Control and Prevention, Nanjing, 210015, China
Yingqian Song
You can also search for this author in PubMed Google Scholar
Contributions
Authors’ contributionsConceptualization, X.H. and N.Z.; Methodology, Y.C., J.D. and X.H.; Investigation and resources, Y.C., J.D., N.Z., Y.S., W.W. and X.H.; Data curation and analysis, Y.C., J.D., Y.S. and W.W.; Writing, review, and/or revision of the manuscript, Y.C., J.D. and X.H.; Administrative and technical support, X.H. and N.Z.; Study supervision, X.H. and N.Z. All authors have read and agreed to the published version of the manuscript.
Corresponding author
Correspondence to Xin Hong .
Ethics declarations
Ethics approval and consent to participate.
The study was approved by the academic ethics committee of Nanjing Municipal Center for Disease Control and Prevention (approval number: PJ2017-B001-01). Informed consent was obtained from all individual participants included in the study.
Consent for publication
Not Applicable.
Competing interests
The authors declare no competing interests.
Additional information
Publisher’s note.
Springer Nature remains neutral with regard to jurisdictional claims in published maps and institutional affiliations.
Electronic supplementary material
Below is the link to the electronic supplementary material.
Supplementary Material 1
Supplementary material 2, rights and permissions.
Open Access This article is licensed under a Creative Commons Attribution 4.0 International License, which permits use, sharing, adaptation, distribution and reproduction in any medium or format, as long as you give appropriate credit to the original author(s) and the source, provide a link to the Creative Commons licence, and indicate if changes were made. The images or other third party material in this article are included in the article’s Creative Commons licence, unless indicated otherwise in a credit line to the material. If material is not included in the article’s Creative Commons licence and your intended use is not permitted by statutory regulation or exceeds the permitted use, you will need to obtain permission directly from the copyright holder. To view a copy of this licence, visit http://creativecommons.org/licenses/by/4.0/ . The Creative Commons Public Domain Dedication waiver ( http://creativecommons.org/publicdomain/zero/1.0/ ) applies to the data made available in this article, unless otherwise stated in a credit line to the data.
Reprints and permissions
About this article
Cite this article.
Chen, Y., Du, J., Zhou, N. et al. Correlation between triglyceride glucose-body mass index and hypertension risk: evidence from a cross-sectional study with 60,283 adults in eastern China. BMC Cardiovasc Disord 24 , 270 (2024). https://doi.org/10.1186/s12872-024-03934-8
Download citation
Received : 21 August 2023
Accepted : 09 May 2024
Published : 23 May 2024
DOI : https://doi.org/10.1186/s12872-024-03934-8
Share this article
Anyone you share the following link with will be able to read this content:
Sorry, a shareable link is not currently available for this article.
Provided by the Springer Nature SharedIt content-sharing initiative
- Hypertension
- Triglyceride
- Body mass index
- Triglyceride glucose-body mass index
BMC Cardiovascular Disorders
ISSN: 1471-2261
- Submission enquiries: [email protected]
- General enquiries: [email protected]

An official website of the United States government
The .gov means it’s official. Federal government websites often end in .gov or .mil. Before sharing sensitive information, make sure you’re on a federal government site.
The site is secure. The https:// ensures that you are connecting to the official website and that any information you provide is encrypted and transmitted securely.
- Publications
- Account settings
Preview improvements coming to the PMC website in October 2024. Learn More or Try it out now .
- Advanced Search
- Journal List
- Lippincott Open Access

Body Mass Index
The body mass index (BMI) is the metric currently in use for defining anthropometric height/weight characteristics in adults and for classifying (categorizing) them into groups. The common interpretation is that it represents an index of an individual’s fatness. It also is widely used as a risk factor for the development of or the prevalence of several health issues. In addition, it is widely used in determining public health policies.The BMI has been useful in population-based studies by virtue of its wide acceptance in defining specific categories of body mass as a health issue. However, it is increasingly clear that BMI is a rather poor indicator of percent of body fat. Importantly, the BMI also does not capture information on the mass of fat in different body sites. The latter is related not only to untoward health issues but to social issues as well. Lastly, current evidence indicates there is a wide range of BMIs over which mortality risk is modest, and this is age related. All of these issues are discussed in this brief review.
Body fatness has been an important psychosocial issue among humans for millennia. It is clearly manifested by paleolithic statuettes of exceedingly plump women. This suggests being “full figured” was highly desirable at least for women. In contrast, images of obese people, males or females, are never exhibited in ancient Egyptian funerary wall paintings, stellae, or statues suggesting that fatness was not considered to be a desirable trait there. This also is the case in artifacts from other cultures in the Middle East in that era. Why the degree of fatness has varied in different cultures is not clear. However, it may have depended on the availability of a reliable food supply and the effort required in obtaining it.
More recently, the degree of rotundity considered ideal also has varied considerably in the general population, but particularly for young women. Before the 1920s, “full figured” women were considered to be desirable as long as the distribution was hourglass in type. However, the 1920s Flapper era introduced abbreviated and revealing dresses. The result was that thinness was not only desirable but also required. This concept has moderated but still influences women’s views of beauty and eating habits at present.
Traditionally, a person’s fatness has been defined at a personal level as well as at a societal level. However, this is difficult to quantify. That is, each individual has his/her own perception of how fat he/she should be. As indicated above, this often depends on a general concept of societal norms or is due to peer pressure. For example, currently in Western societies, young women are often concerned about their body image, and most consider themselves to be too fat, even though they are well within population-based references. This is not only due to societal concepts of an ideal degree of fatness, but also due to thinness being a goal promulgated by the fashion industry and reinforced by commercial advertising.
At a societal level, although poorly described or quantified, there also is a degree of fatness beyond which a person generally is considered to be unacceptably fat; that is, there is an ill-defined threshold at which a person is labeled as being “fat” or “obese.” However, it is based on the “I can’t define it but I know it when I see it” concept. In addition, implicit in this context is that the location of the excess fat plays a role, as does a person’s age. It is much more acceptable to be “overweight” when one is old than when one is young. Also particularly in women, the accumulation of fat in certain areas of the body is considered to be much more acceptable than in other areas. For example, truncal (belly fat) accumulation would be considered to be less acceptable than the accumulation of fat in the peripelvic and thigh areas as well as in the breast area 1 ; that is, one may be statistically “fat” but with an appropriate figure be merely referred to “as amply endowed” or “pleasingly plump.”
