- Search Menu
- Browse content in Arts and Humanities
- Browse content in Archaeology
- Anglo-Saxon and Medieval Archaeology
- Archaeological Methodology and Techniques
- Archaeology by Region
- Archaeology of Religion
- Archaeology of Trade and Exchange
- Biblical Archaeology
- Contemporary and Public Archaeology
- Environmental Archaeology
- Historical Archaeology
- History and Theory of Archaeology
- Industrial Archaeology
- Landscape Archaeology
- Mortuary Archaeology
- Prehistoric Archaeology
- Underwater Archaeology
- Urban Archaeology
- Zooarchaeology
- Browse content in Architecture
- Architectural Structure and Design
- History of Architecture
- Residential and Domestic Buildings
- Theory of Architecture
- Browse content in Art
- Art Subjects and Themes
- History of Art
- Industrial and Commercial Art
- Theory of Art
- Biographical Studies
- Byzantine Studies
- Browse content in Classical Studies
- Classical Literature
- Classical Reception
- Classical History
- Classical Philosophy
- Classical Mythology
- Classical Art and Architecture
- Classical Oratory and Rhetoric
- Greek and Roman Archaeology
- Greek and Roman Epigraphy
- Greek and Roman Law
- Greek and Roman Papyrology
- Late Antiquity
- Religion in the Ancient World
- Digital Humanities
- Browse content in History
- Colonialism and Imperialism
- Diplomatic History
- Environmental History
- Genealogy, Heraldry, Names, and Honours
- Genocide and Ethnic Cleansing
- Historical Geography
- History by Period
- History of Agriculture
- History of Education
- History of Emotions
- History of Gender and Sexuality
- Industrial History
- Intellectual History
- International History
- Labour History
- Legal and Constitutional History
- Local and Family History
- Maritime History
- Military History
- National Liberation and Post-Colonialism
- Oral History
- Political History
- Public History
- Regional and National History
- Revolutions and Rebellions
- Slavery and Abolition of Slavery
- Social and Cultural History
- Theory, Methods, and Historiography
- Urban History
- World History
- Browse content in Language Teaching and Learning
- Language Learning (Specific Skills)
- Language Teaching Theory and Methods
- Browse content in Linguistics
- Applied Linguistics
- Cognitive Linguistics
- Computational Linguistics
- Forensic Linguistics
- Grammar, Syntax and Morphology
- Historical and Diachronic Linguistics
- History of English
- Language Variation
- Language Families
- Language Acquisition
- Language Evolution
- Language Reference
- Lexicography
- Linguistic Theories
- Linguistic Typology
- Linguistic Anthropology
- Phonetics and Phonology
- Psycholinguistics
- Sociolinguistics
- Translation and Interpretation
- Writing Systems
- Browse content in Literature
- Bibliography
- Children's Literature Studies
- Literary Studies (Modernism)
- Literary Studies (Asian)
- Literary Studies (European)
- Literary Studies (Eco-criticism)
- Literary Studies (Romanticism)
- Literary Studies (American)
- Literary Studies - World
- Literary Studies (1500 to 1800)
- Literary Studies (19th Century)
- Literary Studies (20th Century onwards)
- Literary Studies (African American Literature)
- Literary Studies (British and Irish)
- Literary Studies (Early and Medieval)
- Literary Studies (Fiction, Novelists, and Prose Writers)
- Literary Studies (Gender Studies)
- Literary Studies (Graphic Novels)
- Literary Studies (History of the Book)
- Literary Studies (Plays and Playwrights)
- Literary Studies (Poetry and Poets)
- Literary Studies (Postcolonial Literature)
- Literary Studies (Queer Studies)
- Literary Studies (Science Fiction)
- Literary Studies (Travel Literature)
- Literary Studies (War Literature)
- Literary Studies (Women's Writing)
- Literary Theory and Cultural Studies
- Mythology and Folklore
- Shakespeare Studies and Criticism
- Browse content in Media Studies
- Browse content in Music
- Applied Music
- Dance and Music
- Ethics in Music
- Ethnomusicology
- Gender and Sexuality in Music
- Medicine and Music
- Music Cultures
- Music and Culture
- Music and Religion
- Music and Media
- Music Education and Pedagogy
- Music Theory and Analysis
- Musical Scores, Lyrics, and Libretti
- Musical Structures, Styles, and Techniques
- Musicology and Music History
- Performance Practice and Studies
- Race and Ethnicity in Music
- Sound Studies
- Browse content in Performing Arts
- Browse content in Philosophy
- Aesthetics and Philosophy of Art
- Epistemology
- Feminist Philosophy
- History of Western Philosophy
- Metaphysics
- Moral Philosophy
- Non-Western Philosophy
- Philosophy of Action
- Philosophy of Law
- Philosophy of Religion
- Philosophy of Science
- Philosophy of Language
- Philosophy of Mind
- Philosophy of Perception
- Philosophy of Mathematics and Logic
- Practical Ethics
- Social and Political Philosophy
- Browse content in Religion
- Biblical Studies
- Christianity
- East Asian Religions
- History of Religion
- Judaism and Jewish Studies
- Qumran Studies
- Religion and Education
- Religion and Health
- Religion and Politics
- Religion and Science
- Religion and Law
- Religion and Art, Literature, and Music
- Religious Studies
- Browse content in Society and Culture
- Cookery, Food, and Drink
- Cultural Studies
- Customs and Traditions
- Ethical Issues and Debates
- Hobbies, Games, Arts and Crafts
- Lifestyle, Home, and Garden
- Natural world, Country Life, and Pets
- Popular Beliefs and Controversial Knowledge
- Sports and Outdoor Recreation
- Technology and Society
- Travel and Holiday
- Visual Culture
- Browse content in Law
- Arbitration
- Browse content in Company and Commercial Law
- Commercial Law
- Company Law
- Browse content in Comparative Law
- Systems of Law
- Competition Law
- Browse content in Constitutional and Administrative Law
- Government Powers
- Judicial Review
- Local Government Law
- Military and Defence Law
- Parliamentary and Legislative Practice
- Construction Law
- Contract Law
- Browse content in Criminal Law
- Criminal Procedure
- Criminal Evidence Law
- Sentencing and Punishment
- Employment and Labour Law
- Environment and Energy Law
- Browse content in Financial Law
- Banking Law
- Insolvency Law
- History of Law
- Human Rights and Immigration
- Intellectual Property Law
- Browse content in International Law
- Private International Law and Conflict of Laws
- Public International Law
- IT and Communications Law
- Jurisprudence and Philosophy of Law
- Law and Society
- Law and Politics
- Browse content in Legal System and Practice
- Courts and Procedure
- Legal Skills and Practice
- Primary Sources of Law
- Regulation of Legal Profession
- Medical and Healthcare Law
- Browse content in Policing
- Criminal Investigation and Detection
- Police and Security Services
- Police Procedure and Law
- Police Regional Planning
- Browse content in Property Law
- Personal Property Law
- Study and Revision
- Terrorism and National Security Law
- Browse content in Trusts Law
- Wills and Probate or Succession
- Browse content in Medicine and Health
- Browse content in Allied Health Professions
- Arts Therapies
- Clinical Science
- Dietetics and Nutrition
- Occupational Therapy
- Operating Department Practice
- Physiotherapy
- Radiography
- Speech and Language Therapy
- Browse content in Anaesthetics
- General Anaesthesia
- Neuroanaesthesia
- Browse content in Clinical Medicine
- Acute Medicine
- Cardiovascular Medicine
- Clinical Genetics
- Clinical Pharmacology and Therapeutics
- Dermatology
- Endocrinology and Diabetes
- Gastroenterology
- Genito-urinary Medicine
- Geriatric Medicine
- Infectious Diseases
- Medical Oncology
- Medical Toxicology
- Pain Medicine
- Palliative Medicine
- Rehabilitation Medicine
- Respiratory Medicine and Pulmonology
- Rheumatology
- Sleep Medicine
- Sports and Exercise Medicine
- Clinical Neuroscience
- Community Medical Services
- Critical Care
- Emergency Medicine
- Forensic Medicine
- Haematology
- History of Medicine
- Medical Ethics
