Chemical Reaction Engineering
- First Online: 01 January 2014

Cite this chapter
- Hugo A. Jakobsen 2
5896 Accesses
3 Altmetric
Chemical reaction engineering (CRE) emerged as a methodology that quantifies the interplay between transport phenomena and kinetics on a variety of scales and allows formulation of quantitative models for various measures of reactor performance
This is a preview of subscription content, log in via an institution to check access.
Access this chapter
- Available as PDF
- Read on any device
- Instant download
- Own it forever
- Available as EPUB and PDF
- Compact, lightweight edition
- Dispatched in 3 to 5 business days
- Free shipping worldwide - see info
- Durable hardcover edition
Tax calculation will be finalised at checkout
Purchases are for personal use only
Institutional subscriptions
Recently a branch of CRE that is mainly focusing on transport phenomena and fluid flow analysis, rather than reaction kinetics, has emerged. By these groups the abbreviation CRE is frequently interpreted as chemical reactor engineering .
In Chap. 1 it was shown that when the total mole balance is used instead, the reaction term does not always vanish on the RHS because the number of moles may change in a chemical process. This total molar balance can be obtained starting out from the species mass balance ( 6.4 ), after dividing by the molecular mass for each species to obtain a species mole balance and finally sum these equations for all species in the mixture.
In classical thermodynamics a simple system is defined as a system that is macroscopically homogeneous, isotropic, and uncharged, that are large enough so that surface effects can be neglected, and that are not acted on by electric, magnetic, or gravitational fields [ 8 ]. A thermal reservoir is defined as a reversible heat source that is so large that any heat transfer of interest does not alter the temperature of the thermal reservoir. Such a thermal reservoir is a system enclosed by rigid impermeable walls and characterized by relaxation times sufficiently short so that all processes of interest therein are essentially quasi-static. Given two or more simple systems, they may be considered as constituting a single composite system. The composite system is termed closed if it is surrounded by a wall that is restrictive with respect to the total energy, the total volume, and the total mole numbers of each species of the composite system. In the present outline the universe is considered a composite system, the system represents a simple subsystem and the surroundings a thermal reservoir.
The Helmholtz free energy is sometimes denoted by \(\hat{A}\) ( \(J\) ).
The American chemist G. N. Lewis (1875–1946) introduced the fugacity function (Latin fugare , to fly) as a measure of the pressure adjusted for the lack of ideality [ 9 ]. For an ideal gas the fugacity is equal to the pressure. For a non-ideal gas we normally define the standard state to correspond to unit fugacity, \(f_i^0 = 1\) ( \(bar\) ).
This approach can also be adopted for liquid mixtures provided that an appropriate EOS is available.
The elements of Lagrangian mechanics are explained in Chap. 2 .
Duducovi \(\check{c}\) MP (1999) Trends in catalytic reaction engineering. Catal Today 48(1–4):5–15
Google Scholar
Froment GF, Hofmann HPK (1987) Design of fixed-bed gas-solid catalytic reactors. In: Carberry JJ, Varma A (eds) Chemical reaction and reaction engineering. Marcel Dekker Inc, New York and Basel, pp 373–440
Bird RB (1957) The equations of change and the macroscopic mass, momentum, and energy balances. Chem Eng Sci 6:123–181
Article Google Scholar
Fogler HS (2006) Elements of chemical reaction engineering, 4th edn. Pearson Education International, Upper Saddle River
Froment GF, Bischoff KB (1990) Chemical reactor analysis and design, 2nd edn. Wiley, New York
Ergun S (1952) Fluid flow through packed columns. Chem Eng Prog 48(2):89–94
Himmelblau DM, Bischoff KB (1968) Process analysis and simulation: deterministic systems. Wiley, New York
MATH Google Scholar
Callen HB (1985) Thermodynamics and an introduction to thermostatics, 2nd edn. Wiley, New York
Laidler KJ, Meiser JH (1995) Physical chemistry, 2nd edn. Houghton Mifflin Company, Boston
Smith WR, Missen RW (1982) Chemical reaction equilibrium analysis: theory and algorithms, 2nd edn. Wiley, New York
Jones JB, Dugan RE (1996) Engineering thermodynamics. Prentice-Hall International Inc, Englewood Cliffs
Prausnitz JM, Lichtenthaler RN, Azevedo EG de (1999) Molecular thermodynamics of fluid-phase equilibria, 3rd edn. Pretence Hall PTR, Upper Saddle Rive
Michelsen ML, Mollerup JM (2004) Thermodynamic models: fundamentals & computational aspects. Tie-Line Publications, Holte
White WB, Johnson SM, Dantzig GB (1958) Chemical equilibrium in complex mixtures. J Chem Phys 28(5):751–755
Greiner H (1991) An efficient implementation of Newton’s method for complex nonideal chemical equilibria. Comput Chem Eng 15:115–123
Article MathSciNet Google Scholar
Fletcher R (1981) Practical methods of optimization. Constrained optimization, Vol 2. Wiley, Chichester
Download references
Author information
Authors and affiliations.
Department of Chemical Engineering, Norwegian University of Science and Technology, Trondheim, Norway
Hugo A. Jakobsen
You can also search for this author in PubMed Google Scholar
Corresponding author
Correspondence to Hugo A. Jakobsen .