The social consequences of being “too fat” are severe. Discrimination begins in childhood and results in serious emotional scars. Societal discrimination limits career choices, and indeed many career paths are closed to those considered to be too fat. Also, societal stigmatization often impairs a person’s ability to express his/her intellectual and other talents; that is, they become underachievers. In addition, the potential pool of mates is limited because of their perceived unattractiveness. Thus, obese people tend to marry other obese people and, parenthetically, to produce obese children. 2 – 4
Fatness as a Medical Issue
Not only the societal but also the functional and indirectly the medical consequences of an excessive accumulation of fat also have been recognized for millennia. Nevertheless, the concept that “body build” (fatness) is a major population-based medical issue gained popularity in this country only shortly before 1900. Life insurance data accumulated at that time 5 and subsequently 6 indicated that body weight, adjusted for height (Wt/Ht), was an independent determinant of life expectancy, and in 1910, the effects of being overweight were noted to be greater for younger people than for the elderly. 6
Subsequently, the Metropolitan Life Insurance Company in 1959 published tables of average body weights for heights (Wt/Ht) by gender and at different ages. 7 This was based on data from 1935 to 1953 from more than 4 million adults, mostly men, insured by 26 different insurance companies. The risk for development of certain diseases as well as mortality data related to Wt/Ht differences also were analyzed and reported in the 1960 Statistical Bulletin of the Metropolitan Life Insurance Co. 8 , 9
The Wt/Ht tables were used for many years as a reference for population-based studies. If a person’s Wt/Ht was 20% above or below the mean for that height category, he/she was considered to be overweight or underweight, respectively. The insurance data also indicated the ratios of weights for heights (the term used was “body build”) at which mortality was lowest in adults. The latter was referred to as the “ideal” or later the “desirable” weight. All of these data were periodically updated. 10 Interestingly, from 1959 to 1983, the desirable weight, that is, the weight/height representing the lowest mortality had increased. 10 – 12 However, a “desirable body” weight for height was invariably lower than the average weight for height in the insured population. 7 , 10 , 13
Early on it was recognized that tall people had a lower death rate than did short people 7 , 8 , 13 with the same Wt/Ht ratio. It also was recognized that a person’s height in general and leg length in particular could affect the calculated body mass adjusted for height. A person’s bony frame, that is, bone mass, also could affect the interpretation of this ratio. In general, it reflected whether one was narrowly or broadly built. Thus, efforts were made to eliminate lower limb length and frame size as variables. 7 , 10 The strategy was to develop representations of body build, that is, charts of weight/height that were independent of these variables. The overall goal was to have the same distribution of Wt/Ht at each level of height.
Although not stated, the implicit goal in developing these tables was to define a person’s fat mass as a proportion of their total mass, irrespective of their height or frame size. 14 Efforts were made to adjust for frame size (nonfat mass) by categorizing people as those with a small, medium, or large frame. Estimation of frame size was attempted using a number of measurements including shoulder width, elbow width, knee width, ankle width, and so on. 15 None of these were widely adopted. Nevertheless, frame size based on elbow width was included in the Metropolitan Life weight/height tables, 7 , 10 even though it was never validated.
Mathematically, the issue of adjusting body build for differences in height was approached with the concept that the body, particularly the trunk, could be considered as being a 3-dimensional volume or mass. Thus if a tall person were simply a scaled-up version of a short person, weight would increase approximately with the cube of height. 16 Indeed, several equations were developed and tested based on this concept; that is, the cube root of weight divided by height ( 3 √Wt/Ht) or weight/height, 3 and so on, but none were ideal. 17 This is because tall people are not just scaled-up versions of short people. As indicated previously, they tend to be more narrowly built resulting in a greater lean/fat proportion of body mass.
Later, it was shown that the body mass for height actually scaled best with weight for height when the height was raised to the 1.6 to 1.7 exponent (Wt/Ht, 1.6 etc). 18 Thus, with an increase in Ht, the effect of Ht on the ratio is exponential, whereas the change in Wt is linear. This has the effect of Ht on the ratio to be magnified as Ht increases. Overall, it results in a lower ratio in tall people than will be obtained with just a Wt/Ht ratio. Thus, it potentially compensates for a narrower build in tall compared with short people.
This exponent is not convenient for use in population-based studies, and it was determined that Wt/Ht 2 generally was satisfactory. 16 , 18 The latter represents the Quetelet Index. It was developed by Dr Quetelet in the 1800s.
I would like to briefly mention who Dr Quetelet was and how the “Quetelet Index” was derived. 19 – 21 Lambert Adolphe Jacque Quetelet (1796–1874) was a Flemish astronomer and statistician. Indeed, he is considered to be the patriarch of statisticians. He introduced the concept of “social averages.” In developing the “social average” concept, his goal was to determine the characteristics of an “average man” and the distribution of various human characteristics around the “average man.” Overall, it was his desire to obtain a distribution such that it formed a bell-shaped curve, that is, a Gaussian or normal distribution. He referred to his studies as “social physics.” Thus, this represents the first application of distribution mathematics to human characteristics. In 1835, Quetelet noted the body mass relationship to height in normal young adults was least affected by height when the ratio of weight to height squared was used rather than merely using the ratio of the weight to height or weight to height raised to the third power. 16
In 1972, Keys et al 16 severely criticized the validity of Metropolitan Life Insurance published data per se, and the then-published tables of desirable weight for height, as well as the tables used to define people who were underweight or overweight. 7 (The pejorative term “obese” was rarely used in that era.) Instead, Keys et al, using better documented weight for height data, popularized the Quetelet Index in population-based studies. They referred to it as the body mass index (BMI). Thus, Quetelet Index = body weight (kilograms) divided by height squared (meters) = BMI.
As indicated above, by squaring the height, it reduces the contribution of leg length in the equation and tends to normalize the body mass distribution at each level of height; that is, it reduces the effect of a variance in height in the relationship of weight to height. This was considered to be important because most of body fat is in the trunk. Nevertheless, as also pointed out by Keys et al, 16 even the BMI rather poorly represents a person’s percent of body fat.
Despite all the criticisms, the Metropolitan Life Tables criteria for defining obesity were widely used in the United States until the early 1990s. 22 – 24 At about that time, the World Health Organization (WHO) classification of body weight for height, based on the BMI, was published, 25 and later it was widely adopted. 26
Although a BMI determination reduces the effect of lower-extremity length on the Wt/Ht ratio, whether one uses the BMI or merely the ratio of weight to height, the population distribution is still not Gaussian. That is, it is not symmetrical but is always skewed to the right, that is, toward a higher ratio of weight (body mass) to height. For example, the distribution of BMIs in adult American men and women was determined in 1923 in 1026 individuals (Figure). 27 The median BMI was 24, but the mean BMI was 25. The distribution curve clearly indicated a skewing toward an increase in BMI, and this trend has continued. 26

Distribution of BMI in Adult American Men and Women (Carnegie Institute of Washington, Publ No 329. 1923). Adapted from Rony. 27 , p192
This skewing is not surprising because a markedly reduced BMI, theoretically and actually, would be incompatible with life because of an excessive reduction in lean as well as fat mass as a result of under nutrition 28 or disease. In contrast, excessive accumulation of body fat with maintenance or usually an increase in lean mass 29 , 30 is at least compatible with life, even though it may eventually affect long-term survival.