- Browse content in Medical Dentistry
- Oral and Maxillofacial Surgery
- Paediatric Dentistry
- Restorative Dentistry and Orthodontics
- Surgical Dentistry
- Browse content in Medical Skills
- Clinical Skills
- Communication Skills
- Nursing Skills
- Surgical Skills
- Medical Statistics and Methodology
- Browse content in Neurology
- Clinical Neurophysiology
- Neuropathology
- Nursing Studies
- Browse content in Obstetrics and Gynaecology
- Gynaecology
- Occupational Medicine
- Ophthalmology
- Otolaryngology (ENT)
- Browse content in Paediatrics
- Neonatology
- Browse content in Pathology
- Chemical Pathology
- Clinical Cytogenetics and Molecular Genetics
- Histopathology
- Medical Microbiology and Virology
- Patient Education and Information
- Browse content in Pharmacology
- Psychopharmacology
- Browse content in Popular Health
- Caring for Others
- Complementary and Alternative Medicine
- Self-help and Personal Development
- Browse content in Preclinical Medicine
- Cell Biology
- Molecular Biology and Genetics
- Reproduction, Growth and Development
- Primary Care
- Professional Development in Medicine
- Browse content in Psychiatry
- Addiction Medicine
- Child and Adolescent Psychiatry
- Forensic Psychiatry
- Learning Disabilities
- Old Age Psychiatry
- Psychotherapy
- Browse content in Public Health and Epidemiology
- Epidemiology
- Public Health
- Browse content in Radiology
- Clinical Radiology
- Interventional Radiology
- Nuclear Medicine
- Radiation Oncology
- Reproductive Medicine
- Browse content in Surgery
- Cardiothoracic Surgery
- Gastro-intestinal and Colorectal Surgery
- General Surgery
- Neurosurgery
- Paediatric Surgery
- Peri-operative Care
- Plastic and Reconstructive Surgery
- Surgical Oncology
- Transplant Surgery
- Trauma and Orthopaedic Surgery
- Vascular Surgery
- Browse content in Science and Mathematics
- Browse content in Biological Sciences
- Aquatic Biology
- Biochemistry
- Bioinformatics and Computational Biology
- Developmental Biology
- Ecology and Conservation
- Evolutionary Biology
- Genetics and Genomics
- Microbiology
- Molecular and Cell Biology
- Natural History
- Plant Sciences and Forestry
- Research Methods in Life Sciences
- Structural Biology
- Systems Biology
- Zoology and Animal Sciences
- Browse content in Chemistry
- Analytical Chemistry
- Computational Chemistry
- Crystallography
- Environmental Chemistry
- Industrial Chemistry
- Inorganic Chemistry
- Materials Chemistry
- Medicinal Chemistry
- Mineralogy and Gems
- Organic Chemistry
- Physical Chemistry
- Polymer Chemistry
- Study and Communication Skills in Chemistry
- Theoretical Chemistry
- Browse content in Computer Science
- Artificial Intelligence
- Computer Architecture and Logic Design
- Game Studies
- Human-Computer Interaction
- Mathematical Theory of Computation
- Programming Languages
- Software Engineering
- Systems Analysis and Design
- Virtual Reality
- Browse content in Computing
- Business Applications
- Computer Games
- Computer Security
- Computer Networking and Communications
- Digital Lifestyle
- Graphical and Digital Media Applications
- Operating Systems
- Browse content in Earth Sciences and Geography
- Atmospheric Sciences
- Environmental Geography
- Geology and the Lithosphere
- Maps and Map-making
- Meteorology and Climatology
- Oceanography and Hydrology
- Palaeontology
- Physical Geography and Topography
- Regional Geography
- Soil Science
- Urban Geography
- Browse content in Engineering and Technology
- Agriculture and Farming
- Biological Engineering
- Civil Engineering, Surveying, and Building
- Electronics and Communications Engineering
- Energy Technology
- Engineering (General)
- Environmental Science, Engineering, and Technology
- History of Engineering and Technology
- Mechanical Engineering and Materials
- Technology of Industrial Chemistry
- Transport Technology and Trades
- Browse content in Environmental Science
- Applied Ecology (Environmental Science)
- Conservation of the Environment (Environmental Science)
- Environmental Sustainability
- Environmentalist Thought and Ideology (Environmental Science)
- Management of Land and Natural Resources (Environmental Science)
- Natural Disasters (Environmental Science)
- Nuclear Issues (Environmental Science)
- Pollution and Threats to the Environment (Environmental Science)
- Social Impact of Environmental Issues (Environmental Science)
- History of Science and Technology
- Browse content in Materials Science
- Ceramics and Glasses
- Composite Materials
- Metals, Alloying, and Corrosion
- Nanotechnology
- Browse content in Mathematics
- Applied Mathematics
- Biomathematics and Statistics
- History of Mathematics
- Mathematical Education
- Mathematical Finance
- Mathematical Analysis
- Numerical and Computational Mathematics
- Probability and Statistics
- Pure Mathematics
- Browse content in Neuroscience
- Cognition and Behavioural Neuroscience
- Development of the Nervous System
- Disorders of the Nervous System
- History of Neuroscience
- Invertebrate Neurobiology
- Molecular and Cellular Systems
- Neuroendocrinology and Autonomic Nervous System
- Neuroscientific Techniques
- Sensory and Motor Systems
- Browse content in Physics
- Astronomy and Astrophysics
- Atomic, Molecular, and Optical Physics
- Biological and Medical Physics
- Classical Mechanics
- Computational Physics
- Condensed Matter Physics
- Electromagnetism, Optics, and Acoustics
- History of Physics
- Mathematical and Statistical Physics
- Measurement Science
- Nuclear Physics
- Particles and Fields
- Plasma Physics
- Quantum Physics
- Relativity and Gravitation
- Semiconductor and Mesoscopic Physics
- Browse content in Psychology
- Affective Sciences
- Clinical Psychology
- Cognitive Neuroscience
- Cognitive Psychology
- Criminal and Forensic Psychology
- Developmental Psychology
- Educational Psychology
- Evolutionary Psychology
- Health Psychology
- History and Systems in Psychology
- Music Psychology
- Neuropsychology
- Organizational Psychology
- Psychological Assessment and Testing
- Psychology of Human-Technology Interaction
- Psychology Professional Development and Training
- Research Methods in Psychology
- Social Psychology
- Browse content in Social Sciences
- Browse content in Anthropology
- Anthropology of Religion
- Human Evolution
- Medical Anthropology
- Physical Anthropology
- Regional Anthropology
- Social and Cultural Anthropology
- Theory and Practice of Anthropology
- Browse content in Business and Management
- Business History
- Business Strategy
- Business Ethics
- Business and Government
- Business and Technology
- Business and the Environment
- Comparative Management
- Corporate Governance
- Corporate Social Responsibility
- Entrepreneurship
- Health Management
- Human Resource Management
- Industrial and Employment Relations
- Industry Studies
- Information and Communication Technologies
- International Business
- Knowledge Management
- Management and Management Techniques
- Operations Management
- Organizational Theory and Behaviour
- Pensions and Pension Management
- Public and Nonprofit Management
- Strategic Management
- Supply Chain Management
- Browse content in Criminology and Criminal Justice
- Criminal Justice
- Criminology
- Forms of Crime
- International and Comparative Criminology
- Youth Violence and Juvenile Justice
- Development Studies
- Browse content in Economics
- Agricultural, Environmental, and Natural Resource Economics
- Asian Economics
- Behavioural Finance
- Behavioural Economics and Neuroeconomics
- Econometrics and Mathematical Economics
- Economic Methodology
- Economic Systems
- Economic History
- Economic Development and Growth
- Financial Markets
- Financial Institutions and Services
- General Economics and Teaching
- Health, Education, and Welfare
- History of Economic Thought
- International Economics
- Labour and Demographic Economics
- Law and Economics
- Macroeconomics and Monetary Economics
- Microeconomics
- Public Economics