Rights and permissions
Reprints and permissions
Copyright information
© 2014 Springer International Publishing Switzerland
About this chapter
Jakobsen, H.A. (2014). Chemical Reaction Engineering. In: Chemical Reactor Modeling. Springer, Cham. https://doi.org/10.1007/978-3-319-05092-8_6
Download citation
DOI : https://doi.org/10.1007/978-3-319-05092-8_6
Published : 03 April 2014
Publisher Name : Springer, Cham
Print ISBN : 978-3-319-05091-1
Online ISBN : 978-3-319-05092-8
eBook Packages : Engineering Engineering (R0)
Share this chapter
Anyone you share the following link with will be able to read this content:
Sorry, a shareable link is not currently available for this article.
Provided by the Springer Nature SharedIt content-sharing initiative
- Publish with us
Policies and ethics
- Find a journal
- Track your research
- Architecture and Design
- Asian and Pacific Studies
- Business and Economics
- Classical and Ancient Near Eastern Studies
- Computer Sciences
- Cultural Studies
- Engineering
- General Interest
- Geosciences
- Industrial Chemistry
- Islamic and Middle Eastern Studies
- Jewish Studies
- Library and Information Science, Book Studies
- Life Sciences
- Linguistics and Semiotics
- Literary Studies
- Materials Sciences
- Mathematics
- Social Sciences
- Sports and Recreation
- Theology and Religion
- Publish your article
- The role of authors
- Promoting your article
- Abstracting & indexing
- Publishing Ethics
- Why publish with De Gruyter
- How to publish with De Gruyter
- Our book series
- Our subject areas
- Your digital product at De Gruyter
- Contribute to our reference works
- Product information
- Tools & resources
- Product Information
- Promotional Materials
- Orders and Inquiries
- FAQ for Library Suppliers and Book Sellers
- Repository Policy
- Free access policy
- Open Access agreements
- Database portals
- For Authors
- Customer service
- People + Culture
- Journal Management
- How to join us
- Working at De Gruyter
- Mission & Vision
- De Gruyter Foundation
- De Gruyter Ebound
- Our Responsibility
- Partner publishers

Your purchase has been completed. Your documents are now available to view.
Ahead of Print / Just Accepted
Issue of international journal of chemical reactor engineering.
Thank you for visiting nature.com. You are using a browser version with limited support for CSS. To obtain the best experience, we recommend you use a more up to date browser (or turn off compatibility mode in Internet Explorer). In the meantime, to ensure continued support, we are displaying the site without styles and JavaScript.
- View all journals
- Explore content
- About the journal
- Publish with us
- Sign up for alerts
- Perspective
- Published: 26 January 2023
Exploring catalytic reaction networks with machine learning
- Johannes T. Margraf ORCID: orcid.org/0000-0002-0862-5289 1 ,
- Hyunwook Jung 1 ,
- Christoph Scheurer 1 , 2 &
- Karsten Reuter ORCID: orcid.org/0000-0001-8473-8659 1
Nature Catalysis volume 6 , pages 112–121 ( 2023 ) Cite this article
6972 Accesses
25 Citations
12 Altmetric
Metrics details
- Computational chemistry
- Heterogeneous catalysis
Chemical reaction networks form the heart of microkinetic models, which are one of the key tools available for gaining detailed mechanistic insight into heterogeneous catalytic processes. The exploration of complex chemical reaction networks is therefore a central task in current catalysis research. Unfortunately, microscopic experimental information about which elementary reaction steps are relevant to a given process is almost always sparse, making the inference of networks from experiments alone almost impossible. While computational approaches provide important complementary insights to this end, their predictions also come with substantial uncertainties related to the underlying approximations and, crucially, the use of idealized structure models. In this Perspective, we aim to shine a light on recent applications of machine learning in the context of catalytic reaction networks, aiding both the inference of effective kinetic rate laws from experiment and the computational exploration of chemical reaction networks.
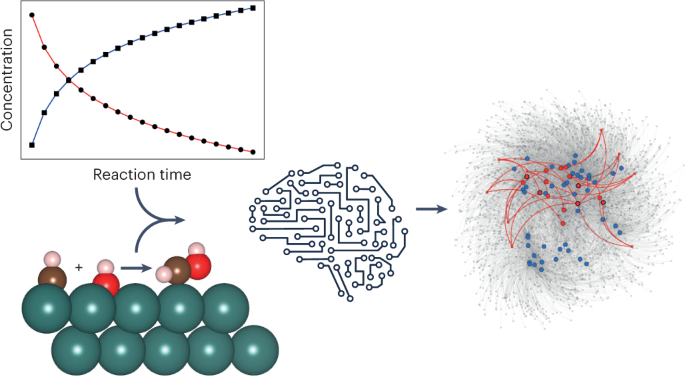
This is a preview of subscription content, access via your institution
Access options
Access Nature and 54 other Nature Portfolio journals
Get Nature+, our best-value online-access subscription
24,99 € / 30 days
cancel any time
Subscribe to this journal
Receive 12 digital issues and online access to articles
111,21 € per year
only 9,27 € per issue
Buy this article
- Purchase on Springer Link
- Instant access to full article PDF
Prices may be subject to local taxes which are calculated during checkout
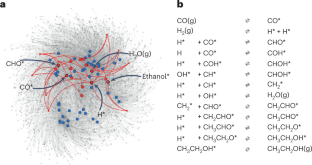
Similar content being viewed by others
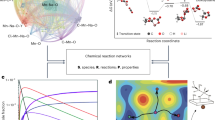
Chemical reaction networks and opportunities for machine learning
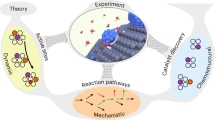
Bridging the complexity gap in computational heterogeneous catalysis with machine learning
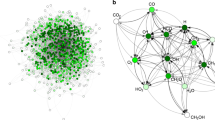
Machine learning in chemical reaction space
Pablo-García, S., García-Muelas, R., Sabadell-Rendón, A. & López, N. Dimensionality reduction of complex reaction networks in heterogeneous catalysis: from linear-scaling relationships to statistical learning techniques. Wiley Interdiscip. Rev. Comput. Mol. Sci. 11 , e1540 (2021).