In 1993, the WHO assembled an Expert Consultation Group with a charge of developing uniform categories of the BMI. The results were published as a technical report in 1995. 25 Four categories were established: underweight, normal, overweight, and obese. An individual would be considered to be underweight if his/her BMI was in the range of 15 to 19.9, normal weight if the BMI was 20 to 24.9, overweight if the BMI was 25 to 29.9, and obese if it was 30 to 35 or greater. Using linear regression, a BMI of 16.9 in men and 13.7 in women represents a complete absence of body fat stores. 31
The above 4 categories are similar to those suggested by John S. Garrow in 1981, 31 , 32 but the terminology was changed. The terminology he used was “desirable” for a BMI up to 25, “grade I obesity” between 25 and 29.9, “grade II obesity,” between 30 and 40, and “grade III obesity” for BMI greater than 40.
The latter classification was based on Rosenbaum and colleagues’ 33 own data obtained in a survey of an adult population, aged 16 to 64 years, in Great Britain and published in 1985.
The population-based data indicated the majority of people were in the “desirable” range of the BMI distribution as indicated in Table Table1. 1 . Unfortunately, this distribution is not and has not been similar to those found in other surveys. The BMIs have been higher.
Garrow Classification

At the time that the WHO classification was published, the National Institutes of Health (NIH) in the United States classified people with a BMI of 27.8 (men) and 27.3 (women) or greater as being overweight. If they were below this BMI, they were considered to be “normal.” This was based on an 85% cutoff point of people examined in the National Health and Nutrition Examination Study (NHANES) II. 12 , 22 , 34 Subsequently, in 1998, the cutoff point between normal and overweight was reduced to a BMI of 25 to bring it into line with the 4 categories in the WHO guidelines. 25 , 35 Parenthetically, this instantaneously converted millions of Americans from being “normal weight” to being “overweight.”
In 1997, the International Obesity Task Force expanded the number of BMI categories to include different degrees of obesity and changed the terminology modestly. 36 A BMI of 25 to 29.9 is referred as “preobesity,” a BMI of 30 to 34.9 is class I obesity, 34.9 to 39.9 is class II obesity, and a BMI of 40 or greater is class III obesity. 37 , 38
The new terminology appears to be a bit presumptuous and careless because the BMI is not a direct measure of percent of fat mass, and the dynamic concept that those in the former “overweight” category are now in the “preobesity” category invariably going on to “obesity” is not the case. Also those with a lower BMI initially, but with a dynamic weight gain over time, would have to transition through this category in order to become classified as “obese” regardless of the terminology. By analogy, should those classified as “underweight” now be referred to as being “prenormal”?
It should be understood that in Western population-based studies, generally the mean or median BMI is about 24 to 27. 22 , 27 , 39 , 40 Thus, the consequence of adopting the WHO classification is that ~50% or more of the general adult population will always be in the overweight (now preobese) and obese categories. Indeed, the term “overweight” or particularly “preobesity” is prejudicial since people in this category are a major part of the expected normal distribution of BMI in the general population, and this has been the case for decades. Unfortunately, in discussing the so-called “obesity epidemic,” the number of people in the overweight (preobese) category generally is lumped together with those in the obese category in order to advertise and dramatize the perceived seriousness of this issue.
Regardless of the terminology and population reference issues, at present the BMI is the currency by which we define the obesity issue throughout the Western world. It was developed for the convenience of the epidemiologists, and indeed it did provide a uniform codification of body weight for height reporting. The BMI categories are shown in (Table (Table2 2 ).
Categories of BMI

A particular problem with BMI as an index of obesity is that it does not differentiate between body lean mass and body fat mass; that is, a person can have a high BMI but still have a very low fat mass and vice versa. 39 , 41 – 46
From an anatomical and metabolic perspective, the term obesity should refer to an excessive accumulation of body fat (triacylglycerols), and upon these grounds, the accuracy of the BMI as a determinant of body fat mass has been repeatedly questioned, 16 , 39 – 41 , 46 – 48 because it clearly has limitations in this regard. Gender, age, ethnic group, and leg length are important variables. 45 , 49 – 55 It should be noted that in population-based studies women generally have a BMI that is lower than that in men, even though their fat mass relative to their body build or BMI is considerably greater (~20% to 45%+).
The relatively poor correlation between percent of body fat mass and BMI in males has been known for many years 16 and was clearly shown in a study in which percent of body fat was determined by a densitometric method. 56 For men with a BMI of 27 in that study, the 95% confidence intervals for percent of body fat were 10% to 32%; that is, in this group, the percent of body fat varied from very little to that considered to be in the obesity range. (NIH-suggested criterion for obesity based on percent of body fat for men is ≥25%, and that for women is ≥35%. 57 )
The relatively poor correlation between percent of body fat mass and BMI also clearly has been shown more recently in the NHANES III database in which bioelectrical impedance was used to estimate the fat component of body composition. 51 In subjects with a BMI of 25 kg/m 2 , the percent of body fat in men varied between 14% and 35%, and in women it varied between 26% and 43%. Thus, using the NIH-suggested criterion based on percent of body fat to define obesity, subjects with a BMI of 25, a group that would be considered to be essentially normal, were associated with a body fat mass that varied again between low normal to obese. Also it is of interest that in the entire NHANES cohort, the BMI correlated better with lean body mass than with fat mass in men. 51 More recent NHANES data also indicate a poor correlation of BMI with percent of body fat, particularly in men. 58
In addition, in a recent study in individuals with or without diabetes in which the loss of lean body mass with aging was reported, the mean BMI in those without diabetes was 26.8. In those with diabetes, the BMI was 29.1; that is, it was higher as generally expected. However, the percent of lean body mass was the same; that is, the increased BMI in those with diabetes was not due only to an excessive accumulation of fat. 59
Over the past several decades, there has been an increase in BMI in the general population. This has resulted in predictions of a public health disaster. It should be recognized that in the United States during the period from 1960 to 2002 not only has the mean weight increased by 24 lb among men aged 20 to 74 years, but also the height has increased by about 1 in. We can then calculate that the weight increase per year has only been 0.57 lb, and as indicated above, this could be due to an increase in lean mass rather than fat mass, or it may be a combination of the two. In women, there was a similar increase in weight and height. 40
In an earlier report, life-insured men up to age 40 years were reported to be 0.5 to 1.5 inches taller and 2 to 9 lb heavier for the same height in 1959 than those studied 50 to 60 years prior to 1959. Also, in the earlier study, the mortality rate was lowest in those with higher weight-to-height ratios. This was attributed to the presence in the population of wasting diseases such as tuberculosis that resulted in an increased death rate. 13 Previously, a secular upward trend in height in adults in the United Kingdom also was reported. 60 In addition, in a twin study in the United Kingdom, children in 2005 were not only heavier but also taller than 1990 norms, whereas their BMIs were essentially the same. 3
Overall, the history of changes in height and weight in Western European men and probably women has been that of an increase in both weight and height. In the 17th century, the average height of men in Northern Europe was ~5 ft 3 in. It now approaches 6 ft. 61 These data suggest that the BMI categories should be adjusted upward periodically to accommodate population-based changes. Improvements in mortality rates also suggest an adjustment would be useful.