- Urban, Rural, and Regional Economics
- Welfare Economics
- Browse content in Education
- Adult Education and Continuous Learning
- Care and Counselling of Students
- Early Childhood and Elementary Education
- Educational Equipment and Technology
- Educational Strategies and Policy
- Higher and Further Education
- Organization and Management of Education
- Philosophy and Theory of Education
- Schools Studies
- Secondary Education
- Teaching of a Specific Subject
- Teaching of Specific Groups and Special Educational Needs
- Teaching Skills and Techniques
- Browse content in Environment
- Applied Ecology (Social Science)
- Climate Change
- Conservation of the Environment (Social Science)
- Environmentalist Thought and Ideology (Social Science)
- Natural Disasters (Environment)
- Social Impact of Environmental Issues (Social Science)
- Browse content in Human Geography
- Cultural Geography
- Economic Geography
- Political Geography
- Browse content in Interdisciplinary Studies
- Communication Studies
- Museums, Libraries, and Information Sciences
- Browse content in Politics
- African Politics
- Asian Politics
- Chinese Politics
- Comparative Politics
- Conflict Politics
- Elections and Electoral Studies
- Environmental Politics
- European Union
- Foreign Policy
- Gender and Politics
- Human Rights and Politics
- Indian Politics
- International Relations
- International Organization (Politics)
- International Political Economy
- Irish Politics
- Latin American Politics
- Middle Eastern Politics
- Political Theory
- Political Methodology
- Political Communication
- Political Philosophy
- Political Sociology
- Political Behaviour
- Political Economy
- Political Institutions
- Politics and Law
- Public Administration
- Public Policy
- Quantitative Political Methodology
- Regional Political Studies
- Russian Politics
- Security Studies
- State and Local Government
- UK Politics
- US Politics
- Browse content in Regional and Area Studies
- African Studies
- Asian Studies
- East Asian Studies
- Japanese Studies
- Latin American Studies
- Middle Eastern Studies
- Native American Studies
- Scottish Studies
- Browse content in Research and Information
- Research Methods
- Browse content in Social Work
- Addictions and Substance Misuse
- Adoption and Fostering
- Care of the Elderly
- Child and Adolescent Social Work
- Couple and Family Social Work
- Developmental and Physical Disabilities Social Work
- Direct Practice and Clinical Social Work
- Emergency Services
- Human Behaviour and the Social Environment
- International and Global Issues in Social Work
- Mental and Behavioural Health
- Social Justice and Human Rights
- Social Policy and Advocacy
- Social Work and Crime and Justice
- Social Work Macro Practice
- Social Work Practice Settings
- Social Work Research and Evidence-based Practice
- Welfare and Benefit Systems
- Browse content in Sociology
- Childhood Studies
- Community Development
- Comparative and Historical Sociology
- Economic Sociology
- Gender and Sexuality
- Gerontology and Ageing
- Health, Illness, and Medicine
- Marriage and the Family
- Migration Studies
- Occupations, Professions, and Work
- Organizations
- Population and Demography
- Race and Ethnicity
- Social Theory
- Social Movements and Social Change
- Social Research and Statistics
- Social Stratification, Inequality, and Mobility
- Sociology of Religion
- Sociology of Education
- Sport and Leisure
- Urban and Rural Studies
- Browse content in Warfare and Defence
- Defence Strategy, Planning, and Research
- Land Forces and Warfare
- Military Administration
- Military Life and Institutions
- Naval Forces and Warfare
- Other Warfare and Defence Issues
- Peace Studies and Conflict Resolution
- Weapons and Equipment


Dementia: A Very Short Introduction

Author webpage
- Cite Icon Cite
- Permissions Icon Permissions
Dementia: A Very Short Introduction explains how dementia is diagnosed, its different types and symptoms, and its effects on sufferers and their families. Why is dementia resistant to treatment? Why has the most successful scientific hypothesis not led to a cure? Are there variations between different countries, and given the rise in the ageing population, are there more or fewer cases than we think? This VSI looks at the history of dementia research and examines the genetic, physiological, and environmental risk factors and how individuals might reduce them. It also investigates developments in diagnosis and symptom management, and the economic and political context of dementia care.
Signed in as
Institutional accounts.
- GoogleCrawler [DO NOT DELETE]
- Google Scholar Indexing
Personal account
- Sign in with email/username & password
- Get email alerts
- Save searches
- Purchase content
- Activate your purchase/trial code
- Add your ORCID iD
Institutional access
Sign in with a library card.
- Sign in with username/password
- Recommend to your librarian
- Institutional account management
- Get help with access
Access to content on Oxford Academic is often provided through institutional subscriptions and purchases. If you are a member of an institution with an active account, you may be able to access content in one of the following ways:
IP based access
Typically, access is provided across an institutional network to a range of IP addresses. This authentication occurs automatically, and it is not possible to sign out of an IP authenticated account.
Sign in through your institution
Choose this option to get remote access when outside your institution. Shibboleth/Open Athens technology is used to provide single sign-on between your institution’s website and Oxford Academic.
- Click Sign in through your institution.
- Select your institution from the list provided, which will take you to your institution's website to sign in.
- When on the institution site, please use the credentials provided by your institution. Do not use an Oxford Academic personal account.
- Following successful sign in, you will be returned to Oxford Academic.
If your institution is not listed or you cannot sign in to your institution’s website, please contact your librarian or administrator.
Enter your library card number to sign in. If you cannot sign in, please contact your librarian.
Society Members
Society member access to a journal is achieved in one of the following ways:
Sign in through society site
Many societies offer single sign-on between the society website and Oxford Academic. If you see ‘Sign in through society site’ in the sign in pane within a journal:
- Click Sign in through society site.
- When on the society site, please use the credentials provided by that society. Do not use an Oxford Academic personal account.
If you do not have a society account or have forgotten your username or password, please contact your society.
Sign in using a personal account
Some societies use Oxford Academic personal accounts to provide access to their members. See below.
A personal account can be used to get email alerts, save searches, purchase content, and activate subscriptions.
Some societies use Oxford Academic personal accounts to provide access to their members.
Viewing your signed in accounts
Click the account icon in the top right to:
- View your signed in personal account and access account management features.
- View the institutional accounts that are providing access.
Signed in but can't access content
Oxford Academic is home to a wide variety of products. The institutional subscription may not cover the content that you are trying to access. If you believe you should have access to that content, please contact your librarian.
For librarians and administrators, your personal account also provides access to institutional account management. Here you will find options to view and activate subscriptions, manage institutional settings and access options, access usage statistics, and more.
Our books are available by subscription or purchase to libraries and institutions.