Article Google Scholar
Ulissi, Z. W., Medford, A. J., Bligaard, T. & Nørskov, J. K. To address surface reaction network complexity using scaling relations machine learning and DFT calculations. Nat. Commun. 8 , 14621 (2017).
Article PubMed PubMed Central Google Scholar
Stocker, S., Csányi, G., Reuter, K. & Margraf, J. T. Machine learning in chemical reaction space. Nat. Commun. 11 , 5505 (2020).
Article CAS PubMed PubMed Central Google Scholar
Stamatakis, M. Kinetic modelling of heterogeneous catalytic systems. J. Phys. Condens. Matter 27 , 013001 (2015).
Article CAS PubMed Google Scholar
Sutton, J. E. & Vlachos, D. G. Building large microkinetic models with first-principles’ accuracy at reduced computational cost. Chem. Eng. Sci. 121 , 190–199 (2015).
Article CAS Google Scholar
Reuter, K. Ab initio thermodynamics and first-principles microkinetics for surface catalysis. Catal. Lett. 146 , 541–563 (2016).
Bruix, A., Margraf, J. T., Andersen, M. & Reuter, K. First-principles-based multiscale modelling of heterogeneous catalysis. Nat. Catal. 2 , 659–670 (2019).
Kim, Y., Kim, J. W., Kim, Z. & Kim, W. Y. Efficient prediction of reaction paths through molecular graph and reaction network analysis. Chem. Sci. 9 , 825–835 (2018).
Unsleber, J. P. & Reiher, M. The exploration of chemical reaction networks. Annu. Rev. Phys. Chem. 71 , 121–142 (2020).
Reuter, K., Plaisance, C. P., Oberhofer, H. & Andersen, M. Perspective: on the active site model in computational catalyst screening. J. Chem. Phys. 146 , 040901 (2017).
Article PubMed Google Scholar
Newton, M. A. Dynamic adsorbate/reaction induced structural change of supported metal nanoparticles: heterogeneous catalysis and beyond. Chem. Soc. Rev. 37 , 2644–2657 (2008).
Reuter, K., Frenkel, D. & Scheffler, M. The steady state of heterogeneous catalysis, studied by first-principles statistical mechanics. Phys. Rev. Lett. 93 , 116105 (2004).
Honkala, K. et al. Ammonia synthesis from first-principles calculations. Science 307 , 555–558 (2005).
Mills, G., Jónsson, H. & Schenter, G. K. Reversible work transition state theory: application to dissociative adsorption of hydrogen. Surf. Sci. 324 , 305–337 (1995).
Margraf, J. T. & Reuter, K. Systematic enumeration of elementary reaction steps in surface catalysis. ACS Omega 4 , 3370–3379 (2019).
Bligaard, T. et al. The Brønsted–Evans–Polanyi relation and the volcano curve in heterogeneous catalysis. J. Catal. 224 , 206–217 (2004).
Wang, S. et al. Universal Brønsted–Evans–Polanyi relations for C–C, C–O, C–N, N–O, N–N, and O–O dissociation reactions. Catal. Lett. 141 , 370–373 (2011).
del Río, E. G., Mortensen, J. J. & Jacobsen, K. W. Local Bayesian optimizer for atomic structures. Phys. Rev. B 100 , 104103 (2019).
Denzel, A. & Kästner, J. Gaussian process regression for geometry optimization. J. Chem. Phys. 148 , 094114 (2018).
Schmitz, G. & Christiansen, O. Gaussian process regression to accelerate geometry optimizations relying on numerical differentiation. J. Chem. Phys. 148 , 241704 (2018).
Chanussot, L. et al. Open Catalyst 2020 (OC20) dataset and community challenges. ACS Catal. 11 , 6059–6072 (2021).
Tran, R. et al. The Open Catalyst 2022 (OC22) dataset and challenges for oxide electrocatalysis. Preprint at https://arxiv.org/abs/2206.08917 (2022).
Musielewicz, J., Wang, X., Tian, T. & Ulissi, Z. W. Finetuna: fine-tuning accelerated molecular simulations. Mach. Learn. Sci. Technol. 3 , 03LT01 (2022).
Andersen, M. & Reuter, K. Adsorption enthalpies for catalysis modeling through machine-learned descriptors. Acc. Chem. Res. 54 , 2741–2749 (2021).
Xu, W., Reuter, K. & Andersen, M. Predicting binding motifs of complex adsorbates using machine learning with a physics-inspired graph representation. Nat. Comput. Sci. 2 , 443–450 (2022).