An additional limitation of the BMI is it does not capture body fat location information. This is an important variable in assessing the metabolic as well as mortality consequences of excessive fat accumulation. It was first recognized in France by Dr Jon Vague 62 in the 1940-1950s. He noted that accumulation of fat in the upper part of the body versus the lower part of the body was associated with an increased risk for coronary heart disease, diabetes, and also gallstones and gout. That is, individuals who accumulated excessive fat in the lower body segment were relatively spared from these complications. The body fat distribution was referred to as being “android” if it occurred in the upper body and “gynecoid” when it occurred in the lower segment of the body. This is because men tend to accumulate fat in the abdominal (upper body) area, whereas women tend to accumulate it in the peripelvic (gluteal) area and the thighs. A surrogate for this information has been to determine the abdominal circumference or an abdominal/hip circumference ratio. Subsequent data indicate that indeed the risk for development of diabetes and the so-called “metabolic syndrome,” as well as coronary heart disease, is more strongly related to the accumulation of upper body fat than lower body fat in both sexes. 63 – 67 That is, an android (male) distribution more closely predicts the development of the chronic diseases of aging than does the gynecoid (female) distribution.
More specifically, both visceral fat accumulation 68 , 69 and an expanded girth have been associated with development of insulin resistance, diabetes, and risk for coronary heart disease and hypertension. 63 , 64 , 70 – 74 Accumulation of fat in the abdominal area appears to correlate best with triacylglycerols accumulating in the liver and skeletal muscle. These may actually represent the pathogeneticially important metabolic consequences that result in insulin resistance and more directly correlate with development of the above adverse medical conditions. 68 , 75 , 76 Incidentally, the relatively small accumulation of fat in these organs would not be detectible by BMI determinations, and they do not correlate simply with total body fat mass. 75
Prior to puberty, boys and girls tend to be lean and not much different in this regard. Girls tend to accumulate relatively large amounts of fat during and after puberty, particularly in the peripelvic and thigh region; boys do not. During and after puberty, boys accumulate a relatively large amount of lean mass (bone and muscle) but not fat mass. In both sexes, these changes are reflected in an increased BMI. With aging, both sexes tend to develop fat in the upper part of the body (circumferentially), that is, the middle-age spread. 49 , 77 – 80 The reason for these changes in amount and distribution is not completely understood. Generally, it is considered to be due to hormonal changes.
It is of some interest that accumulation of fat in the lower body at puberty in females is unique to humans, is not present in any of the great apes, and most likely is estrogen mediated. 1
In a teleological sense, why this occurs, if due to estrogen, is uncertain. It could represent a means of maintaining body fat during pregnancy without an undue expansion in abdominal girth. It also may act as a counterbalance when women carry a child either during pregnancy or afterward. It also may be a space-filling fat site due to the relatively larger pelvis in postpubertal females. 81 Overall, it may represent an adaptation to the human upright bipedal posture. In any event, it results in a lower center of gravity among women compared with men. Indeed, the lower body segment in females becomes ~40% greater than in males (quoted in Singh, 1993), 1 and it has strong sex-related overtones.
Not only is thigh fat greater in women than in men, but also women generally have a preponderance of slow-twitch fibers, whereas men have a preponderance of fast-twitch fibers in their quadriceps muscles, as do upper-body-obese women, 82 suggesting either genetic or earlier developmental differentiation events. Could this be an adaptation for load-bearing versus speed as a group survival adaptation?
As indicated above, the accumulation of fat with aging in both sexes tends to occur in the truncal area and is associated with an increase in visceral fat. In women, this could be explained by a decrease in circulating estradiol, that is, a decreased estrogen/testosterone ratio associated with the menopause. (Again of some interest, it is only humans who have a defined menopause).
In men, with aging, there is a decrease in testosterone and a relative increase in estrogen, resulting in a decrease in the testosterone/estrogen ratio. 83 Thus, in men, a change in sex hormone concentrations could possibly explain the increased accumulation of fat in general. However, why there is a preferential accumulation in the truncal location, that is, why they too develop an increase in visceral fat, is unclear. Clearly, location of fat in this area would help to maintain mobility. The latter could be of great importance in hunter-gatherer societies and in defense of the tribe. Perhaps the distribution is programmed by gender earlier in life.
In this regard, it should be recognized that the accumulation of fat in certain body areas as well as the total amount of fat accumulated has a strong genetic or at least a familial component that diminishes with age. 3 , 27 , 84 , 85
At present, simple, accurate methods for measuring percent of body fat and, in particular, body fat in different fat depots are not available. The indirect methods currently in use for estimating total percent of body fat include underwater weighing, an air displacement and density determination using a Bod Pod, a bioelectrical impedance analyzer, and a determination of the isotopically labeled water mass. In the past, determination of the total body radioactive potassium and thus metabolizing tissue mass have been used to estimate lean body mass, and by difference, the fat mass. 86
Anthropometric determination of fat mass directly has been done using skin-fold thickness measured at various sites. 87 A dual-energy x-ray absorptiometry (DEXA) scan, which provides a 3-dimensional picture of body organ densities, can be used for estimating total body fat. Its location also can be determined. Single computed tomography (CT) slices of the abdomen and thigh can be used to obtain 2 dimensions of those fat depots from which a 3-dimensional fat area can be reconstructed. This also can be done using magnetic resonance imaging, but magnetic resonance imaging is very expensive. One cannot do serial sections of the body using CT to determine fat mass because of the excess radiation associated with this procedure.