External resource
- In the OUP print catalogue
- About Oxford Academic
- Publish journals with us
- University press partners
- What we publish
- New features
- Open access
- Rights and permissions
- Accessibility
- Advertising
- Media enquiries
- Oxford University Press
- Oxford Languages
- University of Oxford
Oxford University Press is a department of the University of Oxford. It furthers the University's objective of excellence in research, scholarship, and education by publishing worldwide
- Copyright © 2024 Oxford University Press
- Cookie settings
- Cookie policy
- Privacy policy
- Legal notice
This Feature Is Available To Subscribers Only
Sign In or Create an Account
This PDF is available to Subscribers Only
For full access to this pdf, sign in to an existing account, or purchase an annual subscription.
Advertisement
Machine Learning for Dementia Prediction: A Systematic Review and Future Research Directions
- Original Paper
- Open access
- Published: 01 February 2023
- Volume 47 , article number 17 , ( 2023 )
Cite this article
You have full access to this open access article
- Ashir Javeed 1 , 2 na1 ,
- Ana Luiza Dallora 2 na1 ,
- Johan Sanmartin Berglund 2 ,
- Arif Ali 3 ,
- Liaqata Ali 4 &
- Peter Anderberg 2 , 5
16k Accesses
26 Citations
46 Altmetric
Explore all metrics
Nowadays, Artificial Intelligence (AI) and machine learning (ML) have successfully provided automated solutions to numerous real-world problems. Healthcare is one of the most important research areas for ML researchers, with the aim of developing automated disease prediction systems. One of the disease detection problems that AI and ML researchers have focused on is dementia detection using ML methods. Numerous automated diagnostic systems based on ML techniques for early prediction of dementia have been proposed in the literature. Few systematic literature reviews (SLR) have been conducted for dementia prediction based on ML techniques in the past. However, these SLR focused on a single type of data modality for the detection of dementia. Hence, the purpose of this study is to conduct a comprehensive evaluation of ML-based automated diagnostic systems considering different types of data modalities such as images, clinical-features, and voice data. We collected the research articles from 2011 to 2022 using the keywords dementia, machine learning, feature selection, data modalities, and automated diagnostic systems. The selected articles were critically analyzed and discussed. It was observed that image data driven ML models yields promising results in terms of dementia prediction compared to other data modalities, i.e., clinical feature-based data and voice data. Furthermore, this SLR highlighted the limitations of the previously proposed automated methods for dementia and presented future directions to overcome these limitations.
Similar content being viewed by others
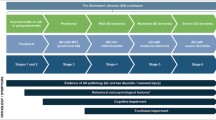
Diagnosis of Early Alzheimer’s Disease: Clinical Practice in 2021
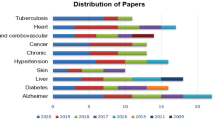
Artificial intelligence in disease diagnosis: a systematic literature review, synthesizing framework and future research agenda
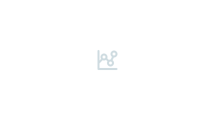
Alzheimer’s Disease: Epidemiology and Clinical Progression
Avoid common mistakes on your manuscript.
Introduction
Over a period of time, the advancements made in the field of medical science helped to increase the lifespan in the modern world [ 1 ]. This increased life expectancy raised the prevalence of neurocognitive disorders, affecting a significant part of the older population as well as global economies. In 2010, it was estimated that $604 billion have been spent on dementia patients in the USA alone[ 2 ]. The number of dementia patients is rapidly increasing worldwide, and statistical projections suggest that 135 million people might be affected by dementia by 2050 [ 3 ]. There are several risk factors that contribute to the development of dementia, including aging, head injury, and lifestyle. While age is the most prominent risk factor for dementia; figures suggest that a person at the age of 65 years old has 1–2% risk of developing dementia disease. By the age of 85 years old, this risk can reach to 30% [ 4 ].
Dementia is a mental disorder that is characterized by a progressive deterioration of cognitive functions that can affect daily life activities such as memory, problem solving, visual perception, and the ability to focus on a particular task. Usually, older adults are most vulnerable to dementia, and people take it as an inevitable consequence of aging, which is perhaps the wrong perception. Dementia is not a part of the normal ageing process; however, it should be considered a serious form of cognitive decline that affects your daily life. Actually, the primary cause for the development of dementia is the several diseases and injuries that affect the human brain [ 5 ]. Dementia is ranked on the seventh place in the leading causes of deaths in the world [ 6 ]. Furthermore, it is the major cause of disability and dependency among older people globally [ 6 ]. A change in the person’s ordinary mental functioning and obvious signs of high cognitive deterioration are required for a diagnosis of dementia [ 7 ]. Figure 1 presents the progression of dementia with age.
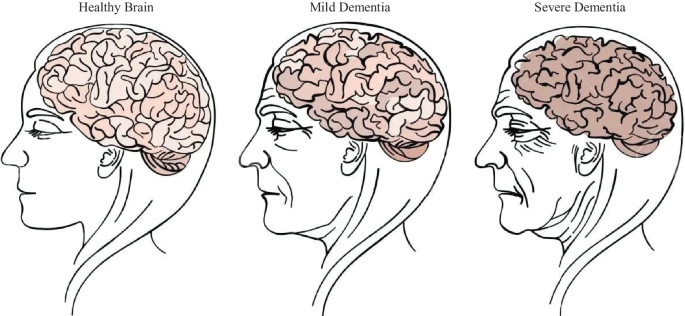
Progression of dementia disease with ageing
Types of dementia
Dementia is not a single disease, but, it is used as a generic term for several different cognitive disorders. Figure 2 provides the overview of different types of dementia along with the percentage of particular dementia type occurrence in the patients [ 8 ]. To have a better idea about dementia, we have studied common types of dementia for better problem awareness.
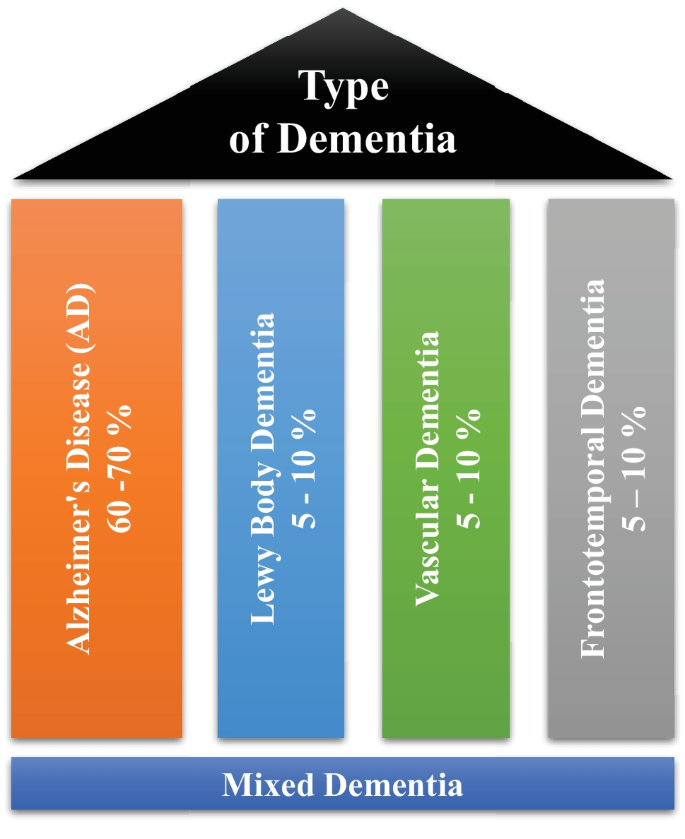
Types of dementia disease
Alzheimer’s disease
Alzheimer’s disease (AD) is thought to develop when abnormal amounts of amyloid beta (A \(\beta\) ) build up in the brain, either extracellularly as amyloid plaques, tau proteins or intracellularly as neurofibrillary tangles, affecting neuronal function, connectivity and leading to progressive brain function loss [ 9 ]. This diminished ability to eliminate proteins with ageing is regulated by brain cholesterol [ 10 ] and is linked to other neurodegenerative illnesses [ 11 ]. Except for 1–2% of cases where deterministic genetic anomalies have been discovered, the aetiology of the majority of Alzheimer’s patients remains unexplained [ 12 ]. The amyloid beta (A \(\beta\) ) hypothesis and the cholinergic hypothesis are two competing theories presented to explain the underlying cause of AD [ 13 ].