Li, Z., Wang, S., Chin, W. S., Achenie, L. E. & Xin, H. High-throughput screening of bimetallic catalysts enabled by machine learning. J. Mater. Chem. A 5 , 24131–24138 (2017).
Tran, K. & Ulissi, Z. W. Active learning across intermetallics to guide discovery of electrocatalysts for CO 2 reduction and H 2 evolution. Nat. Catal. 1 , 696–703 (2018).
García-Muelas, R. & López, N. Statistical learning goes beyond the d -band model providing the thermochemistry of adsorbates on transition metals. Nat. Commun. 10 , 1827 (2019).
Esterhuizen, J. A., Goldsmith, B. R. & Linic, S. Theory-guided machine learning finds geometric structure–property relationships for chemisorption on subsurface alloys. Chem 6 , 3100–3117 (2020).
Mamun, O., Winther, K. T., Boes, J. R. & Bligaard, T. A Bayesian framework for adsorption energy prediction on bimetallic alloy catalysts. npj Comput. Mater. 6 , 177 (2020).
Fung, V., Hu, G., Ganesh, P. & Sumpter, B. G. Machine learned features from density of states for accurate adsorption energy prediction. Nat. Commun. 12 , 88 (2021).
Bisbo, M. K. & Hammer, B. Efficient global structure optimization with a machine-learned surrogate model. Phys. Rev. Lett. 124 , 086102 (2020).
Deringer, V. L., Pickard, C. J. & Csányi, G. Data-driven learning of total and local energies in elemental boron. Phys. Rev. Lett. 120 , 156001 (2018).
Yamashita, T. et al. Crystal structure prediction accelerated by Bayesian optimization. Phys. Rev. Mater. 2 , 013803 (2018).
Kang, P.-L., Shang, C. & Liu, Z.-P. Large-scale atomic simulation via machine learning potentials constructed by global potential energy surface exploration. Acc. Chem. Res. 53 , 2119–2129 (2020).
Jørgensen, M. S., Larsen, U. F., Jacobsen, K. W. & Hammer, B. Exploration versus exploitation in global atomistic structure optimization. J. Phys. Chem. A 122 , 1504–1509 (2018).
Mortensen, H. L., Meldgaard, S. A., Bisbo, M. K., Christiansen, M.-P. V. & Hammer, B. Atomistic structure learning algorithm with surrogate energy model relaxation. Phys. Rev. B 102 , 075427 (2020).
Timmermann, J. et al. IrO 2 surface complexions identified through machine learning and surface investigations. Phys. Rev. Lett. 125 , 206101 (2020).
Timmermann, J. et al. Data-efficient iterative training of Gaussian approximation potentials: application to surface structure determination of rutile IrO 2 and RuO 2 . J. Chem. Phys. 155 , 244107 (2021).
Kaappa, S., del Río, E. G. & Jacobsen, K. W. Global optimization of atomic structures with gradient-enhanced Gaussian process regression. Phys. Rev. B 103 , 174114 (2021).
Ma, S., Huang, S.-D., Fang, Y.-H. & Liu, Z.-P. TiH hydride formed on amorphous black titania: unprecedented active species for photocatalytic hydrogen evolution. ACS Catal. 8 , 9711–9721 (2018).
Todorović, M., Gutmann, M. U., Corander, J. & Rinke, P. Bayesian inference of atomistic structure in functional materials. npj Comput. Mater. 5 , 35 (2019).
Westermayr, J., Chaudhuri, S., Jeindl, A., Hofmann, O. & Maurer, R. Long-range dispersion-inclusive machine learning potentials for structure search and optimization of hybrid organic–inorganic interfaces. Digit. Discov. 1 , 463–475 (2022).
Järvi, J., Todorović, M. & Rinke, P. Efficient modeling of organic adsorbates on oxygen-intercalated graphene on Ir(111). Phys. Rev. B 105 , 195304 (2022).
Jennings, P. C., Lysgaard, S., Hummelshøj, J. S., Vegge, T. & Bligaard, T. Genetic algorithms for computational materials discovery accelerated by machine learning. npj Comput. Mater. 5 , 46 (2019).
Jung, H., Sauerland, L., Stocker, S., Reuter, K. & Margraf, J. T. Machine-learning driven global optimization of surface adsorbate geometries. Preprint at ChemRxiv https://doi.org/10.26434/chemrxiv-2022-q3j0s-v2 (2022).
Peterson, A. A. Acceleration of saddle-point searches with machine learning. J. Chem. Phys. 145 , 074106 (2016).
Koistinen, O.-P., Dagbjartsdóttir, F. B., Ásgeirsson, V., Vehtari, A. & Jónsson, H. Nudged elastic band calculations accelerated with Gaussian process regression. J. Chem. Phys. 147 , 152720 (2017).
Denzel, A. & Kastner, J. Gaussian process regression for transition state search. J. Chem. Theory Comput. 14 , 5777–5786 (2018).
Torres, J. A. G., Jennings, P. C., Hansen, M. H., Boes, J. R. & Bligaard, T. Low-scaling algorithm for nudged elastic band calculations using a surrogate machine learning model. Phys. Rev. Lett. 122 , 156001 (2019).