Because of their convenience, bioelectric impedance methods or DEXA scans are the most commonly used to estimate the amount and, with DEXA scans, the location of body fat depots. Estimates of abdominal and thigh fat depots also can be estimated using CT slices. 52 , 72 , 88
All of the previously mentioned methods use certain assumptions in the calculation of body fat mass, and all are subject to potential error. Nevertheless, there are more specific methods of determining body fat mass than is the BMI. Important information regarding the location of the stored fat also can be determined with some methods.
It now is generally accepted that a relationship between BMI and mortality risk should be applied only to large populations. It should not be applied to an individual in an unqualified fashion. As indicated previously, there is the issue of being “overweight” versus “over fat.” In addition, a segment of the population is now considered to be “fat” by any criteria but “fit” and not at risk for early mortality. 74 , 75 , 89 – 91
The BMI classification system currently is being widely used in population-based studies to assess the risk for mortality in the different categories of BMI. It also is being used in regard to specific etiologies for mortality risk. However, as with its use to estimate percent of body fat, it is a rather crude approach. Even when some comorbidities are considered, the correlation of mortality rates with BMI often does not take into consideration such factors as family history of diabetes, hypertension, coronary heart disease, metabolic syndrome, dyslipidemias, familial longevity or the family prevalence of carcinomas, and so on. Recently it has been reported that more than 50% of susceptibility to coronary artery disease is accounted for by genetic variants. 92
Frequently, when correlations are made they also do not take into consideration a past as well as a current history of smoking, alcohol abuse, serious mental disorders or the duration of obesity, when in the life cycle it appeared, and whether the body weight is relatively stable or rapidly progressive, that is, type 1 or type 2 obesity. 93 , 94 In most population-based studies, only the initial weight and/or BMI are given, even though weight as well as fat stores are known to increase and height to decrease with aging. In addition, the rate of weight gain varies among individuals, 7 , 94 , 95 as does the loss of muscle mass. 95 Muscle mass has been correlated negatively with insulin resistance and prediabetes. 96 Lastly, population-based studies do not take into consideration the present and past history of a person’s occupation, medication-induced obesity, and how comorbidities are being treated. All of the above are significant issues.
Based on data in the literature, there are several additional problems in determining associations between BMI and overall death rate or, more specifically, cardiovascular events or death rates. Many obese people do not have cardiovascular risk factors, and in those who do, BMI no longer correlates with cardiovascular events 97 – 101 when the untoward effects of these other factors are factored out. Another issue is that the treatment status of the previously mentioned cardiovascular risk factors often is unknown or not stated; that is, the efficacy of treatment is rarely considered. This also is the case for diabetes. For example, the prevalence of diabetes has been increasing but not the disease-specific death rate. 102 Also, in people with diabetes, the death rate from cardiovascular disease has decreased dramatically. 102
Recently, there has been concern that an epidemic of obesity is occurring, not only in the United States, but also worldwide based on BMI data. In the NHANES data, there has been a change in the mean but to a greater extent in the distribution of BMI for adults aged 20 to 74 years in the United States. 26 That is, the mean BMI has increased, but there has been a greater increase in skewing toward the right and very large BMI. This results in more individuals being categorized as “obese.” The reason for the recent increase in mean BMI, but particularly in those in the obese category, is unknown, although there are many speculations. The dramatic decrease in smoking is likely to have been a contributor. 91 , 103 – 106 Smoking contributes to population-based BMI by at least 2 mechanisms. Smoking impairs appetite per se. It also is pathogenetically important in the development of chronic obstructive pulmonary disease, which itself results in a lower body mass. Of some interest, NHANES data also indicate that the trend of an increase in BMI has not continued since 1999 in women and only modestly in men. 58 Smoking rates also have stabilized at a low level.
Regardless of an observed increased skewing in the BMI distribution, it is important to note that several recent studies indicate that for most of us being a bit overweight (preobese?) as determined by BMI may not be so bad. 107 – 111
The EPIC observational study is a population-based study that includes 359 387 individuals aged 25 to 70 years living in Europe. 109 The mean age of this group at the initiation of the study was 51.5 years, and the mean follow-up has been 9.7 ± 2 years. In this study, both the crude and adjusted relative risk of death among men was actually the lowest in those with a BMI of 26.5 to 28, that is, those in the overweight (preobese) category. Also, a significant increase in risk of death was present only among those with a BMI of less than 21 or greater than 30. That is, there is a wide range of BMIs in the central part of this population in which there was relatively little impact of BMI on risk of death over a 9.7-year period.
Similar data were obtained in the NIH–American Association of Retired Persons study of 527 265 men and women between the ages of 50 and 71 years in the United States and followed for up to 10 years. 110 The lowest death rate in the entire cohort was among those in the “overweight” category, and this was particularly true among the men. There also was a broad range of BMIs over which there was little difference in mortality (BMI of 23.5 to 30).
The NHANES data going back to 1971 and up to 1994 also indicate that the relative mortality risk is lowest in men with a BMI of 25 to 30 in all age groups, that is, from the age of 25 years up to the age of 70 years. 107 In addition, the risk of mortality was little affected by a BMI from 18.5 up to a BMI of 30 in all age categories. Indeed, in those older than 70 years, there was little impact on the death rate even if they were in the obese category. Similar results have been reported for women in the NHANES reports. 112 The lowest mortality occurred with a BMI of 27.
In a Canadian study, the age-adjusted mortality rate over 13 years in men was essentially unchanged in those with a BMI of 18.5 up to 35, that is, from the Normal Weight category through the obesity class I category. In women, there was only a modest increase over the same range. 113
In summary, there is a large range of BMIs over which there is little association with the death rate. Generally, the range is from a BMI of 21 up to and often including 30. It is centered in the 24-to-28 BMI range. This information is not entirely new. Andres 114 in 1980 summarized 16 different population-based studies in which anthropometrically determined obesity was not associated with increased mortality rate. A detailed analysis in 1960 of the Metropolitan Life Insurance data also suggested little increase in mortality rates in people with a degree of overweight less than 20% or more above the average for a given height and age (quoted in Keys et al 97 ).
Interestingly, in the EPIC observational Study, 109 when the waist circumference–to–BMI ratio was calculated, that is, adjusting the waist circumference for BMI, it tended to linearize the association of BMI with risk for death, and the ratio was greatest for those with a low BMI. Thus, even if an individual had a low BMI but a relatively increased waist circumference, the risk was increased. Indeed, for any given BMI, a 5-cm increase in circumference increased the risk of death by a factor of 1.17 among men and 1.13 for women. Also in this study, the overall greatest mortality risk was in those individuals with the lowest BMI and not those with the highest BMI. Nevertheless, even in the category with the lowest BMI, adjusting for waist circumference affected the mortality rate negatively. This again indicates the importance of the location of body fat in addition to the total amount of fat accumulated.