Vascular dementia
Vascular dementia (VaD) is a subtype of dementia caused by problems with the brain’s blood flow, generally in the form of a series of minor strokes, which results in a slow decline of cognitive capacity [ 14 ]. The VaD refers to a disorder characterized by a complicated mix of cerebrovascular illnesses that result in structural changes in the brain, as a result of strokes and lesions, which lead to cognitive impairment. A chronological relationship between stroke and cognitive impairments is necessary to make the diagnosis [ 15 ]. Ischemic or hemorrhagic infarctions in several brain areas, such as the anterior cerebral artery region, the parietal lobes, or the cingulate gyrus, are associated with VaD. In rare cases, infarcts in the hippocampus or thalamus might cause dementia [ 16 ]. A stroke increases the risk of dementia by 70%, whereas a recent stroke increases the risk by almost 120% [ 17 ]. Brain vascular lesions can also be caused by diffuse cerebrovascular disease, such as small vessel disease [ 18 ]. Risk factors for VaD include age, hypertension, smoking, hypercholesterolemia, diabetes mellitus, cardiovascular disease, and cerebrovascular sickness; geographic origin, genetic proclivity, and past strokes are also risk factors [ 19 ]. Cerebral amyloid angiopathy, which develops when beta amyloid accumulates in the brain, can occasionally lead to vascular dementia.
Lewy body dementia
Lewy body dementia (LBD) is a subtype of dementia characterized by abnormal deposits of the protein alpha-synuclein in the brain. These deposits, known as Lewy bodies, affect brain chemistry, causing problems with thinking, movement, behavior, and mood. Lewy body dementia is one of the most common causes of dementia [ 20 ]. Progressive loss of mental functions, visual hallucinations, as well as changes in alertness and concentration are prevalent in persons with LBD. Other adverse effects include tight muscles, delayed movement, difficulty walking, and tremors, all of which are also signs and symptoms of Parkinson’s disease [ 21 ]. LBD might be difficult to identify. Early LBD symptoms are commonly confused with those of other brain diseases or mental problems. Lewy body dementia can occur alone or in conjunction with other brain disorders [ 22 ]. It is a progressive disorder, which means that symptoms emerge gradually and worsen with time. A timespan of five to eight years is averaged, although it can last anywhere from two to twenty years for certain people [ 23 ]. The rate at which symptoms arise varies greatly from person to person, depending on overall health, age, and the severity of symptoms.
Frontotemporal dementia
Frontotemporal Dementia (FTD) is a subtype of dementia characterized by nerve cell loss in the frontal and temporal lobes of the brain [ 24 ]. As a result, the lobes contract. FTD can have an impact on behavior, attitude, language, and movement. This is one of the most common dementias in people under the age of 65. FTD most commonly affects persons between the ages of 40 and 65; however, it may also afflict young adults and older individuals [ 25 ]. The lobes decrease, and behavior, attitude, language, and mobility can all be affected by FTD. FTD affects both men and women equally. Dissociation from family, extreme oniomania, obscene speech, screaming, and the inability to regulate emotions, behavior, personality, and temperament are examples of social display patterns caused by FTD [ 26 ]. The symptoms of FTD appeared several years prior to visiting a neurologist [ 27 ].
Mixed Dementia (MD)
Mixed dementia occurs, when more than one kind of dementia coexists in a patient, and it is estimated to happen in around 10% of all dementia cases [ 6 ]. AD and VaD dementia are the two subtypes that are most common in MD [ 28 ]. This case is usually associated with factors such as old age, high blood pressure, and brain blood vessel damage [ 29 ]. Because one dementia subtype often predominates, MD is difficult to identify. As a result, the individuals affected by MD are rarely treated and miss out on potentially life-changing medicines. MD can cause symptoms to begin earlier than the actual diagnosis of the disease and spread swiftly to affect the most areas of the brain [ 30 ].
Recently, numerous automated methods have been developed based on machine learning for early the prediction of different diseases [ 31 , 32 , 33 , 34 , 35 , 36 , 37 , 38 , 39 , 40 , 41 , 42 , 43 , 44 , 45 , 46 , 47 , 48 ]. This systematic literature review (SLR) presented hereby, investigates machine learning-based automated diagnostic systems that are designed and developed by scientists to predict dementia and its subtypes, such as AD, VaD, LBD, FTD and MD. We used the Preferred Reporting Items for Systematic Reviews and Meta-Analysis (PRISMA) criteria to conduct this SLR [ 49 , 50 ]. A comprehensive search was conducted to retrieve the research articles that contain ML approaches to predict the development of dementia and its subtypes using three different types of data modalities (images, clinical-variables, voice).
Aim of the study
SLRs are done to synthesize current evidence, to identify gaps in the literature, and to provide the groundwork for future studies [ 51 ]. Previous, SLRs studies have been done on automated diagnostic systems for dementia prediction based on ML approaches, which focused on a single sort of data modality. These SLR investigations did not emphasize the limits of previously published automated approaches for dementia prediction. The SLR presented herein assesses the previously proposed automated diagnostic systems based on deep learning (DL) and ML algorithms for the prediction of dementia and its common subtypes (e.g. AD, VaD, FTD, MD). The aim of this SLR is to analyse and evaluate the performance of automated diagnostic systems for dementia prediction using different data modalities. The main question is decomposed in the following sub-research questions:
What types of ML and DL techniques have been used by researchers to diagnose dementia?
Examine the methods of feature extraction or selection used by the researchers.
Analyze the different performance evaluation measures that are adopted by the researcher to validate the effectiveness of the proposed diagnostic system for demetnia.
Analyze the performance of ML models on various data types.
Identification of weaknesses in previously proposed ML models for dementia prediction.