Henkelman, G. & Jónsson, H. A dimer method for finding saddle points on high dimensional potential surfaces using only first derivatives. J. Chem. Phys. 111 , 7010–7022 (1999).
Koistinen, O.-P., Ásgeirsson, V., Vehtari, A. & Jónsson, H. Minimum mode saddle point searches using Gaussian process regression with inverse-distance covariance function. J. Chem. Theory Comput. 16 , 499–509 (2020).
Kang, P.-L., Shi, Y.-F., Shang, C. & Liu, Z.-P. Artificial intelligence pathway search to resolve catalytic glycerol hydrogenolysis selectivity. Chem. Sci. 13 , 8148–8160 (2022).
Shi, Y.-F., Kang, P.-L., Shang, C. & Liu, Z.-P. Methanol synthesis from CO 2 /CO mixture on Cu–Zn catalysts from microkinetics-guided machine learning pathway search. J. Am. Chem. Soc. 144 , 13401–13414 (2022).
Zhang, X.-J., Shang, C. & Liu, Z.-P. Stochastic surface walking reaction sampling for resolving heterogeneous catalytic reaction network: a revisit to the mechanism of water-gas shift reaction on Cu. J. Chem. Phys. 147 , 152706 (2017).
Sugita, Y. & Okamoto, Y. Replica-exchange molecular dynamics method for protein folding. Chem. Phys. Lett. 314 , 141–151 (1999).
Laio, A. & Parrinello, M. Escaping free-energy minima. Proc. Natl Acad. Sci. USA 99 , 12562–12566 (2002).
Foppa, L., Iannuzzi, M., Copéret, C. & Comas-Vives, A. Adlayer dynamics drives CO activation in Ru-catalyzed Fischer–Tropsch synthesis. ACS Catal. 8 , 6983–6992 (2018).
Xu, J., Cao, X.-M. & Hu, P. Accelerating metadynamics-based free-energy calculations with adaptive machine learning potentials. J. Chem. Theory Comput. 17 , 4465–4476 (2021).
Goldsmith, C. F. & West, R. H. Automatic generation of microkinetic mechanisms for heterogeneous catalysis. J. Phys. Chem. C 121 , 9970–9981 (2017).
Jafari, M. & Zimmerman, P. M. Uncovering reaction sequences on surfaces through graphical methods. Phys. Chem. Chem. Phys. 20 , 7721–7729 (2018).
Iwasa, T. et al. Combined automated reaction pathway searches and sparse modeling analysis for catalytic properties of lowest energy twins of Cu 13 . J. Phys. Chem. A 123 , 210–217 (2019).
Zhao, Q., Xu, Y., Greeley, J. & Savoie, B. M. Deep reaction network exploration at a heterogeneous catalytic interface. Nat. Commun. 13 , 1219 (2022).
Google Scholar
Bilodeau, C., Jin, W., Jaakkola, T., Barzilay, R. & Jensen, K. F. Generative models for molecular discovery: recent advances and challenges. Wiley Interdiscip. Rev. Comput. Mol. Sci. 12 , e1608 (2022).
Türk, H., Landini, E., Kunkel, C., Margraf, J. T. & Reuter, K. Assessing deep generative models in chemical composition space. Chem. Mater. 34 , 9455–9467 (2022).
Kim, S., Noh, J., Gu, G. H., Aspuru-Guzik, A. & Jung, Y. Generative adversarial networks for crystal structure prediction. ACS Cent. Sci. 6 , 1412–1420 (2020).
Noé, F., Olsson, S., Köhler, J. & Wu, H. Boltzmann generators: sampling equilibrium states of many-body systems with deep learning. Science 365 , eaaw1147 (2019).
Yoon, J. et al. Deep reinforcement learning for predicting kinetic pathways to surface reconstruction in a ternary alloy. Mach. Learn. Sci. Technol. 2 , 045018 (2021).
Sultan, M. M. & Pande, V. S. Automated design of collective variables using supervised machine learning. J. Chem. Phys. 149 , 094106 (2018).
Rogal, J., Schneider, E. & Tuckerman, M. E. Neural-network-based path collective variables for enhanced sampling of phase transformations. Phys. Rev. Lett. 123 , 245701 (2019).
Sun, L. et al. Multitask machine learning of collective variables for enhanced sampling of rare events. J. Chem. Theory Comput. 18 , 2341–2353 (2022).
Simm, G. N. & Reiher, M. Error-controlled exploration of chemical reaction networks with Gaussian processes. J. Chem. Theory Comput. 14 , 5238–5248 (2018).
Tran, K. et al. Methods for comparing uncertainty quantifications for material property predictions. Mach. Learn. Sci. Technol. 1 , 025006 (2020).
Winther, K. T. et al. Catalysis-Hub.org, an open electronic structure database for surface reactions. Sci. Data 6 , 75 (2019).
Blackmond, D. G. Kinetic profiling of catalytic organic reactions as a mechanistic tool. J. Am. Chem. Soc. 137 , 10852–10866 (2015).
Trunschke, A. Prospects and challenges for autonomous catalyst discovery viewed from an experimental perspective. Catal. Sci. Technol. 12 , 3650–3669 (2022).
Wehinger, G. D. et al. Quo vadis multiscale modeling in reaction engineering?—A perspective. Chem. Eng. Res. Des. 184 , 39–58 (2022).