A recent analysis of 50 prospective observational studies indicated the lowest mortality at a BMI of 23 to 25. However, these data were obtained in the 1970s and 1980s in an aggregate population with a mean BMI of 24.8, that is, lower than at present. The increased mortality at higher BMI’s was modest up to a BMI of 27.5, and the authors could account for the excess mortality largely on the risk factors known to be associated with obesity. The latter are currently being much better treated than in that era. 115
Overall, a major unresolved issue is which factor of the following is more important in the prediction of comorbidities such as cardiovascular disease, diabetes, hypertension, malignancies, or overall death rates. Is it BMI, total body fat mass, or the distribution of body fat, that is, visceral versus subcutaneous, or upper body fat accumulation (as determined by abdominal circumference, or a waist/hip ratio, or some combination of these, and so on)? The EPIC 109 data suggest that where fat is accumulated is much more important than merely the BMI, with the exception of those with an exceeding large total fat mass.
SUMMARY AND CONCLUSION
It is time to move beyond the BMI as a surrogate for determining body fat mass. Alternatively, if BMI continues to be used, the categories and definitions should be changed to reflect the current distribution of BMIs in the general population.
A better means than the BMI for estimating percent of body fat and its relationship to mortality and various morbidities clearly would be desirable.
The BMI was not originally developed for use specifically as an index of fatness in population-based studies. However, it has been coopted for this use because it is a readily obtained metric. It should be understood that the BMI has serious limitations when used as an indicator of percent of body fat mass. Indeed, it may be misleading in this regard, particularly in men. The terminology currently used also is prejudicial. By definition, one-half or more adults in the recent past and currently are overweight (preobese) or obese in Western, industrialized nations.
The current BMI classification system also is misleading in regard to effects of body fat mass on mortality rates. The role of fat distribution in the prediction of medically significant morbidities as well as for mortality risk is not captured by use of the BMI. Also, numerous comorbidities, lifestyle issues, gender, ethnicities, medically significant familial-determined mortality effectors, duration of time one spends in certain BMI categories, and the expected accumulation of fat with aging are likely to significantly affect interpretation of BMI data, particularly in regard to morbidity and mortality rates. Such confounders as well as the known clustering of obesity in families, the strong role of genetic factors in the development of obesity, the location in which excessive fat accumulates, its role in the development of type 2 diabetes and hypertension, and so on, need to be considered before promulgation of public health policies that are designed to apply to the general population and are based on BMI data alone.
Clearly, obesity, as determined by BMI, is not a monotypic, age-invariant condition requiring a general public health “preventative” approach. A BMI-determined categorization of an individual should not be used exclusively in counseling or in the design of a treatment regimen. In addition, when considering weight loss regimens, variations in body weight attributed to weight loss and dietary cycling may be hazardous. 116 – 120 They have been associated with an increased mortality rate. 116 , 117 , 119 , 121 – 124 The concept of starvation-associated obesity 125 , 126 also needs to be considered.
Prevention and/or Treatment of BMI-Determined Overweight or Obesity
Clearly episodic starvation or semistarvation regimens are not the answer, 127 nor are population-based efforts to increase fresh fruits and vegetables and tax soda pop, and so on. In my opinion, the major focus on prevention and treatment should be on those unfortunate individuals who are grossly obese, mechanically compromised, and who are at very high risk for death. 128 Surgical gastrointestinal intervention has proven to be at least partially successful in improving fuel regulation and storage. 129 , 130 Hopefully, medications will be developed that will reinstitute a metabolic fuel regulatory system that prevents the relentless accumulation of body fat, which is characteristic of those who are grossly obese. For others, an improvement in physical fitness may be salutary.
A Personal Perspective Regarding the Obesity Epidemic
Currently there are 4 truths regarding historical changes in body weights and the prevalence of obesity. People of Western European extraction are on average (1) heavier, (2) taller, and (3) more likely to be “overweight” or “obese” as defined by current BMI standards than those in other parts of the world. However, (4) it also should be pointed out they are healthier and are living longer than in any previous period in history. 131 , 132
Beginning in the 17th century, 61 the general underlying theme in all the studies done on weight gain in populations is an increase in height as well as weight. These changes are likely to be due to an increase in high-quality dietary protein (animal products), as well as an increased availability of total food energy in the diet. That is, there was not only an increase in food availability and variety, but also an increase in food quality. 133 The near elimination of chronic and serious acute infectious diseases also may have played a role, as has the dramatic decrease in cigarette smoking and its serious medical consequences.
The net effect of the above is that the chronic diseases of aging have become more of a public health problem, but better treatments are widely available. The prevalence of type 2 diabetes has increased, but overall the cardiovascular death rate has decreased dramatically. The death rate from malignancies is decreasing, and there has been a remarkable improvement in longevity, which is continuing. 131 The latter also is likely to continue into the future. 131 , 132
Some view the secular trend in the US population over the past 40 years as being one in which the population in general is “more obese, more diabetic, more arthritic, more disabled, and more medicated” but living longer. 134 A less sanguine view is indicated by others. 135 Many consider the overabundance of “calorie dense, processed foods,” the availability of soda pop, 136 and presence of fast-food restaurants and large food portion sizes to be strong, pathogenetic, obesity-inducing factors, 137 or more broadly, they consider obesity to be due to a “toxic” or “poisonous” food supply. 138 Some also are concerned that the increase in obesity (defined by BMI) will overwhelm any gains in health and life expectancy noted over the past several decades, that is, an Apocalypse awaits us. 139 I and others 140 , 141 do not share this pessimism.
Finally, I would like the political activists and doomsday prophets whose professional careers appear to depend on frightening the public and inducing politicians to pass restrictive laws without proven value, to be introduced to the prescient comments made by A. E. Harper 133 33 years ago. It is clear that currently we have a case of “déjà vu all over again.”
In regard to predicting the future, a wise person whose name I cannot recall stated presciently “Predicting the future is a fool’s playground”; the physicist Neils Bohr said, “Prediction is very difficult, especially about the future,” or as stated by that sage of the baseball world, Yogi Berra, “The future ain’t what it used to be.” Bertrand Russell said, “Fools and fanatics are always so sure of themselves, but wiser people are so full of doubt.” The true scientist should always be a skeptic.
Acknowledgments
The author thanks Rachel Anderson for help in preparing the manuscript for submission and Dr Mary C. Gannon for reading the manuscript and making numerous helpful comments.
The author has no conflicts of interest to disclose.