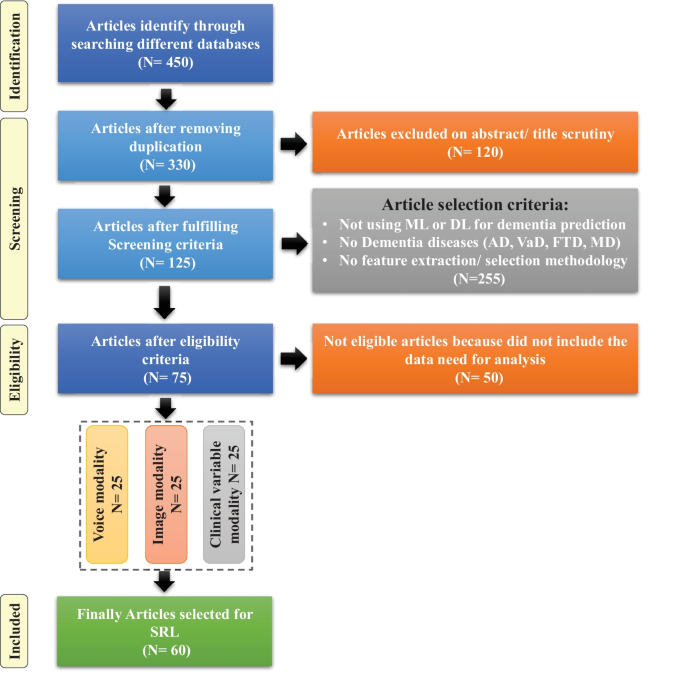
Flow diagram of PRISMA (Preferred Reporting Items for Systematic Reviews and Meta-analyses)
Article selection
For this SLR study, the research articles were selected based on keywords such as ML, DL, dementia and its subtypes (AD, VaD, FTD, and MD). For the collection of research articles, we conducted an electronic search from different online databases such as ScienceDirect, PubMed, IEEE Xplore Digital Library, Springer, Hindawi, and PLOs, which helped to gather 450 research studies on the specific topic. After reviewing the title and abstract in each study, 120 publications were found to be ineligible for processing, while 330 articles were selected for further processing. Following the deduplication of data, 125 full-text publications were retrieved for further processing after the screening phase of the article selection, with 205 of them being eliminated due to not satisfying the article selection criteria of the screening phase. Finally, 50 research articles were eliminated due to not fulfilling the eligibility criteria for article selection. The final set of selected papers consisted of 75 research papers, among these final selected articles, each of the data modalities (image, clinical-variables, voice) contained 25 papers. After rerunning the database searches in May 2022, no further suitable research article was found for the selection. Figure 3 presents the workflow for article selection, which includes the four PRISMA guidelines-recommended steps such as identification, screening, eligibility, and inclusion [ 49 , 50 ]. In recent years, ML scientists have shown a strong interest in designing and developing ML-based automated diagnostic systems for dementia prediction. Therefore, the number of research articles in this research area has been increased and it can be depicted from Fig. 4 where research articles are published years wise with regarding data modality. The publications utilized in this study were selected based on the following criteria:
Studies that present automated diagnostic systems for dementia and its common subtypes (AD,VaD, FTD, MD).
Studies published between 2011 and 2022.
Studies employing ML approaches for dementia diagnosis.
Studies which have utilized several data modalities.
Studies published in the English language.
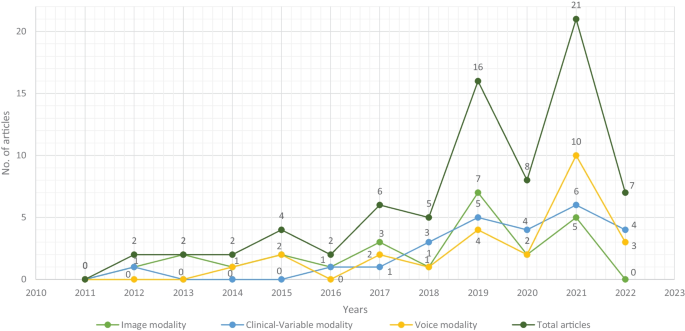
Selected research articles which are published from 2011 to 2022 regarding data modality
Machine learning for dementia
Over the years, the increasing use and availability of medical equipment has resulted in a massive collection of electronic health records (EHR) that might be utilized to identify dementia using developing technologies such as ML and DL [ 52 ]. These EHRs are one of the most widely available and used clinical datasets. They are a crucial component of contemporary healthcare delivery, providing rapid access to accurate, up-to-date, comprehensive patient information while also assisting with precise diagnosis and coordinated, efficient care [ 53 ]. Laboratory tests, vital signs, drugs, and other therapies, as well as comorbidities, can be used to identify the people at risk of dementia using the EHRs’ data [ 54 ]. In some situations, patients may also be subjected to costly and invasive treatments such as neuroimaging scans i.e., magnetic resonance imaging (MRI) and position emission tomography (PET)) and cerebrospinal fluid (CSF) collection for biomarker testing [ 55 , 56 , 57 ]. These tests’ findings may also be found in the EHR. According to researchers, such longitudinal clinical EHR data can be used to track the advancement of AD dementia over time [ 58 ]. Recently, several automated diagnostic systems for different diseases, such as Parkinson’s disease [ 59 ], hepatitis [ 47 ], carcinoma [ 41 ], and heart failure [ 60 , 61 , 62 ] prediction have been designed by employing ML and DL techniques. Inspired by this fact, the unmet demand for dementia knowledge, along with the availability of relevant huge datasets, has motivated scientists to investigate the utility of artificial intelligence (AI), which is gaining a prominent role in the area of healthcare innovation [ 63 ]. ML, a subset of AI, can model the relationship between input quantities and clinical outcomes, identify hidden patterns in enormous volumes of data, and draw conclusions or make decisions that help with more accurate clinical decision-making [ 51 ]. However, computational hypotheses generated by ML models must still be confirmed by subject matter experts in order to achieve enough precision for clinical decision-making [ 64 ].
In this SLR, we have included studies that have used ML predictive models (supervised and unsupervised) for dementia prediction and excluded studies that have used statistical methods for cohort summarization and hypothesis testing (e.g., odds ratio, chi-square distribution, Kruskal-Wallis test, and Kappa-Cohen test). Furthermore, we have referenced the data modality-based study [ 65 ] for this literature review, where we have categorized the three data modality types such as image, clinical-variable and voice. Thus, we have studied each modality-based automated diagnostic system for dementia prediction that has been proposed in the past using ML and DL.
This section explains the datasets that were used in the selected research papers for experiments and performance evaluation of the proposed automated diagnostic systems designed by the researchers using ML algorithms for dementia and its subtypes. A total of 61 datasets were studied from the selected research articles. These datasets are compiled from a wide range of organizations and hospitals throughout the world. Only a few datasets are openly available to the public, while others are compiled by researchers from various hospitals and healthcare institutes. We have only included datasets that have been used to diagnose AD, VaD, FTD, MD, and LBD using ML and DL techniques. On the basis of data modality, we have categorised the dataset into three types: images, clinical_variables and voice datasets. The datasets differ in terms of the number of variables (features) and samples. As a result, we examined each modality of the dataset one by one.
Image modality based datasets
There are several image datasets based on brain imaging, such as magnetic resonance imaging (MRI), collected by the researchers for the diagnosis of dementia. From the Table 1 , it can be depicted that Open Access Series of Imaging Studies (OASIS) and Alzheimer’s Disease Neuroimaging Initiative (ADNI) datasets are mostly used by the researchers for the experimental purpose. OASIS aims to make neuroimaging datasets available to the scientific community for free. By gathering and openly disseminating this multimodal dataset produced by the Knight ADRC and its related researchers, they had used different samples and variables of the datasets in their research work. ADNI researchers acquire, validate, and use data such as MRI, PET imaging, genetics, cognitive assessments, CSF, and blood biomarkers as disease predictors. The ADNI website contains research information and data from the North American ADNI project, which includes Alzheimer’s disease patients, people with mild cognitive impairment, and older controls. Table 1 provides us with the following information: dataset_id, dataset name, number of samples in the particular dataset, variables in the dataset, and finally, the type of dementia.
Clinical-variables modality based datasets
Throughout the course of time, the growing usage and availability of medical devices have resulted in an overwhelming collection of clinical EHR data. Furthermore, the patient’s medical history consists of medical tests and clinical records that can be used for the prediction of diseases. Thus, the importance of clinical data emerges as a vital tool for proactive management of disease. The dataset based on clinical variables for dementia consists of medical tests that are used by doctors to check the dementia status in patients, such as the Mini Mental Status Exam (MMSE), the Montreal Cognitive Assessment (MoCA), the Telephone Interview for Cognitive Status (TICS), and the Brief Interview for Mental Status (BIMS). Clinical-variables based datasets consist of information about these medical tests along with patient personal information, i.e., age, sex, and marital status. Hereby, Table 2 provides the information regarding clinical-variables modality-based datasets that are used by the researchers for the design and development of automated diagnostic systems for dementia patients based on ML. Table 2 presents the dataset_id, dataset name, number of samples in the particular dataset, variables in the dataset, and finally the type of dementia.