Blackmond, D. G. Reaction progress kinetic analysis: a powerful methodology for mechanistic studies of complex catalytic reactions. Angew. Chem. Int. Ed. 44 , 4302–4320 (2005).
Burés, J. Variable time normalization analysis: general graphical elucidation of reaction orders from concentration profiles. Angew. Chem. Int. Ed. 55 , 16084–16087 (2016).
Nielsen, C. D.-T. & Burés, J. Visual kinetic analysis. Chem. Sci. 10 , 348–353 (2019).
Burés, J. What is the order of a reaction? Top. Catal. 60 , 631–633 (2017).
Box, G. E. P. & Draper, N. R. Response Surfaces, Mixtures, and Ridge Analyses (Wiley, 2007).
Book Google Scholar
Felsen, F., Reuter, K. & Scheurer, C. A model-free sparse approximation approach to robust formal reaction kinetics. Chem. Eng. J. 433 , 134121 (2022).
Trunschke, A. et al. Towards experimental handbooks in catalysis. Top. Catal. 63 , 1683–1699 (2020).
Temel, B., Meskine, H., Reuter, K., Scheffler, M. & Metiu, H. Does phenomenological kinetics provide an adequate description of heterogeneous catalytic reactions? J. Chem. Phys. 126 , 204711 (2007).
Download references
Acknowledgements
H.J. gratefully acknowledges support from the Alexander-von-Humboldt (AvH) Foundation.
Author information
Authors and affiliations.
Fritz-Haber-Institut der Max-Planck-Gesellschaft, Berlin, Germany
Johannes T. Margraf, Hyunwook Jung, Christoph Scheurer & Karsten Reuter
Institute of Energy and Climate Research (IEK-9), Forschungszentrum Jülich, Jülich, Germany
Christoph Scheurer
You can also search for this author in PubMed Google Scholar
Contributions
All authors contributed to the writing of the manuscript.
Corresponding authors
Correspondence to Johannes T. Margraf or Karsten Reuter .
Ethics declarations
Competing interests.
The authors declare no competing interests.
Peer review
Peer review information.
Nature Catalysis thanks Brett Savoie and the other, anonymous, reviewer(s) for their contribution to the peer review of this work.

Additional information
Publisher’s note Springer Nature remains neutral with regard to jurisdictional claims in published maps and institutional affiliations.
Rights and permissions
Springer Nature or its licensor (e.g. a society or other partner) holds exclusive rights to this article under a publishing agreement with the author(s) or other rightsholder(s); author self-archiving of the accepted manuscript version of this article is solely governed by the terms of such publishing agreement and applicable law.
Reprints and permissions
About this article
Cite this article.
Margraf, J.T., Jung, H., Scheurer, C. et al. Exploring catalytic reaction networks with machine learning. Nat Catal 6 , 112–121 (2023). https://doi.org/10.1038/s41929-022-00896-y
Download citation
Received : 25 July 2022
Accepted : 08 November 2022
Published : 26 January 2023
Issue Date : February 2023
DOI : https://doi.org/10.1038/s41929-022-00896-y
Share this article
Anyone you share the following link with will be able to read this content:
Sorry, a shareable link is not currently available for this article.
Provided by the Springer Nature SharedIt content-sharing initiative
This article is cited by
A human-machine interface for automatic exploration of chemical reaction networks.
- Miguel Steiner
- Markus Reiher
Nature Communications (2024)
Iterative design of training data to control intricate enzymatic reaction networks
- Bob van Sluijs
- Wilhelm T. S. Huck
Comprehensive exploration of graphically defined reaction spaces
- Qiyuan Zhao
- Sai Mahit Vaddadi
- Brett M. Savoie
Scientific Data (2023)
Accurate transition state generation with an object-aware equivariant elementary reaction diffusion model
- Chenru Duan
- Heather J. Kulik
Nature Computational Science (2023)
Machine-learning driven global optimization of surface adsorbate geometries
- Hyunwook Jung
- Lena Sauerland
- Johannes T. Margraf
npj Computational Materials (2023)
Quick links
- Explore articles by subject
- Guide to authors
- Editorial policies
Sign up for the Nature Briefing newsletter — what matters in science, free to your inbox daily.

Simulation of working of Chemical Reactor in LABVIEW platform
Ieee account.
- Change Username/Password
- Update Address
Purchase Details
- Payment Options
- Order History
- View Purchased Documents
Profile Information
- Communications Preferences
- Profession and Education
- Technical Interests
- US & Canada: +1 800 678 4333
- Worldwide: +1 732 981 0060
- Contact & Support
- About IEEE Xplore
- Accessibility
- Terms of Use
- Nondiscrimination Policy
- Privacy & Opting Out of Cookies
A not-for-profit organization, IEEE is the world's largest technical professional organization dedicated to advancing technology for the benefit of humanity. © Copyright 2024 IEEE - All rights reserved. Use of this web site signifies your agreement to the terms and conditions.
The Open Reaction Database
Research areas.
Data Management
General Science
Meet the teams driving innovation
Our teams advance the state of the art through research, systems engineering, and collaboration across Google.