Prevalence of Obesity According to Body Mass Index, Waist Circumference, and Waist-to-Height Ratio in Peru: A Systematic Review and Meta-Analysis
- Find this author on Google Scholar
- Find this author on PubMed
- Search for this author on this site
- ORCID record for Luisa Erika Milagros Vásquez-Romero
- ORCID record for Fiorella E. Zuzunaga-Montoya
- ORCID record for Joan A. Loayza-Castro
- ORCID record for Enrique Vigil-Ventura
- ORCID record for Willy Ramos
- ORCID record for Víctor Juan Vera-Ponce
- For correspondence: [email protected]
- Info/History
- Preview PDF
Introduction Obesity is a global public health issue with significant health implications. However, studies on the prevalence of obesity in Peru have yielded varied results, highlighting the need for updated data to inform effective public health policies.
Objective The primary objective of this study is to determine the prevalence of obesity in Peru using three anthropometric measures: body mass index (BMI), waist circumference (WC), and waist-to-height ratio (WHtR).
Methods Between March and April 2024, a systematic review of published studies reporting the prevalence of obesity in Peru was conducted. The databases Scopus, Web of Science, Embase, PubMed, LILACS, and Scielo were Searched.
Results Overall, the prevalence of obesity was 23.23%, 38.90%, and 81.53% according to BMI, WC, and WHtR, respectively. However, these figures show wide variability, ranging from 13.10% to 37.4% according to BMI and from 19.4% to 51.6% according to WC. The highest reported prevalence of obesity by WHtR was 85.4%. Nonetheless, only a fraction of these studies were published in the last five years, and few specifically focused on obesity as the primary objective.
Conclusions The prevalence of obesity in Peru varies significantly depending on the anthropometric measure used. To improve the collection and frequency of data on obesity in Peru, it is recommended that cut-off points be standardized to be suitable for the country and that annual national surveys specifically designed for this purpose be implemented.
Competing Interest Statement
The authors have declared no competing interest.
Funding Statement
This study did not receive any funding
Author Declarations
I confirm all relevant ethical guidelines have been followed, and any necessary IRB and/or ethics committee approvals have been obtained.
I confirm that all necessary patient/participant consent has been obtained and the appropriate institutional forms have been archived, and that any patient/participant/sample identifiers included were not known to anyone (e.g., hospital staff, patients or participants themselves) outside the research group so cannot be used to identify individuals.
I understand that all clinical trials and any other prospective interventional studies must be registered with an ICMJE-approved registry, such as ClinicalTrials.gov. I confirm that any such study reported in the manuscript has been registered and the trial registration ID is provided (note: if posting a prospective study registered retrospectively, please provide a statement in the trial ID field explaining why the study was not registered in advance).
I have followed all appropriate research reporting guidelines, such as any relevant EQUATOR Network research reporting checklist(s) and other pertinent material, if applicable.
Luisa Erika Milagros Vásquez Romero, erikavr1995{at}gmail.com , Fiorella E. Zuzunaga-Montoya, fiorellazuzunaga{at}gmail.com , Joan A. Loayza-Castro, loayzacastrojoan{at}gmail.com , Enrique Vigil-Ventura, enrique.vigil{at}untrm.edu.pe , Willy Ramos, willymh98{at}hotmail.com , Víctor Juan
Data Availability
Data are available upon request from the corresponding author.
View the discussion thread.
Thank you for your interest in spreading the word about medRxiv.
NOTE: Your email address is requested solely to identify you as the sender of this article.

Citation Manager Formats
- EndNote (tagged)
- EndNote 8 (xml)
- RefWorks Tagged
- Ref Manager
- Tweet Widget
- Facebook Like
- Google Plus One
Subject Area
- Endocrinology (including Diabetes Mellitus and Metabolic Disease)
- Addiction Medicine (324)
- Allergy and Immunology (632)
- Anesthesia (168)
- Cardiovascular Medicine (2400)
- Dentistry and Oral Medicine (289)
- Dermatology (207)
- Emergency Medicine (381)
- Endocrinology (including Diabetes Mellitus and Metabolic Disease) (850)
- Epidemiology (11797)
- Forensic Medicine (10)
- Gastroenterology (705)
- Genetic and Genomic Medicine (3768)
- Geriatric Medicine (350)
- Health Economics (637)
- Health Informatics (2408)
- Health Policy (940)
- Health Systems and Quality Improvement (905)
- Hematology (342)
- HIV/AIDS (787)
- Infectious Diseases (except HIV/AIDS) (13347)
- Intensive Care and Critical Care Medicine (769)
- Medical Education (369)
- Medical Ethics (105)
- Nephrology (401)
- Neurology (3524)
- Nursing (199)
- Nutrition (529)
- Obstetrics and Gynecology (680)
- Occupational and Environmental Health (667)
- Oncology (1834)
- Ophthalmology (538)
- Orthopedics (221)
- Otolaryngology (287)
- Pain Medicine (234)
- Palliative Medicine (66)
- Pathology (447)
- Pediatrics (1037)
- Pharmacology and Therapeutics (426)
- Primary Care Research (424)
- Psychiatry and Clinical Psychology (3189)
- Public and Global Health (6184)
- Radiology and Imaging (1290)
- Rehabilitation Medicine and Physical Therapy (751)
- Respiratory Medicine (832)
- Rheumatology (380)
- Sexual and Reproductive Health (373)
- Sports Medicine (324)
- Surgery (405)
- Toxicology (50)
- Transplantation (172)
- Urology (147)

IMAGES
VIDEO
COMMENTS
Abstract and Figures. Background: The body mass index (BMI) was described back in 1832 by Adolphe Quetelet and then was validated by Ancel Keys in 1972. BMI is the ratio of body weight expressed ...
Abstract. Body mass index (BMI), a measurement based on a person's height and weight, allows the classification of individuals into categories such as obese or overweight. With these classifications, we can assess risk for hypertension, diabetes, cancer, hypercholesterolemia, and other chronic diseases. Furthermore, childhood BMI serves as a ...
The body mass index (BMI) is the metric currently in use for defining anthropometric height/weight characteristics in adults and for classifying (categorizing) them into groups. The common interpretation is that it represents an index of an individual's fatness. It also is widely used as a risk factor for the development of or the prevalence of ...
Abstract and Figures. The body mass index (BMI) is the metric currently in use for defining anthropometric height/weight characteristics in adults and for classifying (categorizing) them into ...
Abstract Obesity is a chronic, multifactorial, and morbid disease. In the United States, 69% of adults are ... fied by a body mass index (BMI) above 30 kg/m2.4 Obesity affects essentially every or-gan system in the body, and obesity is a risk factor for many morbid conditions, the most impactful of which are type 2 diabetes mellitus (T2DM ...
BMI as a Determinant of Body Fat Mass. A particular problem with BMI as an index of obesity is that it does not differentiate between body lean mass and body fat mass; that is, a person can have a high BMI but still have a very low fat mass and vice versa. 39,41-46 From an anatomical and metabolic perspective, the term obesity should refer to an excessive accumulation of body fat ...