Voice modality based datasets
Speech analysis is a useful technique for clinical linguists in detecting various types of neurodegenerative disorders affecting the language processing areas. Individuals suffering from Parkinson’s disease (PD, deterioration of voice quality, unstable pitch), Alzheimer’s disease (AD, monotonous pitch), and the non-fluent form of Primary Progressive Aphasia (PPA-NF, hesitant, non-fluent speech) may experience difficulties with prosody, fluency, and voice quality. Besides imaging and clinical-variables data, the researchers employed voice recording data to identify dementia using ML and DL algorithms. The data collection process for voice data varies from dataset to dataset, for example, in a few datasets, patients were requested to answer a prepared set of questions (interview) in a specific time interval. In a few datasets, selected neuropsychological tests were carried out, the description of each neuropsychological test was played and was followed by an answering window. Table 3 presents the dataset_id, dataset name, number of samples in the particular dataset, variables in the dataset, and finally the subtype of dementia.
Data sharing challenges
In this digital era, public health decision-making has grown progressively complicated, and the utilization of data has become critical [ 66 ]. Data are employed at the local level to observe public health and target interventions; at the national scale for resource allocation, prioritization, and planning; and at the global scale for disease burden estimates, progress in health and development measurement, and the containment of evolving global health threats [ 67 , 68 ]. Van Panhuis et al. have adequately described the challenges to exchanging health data [ 69 ]. Based on our initial analysis, we built on this taxonomy to identify the hurdles related to data sharing in global public health, and we have highlighted how they may apply to each typology as given below.
Lack of complete data, lost data, restrictive as well as conflicting data formats, a lack of metadata and standards, a lack of interoperability of datasets (e.g., structure or “language”), and a lack of appropriate analytic solutions are examples of technical barriers encountered by health information management systems.
Individuals and organizations face motivational challenges when it comes to sharing data. These impediments include a lack of incentives, opportunity costs, apprehension about criticism, and disagreements over data usage and access.
The potential and present costs of sharing data are both economic hurdles.
Political obstacles are those that are built into the norms of local health governance and often emerge as regulations and guidelines. They can also entail trust and ownership difficulties.
Legal issues that arise as a result of data collection, analysis, and usage include questions regarding who owns or controls the data, transparency, informed permission, security, privacy, copyright, human rights, damage, and stigma.
Ethical constraints include a lack of perceived reciprocity (i.e., the other side will not disclose data) and proportionality (i.e., deciding not to share data based on an assessment of the risks and benefits). An overall concern is that frameworks, rules, and regulations have not kept up with technological changes that are transforming how data is collected, analyzed, shared, and used.
ML based diagnostic models for dementia: Image modality
In recent years, researchers have designed many ML and DL algorithms for the detection of dementia and its subtypes using MRI images of the brain. For example, Dashtipour et al. [ 70 ] proposed a ML based method for the prediction of Alzheimer’s disease. In their proposed model, they used DL techniques to extract the features from brain images, and for classification purposes, they deployed SVM and bidirectional long short-term memory (BiLSTM). Through their proposed model, they had reported the classification accuracy of 91.28%. Moreover, for early detection of the AD, a DL based approach was proposed by Helaly et al. In their proposed work, they employed convolutional neural networks (CNN). The Alzheimer’s disease spectrum is divided into four phases. Furthermore, different binary medical image classifications were used for each two-pair class of Alzheimer’s disease stages. Two approaches were used to categorize medical images and diagnose Alzheimer’s disease. The first technique employs basic CNN architectures based on 2D and 3D convolution to cope with 2D and 3D structural brain images from the ADNI dataset. They had achieved highly promising accuracies for 2D and 3D multi-class AD stage classification of 93.61% and 95.17%, respectively. The VGG19 pre-trained model had been fine-tuned and obtained an accuracy of 97% for multi-class AD stage classification [ 71 ]. Vandenberghe et al. had proposed a method for binary classification of 18F-flutemetramol PET using ML techniques for AD and mild cognitive impairment (MCI). They had tested whether support vector machines (SVM), a supervised ML technique, can duplicate the assignments made by blindfolded visual readers, as well as which image components had the highest diagnostic value according to SVM and how 18F-fluoromethylamol-based SVM classification compares to structural MRI-based SVM classification in the same cases. Their F-flutemetamol based classifier was able to replicate the assignments obtained by visual read with 100% accuracy [ 72 ]. Odusami et al. proposed a novel method for the detection of early-stage dementia from functional brain changes in MRI using a fine-tuned ResNet-18 network. Their research work presents a DL based technique for predicting MCI, early MCI, late MCI, and Alzheimer’s disease (AD). The ADNI fMRI dataset was used for analysis and consisted of 138 participants. On EMCI vs. AD, LMCI vs. AD, and MCI vs. AD, the fine-tuned ResNet18 network obtained classification accuracy of 99.99%, 99.95%, and 99.95%, respectively [ 73 ]. Zheng et al. had presented a ML based framework for differential diagnosis between VaD and AD using structural MRI features. The least absolute shrinkage and selection operator (LASSO) was then used to build a feature set that was fed into SVM for classification. To ensure unbiased evaluation of model performance, a comparative analysis of classification models was conducted using different ML algorithms to discover which one had better performance in the differential diagnosis between VaD and AD. The diagnostic performance of the classification models was evaluated using quantitative parameters derived from the receiver operating characteristic curve (ROC). The experimental finding had shown that the SVM with RBF performed well for the differential diagnosis of VaD and AD, with sensitivity (SEN), specificity (SPE), and accuracy (ACC) values of 82.65%, 87.17%, and 84.35%, respectively (AUC = 86.10–95%, CI = 0.820–0.902) [ 74 ]. Basheer et al. [ 75 ] had presented an innovative technique by making improvements in capsule network design for the best prediction outcomes. The study used the OASIS dataset with dimensions (373 X 15) to categorize the labels as demented or non-demented. To make the model swifter and more accurate, several optimization functions were performed on the variables, as well as the feature selection procedure. The claims were confirmed by demonstrating the correlation accuracy at various iterations and layers with an allowable accuracy of 92.39%. L. K. Leong and A. A. Abdullah had proposed a method for the prediction of AD based on ML techniques with the Boruta algorithm as a feature selection method. According to the Boruta algorithm, Random Forest Grid Search Cross Validation (RF GSCV) outperformed other 12 ML models, including conventional and fine-tuned models, with 94.39% accuracy, 88.24% sensitivity, 100.00% specificity, and 94.44% AUC even for the small OASIS-2 longitudinal MRI dataset [ 76 ]. Battineni et al. had presented a SVM based ML model for the prediction of dementia. Their proposed model had achieved an accuracy and precision of 68.75% and 64.18% using the OASIS-2 dataset [ 77 ]. Mathotaarachchi et al. had analyzed the amyloid imaging using ML approaches for the detection of dementia. To overcome the inherent unfavorable and imbalance proportions between persons with stable and progressing moderate cognitive impairment in a short observation period. The innovative method had achieved 84.00% accuracy and an AUC of 91.00% for the ROC [ 78 ]. Aruna and Chitra had presented a ML approach for the identification of dementia from MRI images, where they had deployed Independent Component Analysis (ICA) to extract the features from the images, and for classification purposes, SVM with different kernels is used. Through their proposed method, they had obtained an accuracy of 90.24% [ 79 ] (Fig. 5 ).