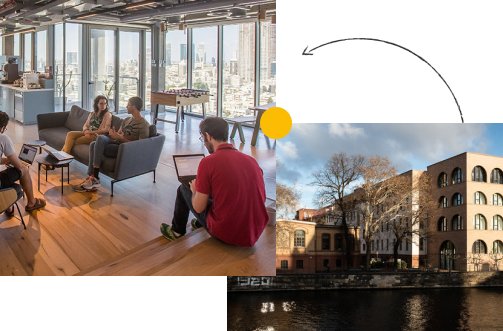
The Open Reaction Database
Affiliations.
- 1 Relay Therapeutics, Cambridge, Massachusetts 02139, United States.
- 2 Division of Chemistry and Chemical Engineering, California Institute of Technology, Pasadena, California 91125, United States.
- 3 Chemistry Capabilities Accelerating Therapeutics, Merck & Co., Inc., Kenilworth, New Jersey 07033, United States.
- 4 Google LLC, Mountain View, California 94043, United States.
- 5 Department of Chemistry & Biochemistry, University of California at Los Angeles, Los Angeles, California 90095, United States.
- 6 Chemical Research and Development, Pfizer Worldwide Research and Development, Groton, Connecticut 06340, United States.
- 7 Department of Chemical Engineering, Massachusetts Institute of Technology, Cambridge, Massachusetts 02139, United States.
- 8 Department of Electrical Engineering and Computer Science, Massachusetts Institute of Technology, Cambridge, Massachusetts 02139, United States.
- PMID: 34727496
- DOI: 10.1021/jacs.1c09820
Chemical reaction data in journal articles, patents, and even electronic laboratory notebooks are currently stored in various formats, often unstructured, which presents a significant barrier to downstream applications, including the training of machine-learning models. We present the Open Reaction Database (ORD), an open-access schema and infrastructure for structuring and sharing organic reaction data, including a centralized data repository. The ORD schema supports conventional and emerging technologies, from benchtop reactions to automated high-throughput experiments and flow chemistry. The data, schema, supporting code, and web-based user interfaces are all publicly available on GitHub. Our vision is that a consistent data representation and infrastructure to support data sharing will enable downstream applications that will greatly improve the state of the art with respect to computer-aided synthesis planning, reaction prediction, and other predictive chemistry tasks.
Publication types
- Research Support, Non-U.S. Gov't

Chemical Communications
In-situ synergistic reduced graphene oxide-boron carbon nitride nanosheets heterostructure for high-performance fabric-based supercapacitors.
We develop a new type of heterostructure nanocomposite made of reduced graphene oxide-boron carbon nitride nanosheets (rGO-BCN) by B-C covalent bonds. rGO-BCN nanocomposite delivers a large specific surface and excellent electrochemical properties, which are then constructed into flexible fabric-based high-performance supercapacitor electrodes based on the microfluidic electrospinning technology.
Supplementary files
- Supplementary information PDF (1180K)
Article information
Download citation, permissions.

Y. Zhang, Q. Huang, L. Zhou, H. Liu, C. Wang, L. Zhu and S. Chen, Chem. Commun. , 2024, Accepted Manuscript , DOI: 10.1039/D4CC01370K
To request permission to reproduce material from this article, please go to the Copyright Clearance Center request page .
If you are an author contributing to an RSC publication, you do not need to request permission provided correct acknowledgement is given.
If you are the author of this article, you do not need to request permission to reproduce figures and diagrams provided correct acknowledgement is given. If you want to reproduce the whole article in a third-party publication (excluding your thesis/dissertation for which permission is not required) please go to the Copyright Clearance Center request page .
Read more about how to correctly acknowledge RSC content .
Social activity
Search articles by author.
This article has not yet been cited.
Advertisements

IMAGES
VIDEO
COMMENTS
Abstract. Chemical reactors are pieces of equipment in which the feedstock is converted into the desired product. When selecting chemical reactors for specific tasks, economic costs, yield, purity, and pollution must be taken into account. Generally, reactors are chosen that will meet the requirements imposed by the reaction mechanisms, rate ...
Abstract. The term "chemical reactor" appears for the first time in open literature at the end of First World War, while in Germany the term "reaction technology" was coined in the 1950s, although chemical reactions and processes have been known for thousands of years. In the early days, reactors were furnaces and pots, which ...
Abstract. In chemical reactors, an enormous variety of possible regimes, both non-steady-state (transient) and steady-state can be observed. Steady-state reaction rates can be characterized by maxima and hystereses. Non-steady-state kinetic dependences may exhibit many phenomena of complex behavior, such as "fast" and "slow" domains ...
Kai Sundmacher, in Computer Aided Chemical Engineering, 2012. Abstract. In order to design optimal chemical reactors, the interaction between reactor and process needs to be considered. In this contribution we propose a new method for the design of optimal reactors in the overall process framework by simultaneous determination of the best ...
5.1 Introduction. Chemical reactors are the center of the all industrial chemical processes since they allow the transformation of the raw materials to products with high added value. This equipment defines the whole process, since the preparation process of raw materials depends on the reaction conditions as well as the effluents of the ...
2.1 Four Contexts of Chemical Reaction, 25 2.2 Chemical Formulas and Stoichiometric Coefficients, 26 2.3 Extent of a Chemical Reaction, 28 2.4 Independent and Dependent Chemical Reactions, 39 2.5 Characterization of the Reactor Feed, 47 2.5.1 Limiting Reactant, 48 2.5.2 Excess Reactant, 49 2.6 Characterization of Reactor Performance, 54
Abstract. Chemical reaction engineering (CRE) emerged as a methodology that quantifies the interplay between transport phenomena and kinetics on a variety of scales and allows formulation of quantitative models for various measures of reactor performance. Download chapter PDF.