We used Cox regression to estimate hazard ratios and 95% confidence intervals for an association between BMI and all-cause mortality, adjusting for age, study, physical activity, alcohol ...
The body mass index (BMI) is a widely used tool to eva-. luate overweight and obesity based on two anthropomet-. ric parameters, height and weight: BMI = weight/height. where weight is measured in ...
Background and aims: Emerging evidence has raised an obesity paradox in observational studies of body mass index (BMI) and health among the oldest-old (aged ≥80 years), as an inverse relationship of BMI with mortality was reported. This study was to investigate the causal associations of BMI, waist circumference (WC), or both with mortality in the oldest-old people in China.
Excess bodyweight, expressed as increased body-mass index (BMI), is associated with the risk of some common adult cancers. We did a systematic review and meta-analysis to assess the strength of associations between BMI and different sites of cancer and to investigate differences in these associations between sex and ethnic groups.
The Emerging Risk Factors Collaboration (ERFC) 1 analysed individual data from 58 prospective studies involving 221 934 people to assess body-mass index (BMI), waist circumference, and waist-to-hip ratio in the prediction of cardiovascular disease. The hazard ratio—adjusted for age, sex, and smoking—for coronary disease was 1·32 (95% CI 1·24-1·41) per 5 kg/m 2 higher baseline BMI ...
Within NBA players , BMI values range from 17 to 29.7 with a mean of 23.5 and a median of 23.6. BMI values within NFL players range from 20.9 to 43.0 with a mean of 28.5 and a median of 28.4. Histograms depicting the distribution of BMIs within the two leagues are shown in Fig. 5.1.
Body mass index was calculated and categorized according to the World Health Organization standard categorization: underweight (BMI <18.5), normal (BMI of 18.5-24.9), overweight (BMI of 25.0-29.9), and obesity (BMI ≥30). 28 The exploratory analysis used the following questions regarding aspirin use from the BQ and SQ, respectively: "During ...
Overall, 10 136 non-CVD deaths (40.8%) were related to cancer (eFigures 1, 2, and 3 in the Supplement ). Competing Risks of CVD Morbidity and Non-CVD Mortality by BMI Strata. The proportions of middle-aged adults who experienced incident CVD or non-CVD death stratified by sex and BMI groups are shown in Table 2.
Structured Graphical Abstract. Open in new tab Download slide. Opposite causal associations of body mass index (BMI) and waist circumference (WC) with mortality among Chinese oldest-old based on 1998-2018 Chinese Longitudinal Healthy Longevity Survey (CLHLS). ... For full access to this pdf, sign in to an existing account, or purchase an ...
A subgroup of four variables had the strongest association — the body-mass index, FEV 1 as a percentage of the predicted value, score on the MMRC dyspnea scale, and the distance walked in six ...
Body mass index (BMI) and lipid disorders are both known to be strongly associated with the development of diabetes, however, the indirect effect of lipid parameters in the BMI-related diabetes risk is currently unknown. This study aimed to investigate the mediating role of lipid parameters in the association of BMI with diabetes risk. We assessed the association of diabetes risk with BMI, as ...
Abstract Body mass index (BMI), a measurement based on a person's height and weight, allows the classification of ... Similar studies have been completed which analyze the relationship between body mass index and the probability of developing hypertension. Hu et al. [16] considered survey data from 17,441 Finnish individuals ...
ISBN 978-1-78985-005-5, eISBN 978-1-78985-006-2, PDF ISBN 978-1-83962-016-4, Published 2019-02-13 The body mass index has an important place in weight control. Attention should be paid to the regularization of anthropometric measures and to physical activity to protect from increasing obesity that is associated with chronic noncommunicable ...
Abstract. It's project time! Body-mass index (BMI) is a measurement model health-care professionals use to determine whether a person is overweight, and if so, by how much. To compute BMI, you need a person's weight in kilograms and height in meters. The BMI is simply weight/ height 2, converted to a unitless value.
Body mass index (BMI), a measureme nt based on a person's height and we ight, allows the classification of. individuals into categories such as ob ese or overweight. With these classific ations ...
Abstract Background: The prevalence of co-occurring heavy alcohol consumption and obesity is increasing in the United States. Despite neurobiological overlap in the regulation of alcohol consumption and eating behavior, alcohol- and body mass index (BMI)-related phenotypes show no or minimal genetic correlation. We hypothesized that the lack of ...
Main Outcomes and Measures Age- and sex-specific z scores of adiposity parameters (body mass index [BMI], fat mass index, waist-to-height ratio, and waist circumference) and cardiometabolic parameters (diastolic and systolic blood pressure, fasting plasma glucose, homeostasis model assessment for insulin resistance, high-density and low-density ...
Background Insulin resistance (IR) and obesity are established risk factors for hypertension, with triglyceride-glucose (TyG) serving as a recognized surrogate marker for IR. The aim of this study was to investigate the association between TyG-BMI and hypertension in the general population. Methods A total of 60,283 adults aged ≥18 years who underwent face-to-face questionnaires ...
Background. Women with a body mass index (BMI) >35 kg/m 2 carry an increased obstetric risk; however, the experience of the Class IV and above obese nulliparous women is less understood.. Aims. To describe maternal and perinatal outcomes in nulliparous women of booking BMI > 50 kg/m 2.. Materials and methods
Abstract. The body mass index (BMI) is the metric currently in use for defining anthropometric height/weight characteristics in adults and for classifying (categorizing) them into groups. The common interpretation is that it represents an index of an individual's fatness. It also is widely used as a risk factor for the development of or the ...
Introduction: Obesity is a global public health issue with significant health implications. However, studies on the prevalence of obesity in Peru have yielded varied results, highlighting the need for updated data to inform effective public health policies. Objective: The primary objective of this study is to determine the prevalence of obesity in Peru using three anthropometric measures: body ...
This study examined the association between low pre-pregnancy body mass index (BMI) and low birthweight using health insurance claims data and health checkup data, including weight measurements. Methods. We used health insurance claims data and health checkup data (JMDC, Tokyo, Japan) of women and their newborns in Japan between 2006 and 2020.
Abstract. Background: Early-stage breast cancer is a common condition with significant effects on health, both as a result of treatment and underlying. ... Body-mass index (BMI) within 1 month of initial biopsy was collected, and weight at 6 months and 1 year after initial biopsy was used to calculate the percentage change in weight. Treatment ...
The body mass index (BMI) is a commonly utilized measure for evaluating overweight and obesity in both children and adult populations. It serves as a measure of an individual's adiposity and is ...