Abstract. Chemical reactors are the heart of chemical and pharmaceutical plants. Tailored reactors with increased efficiency for specific applications can move industry a step forward towards a better environmental performance. Advances in manufacturing techniques expanded the possibilities to produce customized reactors. This work presents a ...
A hierarchical approach to chemical reactor engineering: an application to micro packed bed reactors ... Abstract. Hierarchical modeling is applied for the investigation of micro packed bed reactors. This method allows for the use of Computational Fluid Dynamics (CFD) simulations in the analysis of representative complex geometries, where a ...
The modelling of chemical reactors entails the description of mass, momentum, and energy transport equations, often at the same time. In addition, reactors with complex geometrical configurations are increasingly being applied in the fields, for instance, of thermochemical cycles and micro reactors. ... Graphical abstract. 14 pages, 20359 KiB
Abstract. Over the past decade, continuous flow reactors have emerged as a powerful tool for accelerated fundamental and applied studies of gas-liquid reactions, offering facile gas delivery and process intensification. In particular, unique features of highly gas-permeable tubular membranes in flow reactors ( i.e., tube-in-tube flow reactor ...
Chemical looping hydrogen generation represents a viable technology for high-purity hydrogen production and CO 2 capture. Moving bed reactors are considered effective for this process, but the high cost of experiments and the complexity of the biomass gas reaction have hindered the development of hydrogen generation from biomass gas.This investigation employs Computational Fluid Dynamics ...
Recent years have witnessed increased interest in systems that are capable of supporting multistep chemical processes without the need for manual handling of intermediates. These systems have been based either on collections of batch reactors 1 or on flow-chemistry designs 2-4 , both of which require considerable engineering effort to set up and control.
Abstract. The synergy between photocatalysis and continuous flow chemical reactors has shifted the paradigms of photochemistry, opening new avenues of research with safer and scalable processes that can be readily implemented in academia and industry. Current state-of-the-art photocatalysts are homogeneous transition metal complexes that have ...
Chemical reaction data in journal articles, patents, and even electronic laboratory notebooks are currently stored in various formats, often unstructured, which presents a significant barrier to downstream applications, including the training of machine-learning models. We present the Open Reaction Database (ORD), an open-access schema and infrastructure for structuring and sharing organic ...
Abstract. Chemical reaction networks form the heart of microkinetic models, which are one of the key tools available for gaining detailed mechanistic insight into heterogeneous catalytic processes ...
Abstract: Chemical reactors are extensively used in big industries for mixing products, chemical reactions, distillation & crystallization processes in many batch mode operations. For testing the working of a reactor with new concepts in control strategies, chemical reactions, stability & safety issues, a virtual reactor simulation is better than to test with a real reactor in field.
Due to the dramatic effect of the fluids' contact on reactor performance it is clear that CFD is to some extent revolutionizing chemical reaction engineering. Obviously, not only hydrodynamics is important. Heat transfer and chemical kinetics are integrated in CFD models in a natural way through the conservation equations.
Abstract. Chemical reaction data in journal articles, patents, and even electronic laboratory notebooks are currently stored in various formats, often unstructured, which presents a significant barrier to downstream applications, including the training of machine learning models. We present the Open Reaction Database (ORD), an open access ...
Herein, a portable flow reactor was designed and used to observe the chemical kinetics of a first-order process. Continuous chemical reactions were visualized via the flow reactor as static patterns with color gradients. The thermochromism of the rhodamine B salicylaldehyde hydrazone zinc (II) complex was visualized via the flow reactor and ...
Abstract Chemical reaction data in journal articles, patents, and even electronic laboratory notebooks are currently stored in various formats, often unstructured, which presents a significant barrier to downstream applications, including the training of machine-learning models. We present the Open Reaction Database (ORD), an open-access schema ...
Hopefully, incorporating the proposed correlations between the local temperature and the kinetics data into a computer control algorithm not only provides a simple tool for predicting the rate constant of the O-methylation reaction for other substituted phenols, but also, as a part of the chemical artificial intelligence, the optimum [11 C]MeI ...
Chemical Communications. ... Abstract. A tandem palladium-catalyzed Sonogashira coupling, propargyl-allenyl isomerization, and [2+2] cycloaddition sequence between electron-deficient haloarenes and 1,8-diynylic ethers is developed. ... The reaction shows good functional tolerance and proceeds under mild conditions to provide a new profile of ...
Chemical Communications. ... Abstract. We report Fe porphyrins bearing different meso-substituents for electrocatalytic CO2 reduction reaction (CO2RR). By replacing two and four meso-phenyl groups of Fe tetraphneylporphyrin (FeTPP) with strong electron-withdrawing pentafluorophenyl groups, we synthesized FeF10TPP and FeF20TPP, repectively. ...
Abstract We develop a new type of heterostructure nanocomposite made of reduced graphene oxide-boron carbon nitride nanosheets (rGO-BCN) by B-C covalent bonds. rGO-BCN nanocomposite delivers a large specific surface and excellent electrochemical properties, which are then constructed into flexible fabric-based high-performance supercapacitor ...