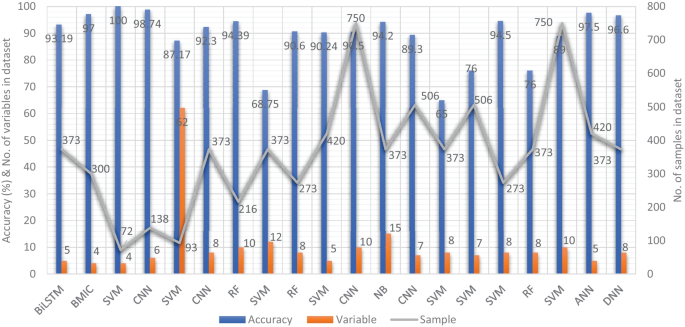
Accuracy comparison of different ML models based on image modality
Supervised ML techniques and CNNs were examined by Herzog and Magoulas. They had achieved the accuracy of 92.5% and 75.0% for NC vs EMCI, 93.0% and 90.5% for NC vs. AD, respectively [ 80 ]. Battineni et al. had comprehensive applied ML model on MRI to predict Alzheimer’s disease (AD) in older subjects, and they had proposed two ML models for AD detection. In the first trial, manual feature selection was utilized for model training, and ANN produced the highest AUC of 81.20% by ROC. The NB had earned the greatest AUC of 94.20% by ROC in the second trial, which included wrapping approaches for the automated feature selection procedure [ 81 ]. Ma et al. had conducted a study where they compared feature-engineered and non-feature-engineered ML methods for blinded clinical evaluation for dementia of Alzheimer’s type classification using FDG-PET. The highest accuracy of 84.20% was obtained through CNN’s [ 82 ]. Bidani et al. had presented a novel approach in the field of DL that combines both the deep convolutional neural network (DCNN) model and the transfer learning model to detect and classify dementia. When the features were retrieved, the dementia detection and classification strategy from brain MRI images using the DCNN model provided an improved classification accuracy of 81.94%. The transfer learning model, on the other hand, had achieved an accuracy of 68.13% [ 83 ].
Moscoso et al. had designed a predictive model for the prediction of Alzheimer’s disease using MRI images. Their proposed model had obtained the highest accuracy of 84.00% [ 84 ]. Khan and Zubair had presented an improved multi-modal based ML approach for the prognosis of AD. Their proposed model had a five-stage ML pipeline, where each stage was further categorized into different sub-levels. Their proposed model had reported the highest accuracy of 86.84% using RF [ 85 ]. Mohammed et al. had evaluated the two CNN models (AlexNet and ResNet-50) and hybrid DL/ML approaches (AlexNet+SVM and ResNet-50+SVM) for AD diagnosis using the OASIS dataset. They had found that RF algorithm had attained an overall accuracy of 94%, as well as precision, recall, and F1 scores of 93%, 98%, and 96%, respectively [ 86 ]. Salvatore et al. had developed a ML method for early AD diagnosis using magnetic resonance imaging indicators. In their proposed ML model, they used PCA for extracting features from the images and SVM for the classification of dementia. They had achieved a classification accuracy of 76% using a 20-fold cross validation scheme [ 87 ]. Katako et al. had identified the AD related FDGPET pattern that is also found in LBD and Parkinson’s disease dementia using ML approaches. They studied different ML algorithms, but SVM with an iterative single data algorithm produced the best performance, i.e., sensitivity 84.00%, specificity 95.00% through 10-fold cross-validation [ 88 ]. Gray et al. had presented a system in which RF proximities were utilized to learn a low-dimensional manifold from labelled training data and then infer the clinical labels of test data that translated to this space. Their proposed model, voxel-based (FDG-PET), obtained an accuracy of 87.9% using ten-fold cross-validation [ 89 ]. Table 4 provides the overall performance evaluation of the ML models that were presented by the researchers for the prediction of dementia and its subtypes by using image data as a modality.
ML based diagnostic models for dementia: Clinical-variable modality
Aside from image-based ML techniques for dementia prediction, several research studies have utilized clinical-variable data with ML algorithms to predict dementia and its subtypes. For instance, Chiu et al. had designed a screening instrument to detect MCI and dementia using ML techniques. They had developed a questionnaire to assist neurologists and neuropsychologists in the screening of MCI and dementia. The contribution of 45 items that matched the patient’s replies to questions was ranked using feature selection through information gain (IG). Among the 45 items, 12 were ranked the highest in feature selection. The ROC analysis showed that AUC in test group was 94.00% [ 96 ]. Stamate et al. had developed a framework for the prediction of MCI and dementia. Their proposed framework was based on the ReliefF approach paired with statistical permutation tests for feature selection, model training, tweaking, and testing using ML algorithms such as RF, SVM, Gaussian Processes, Stochastic Gradient Boosting, and eXtreme Gradient Boosting. The stability of model performances was studied using computationally expensive Monte Carlo simulations, and the results of their proposed framework were given as for dementia detection, the accuracy was 88.00%, sensitivity was 93.00%, and the specificity was 94.00%, whereas moderate cognitive impairment had a sensitivity of 86.00% and a specificity of 90% [ 97 ]. Stamate et al. developed a system for detecting dementia subtypes (AD) in blood utilizing DL and other supervised ML approaches such as RF and extreme gradient boosting. The AUC for the proposed DL method was 85% (0.80–0.89), for XGBoost it was 88% (0.86–0.89), and for RF it was 85% (0.83–0.87). In comparison, CSF measurements of amyloid, p-tau, and t-tau (together with age and gender) gave AUC values of 78%, 83%, and 87%, respectively, by using the XGBoost [ 98 ]. Bansal1 et al. had performed the comparative analysis of the different ML methods for the detection of dementia using clinical-variables. In their experiments, they exploited the performance of four ML models, such as J48, NB, RF, and multilayer perceptrons. From the results of experiments, they had concluded that j48 outperformed the rest of the ML models for the detection of dementia [ 99 ]. Nori et al. had experimented the lasso algorithm on a big dataset of patient and identify the 50 variables by ML model with an AUC of 69.30% [ 100 ]. Alam et al. [ 101 ]used signal processing on wearable sensor data streams (e.g., electrodermal activity (EDA), photoplethysmogram (PPG), and accelerometer (ACC)) and machine learning techniques to measure cognitive deficits and their relationship with functional health deterioration.
Gurevich et al. had used SVM and neuropsychological test for the classification of AD from other causes of cognitive impairment. The highest classification accuracy they had achieved through their proposed method was 89.00% [ 102 ]. Karaglani et al. had proposed a ML based automated diagnosis system for AD by using blood-based biosignatures. In their proposed method, they used mRNA-based statistically equivalent signatures for feature ranking and a RF model for classification. Their proposed automated diagnosis system had reported the accuracy of 84.60% using RF [ 103 ]. Ryzhikova et al. had analyzed cerebrospinal fluid for the diagnosis of AD by using ML algorithms. For classification purposes, artificial neural networks (ANN) and SVM discriminant analysis (SVM-DA) statistical methods were applied, with the best findings allowing for the distinguishing of AD and HC participants with 84.00% sensitivity and specificity. The proposed classification models have a high discriminative power, implying that the technique has a lot of potential for AD diagnosis [ 104 ]. Cho and Chen had designed a double layer dementia diagnosis system based on ML where fuzzy cognitive maps (FCMs) and probability neural networks (PNNs) were used to provide initial diagnoses at the base layer, and Bayesian networks (BNs) were used to provide final diagnoses at the top layer. Diagnosis results, “proposed treatment,” and “no treatment required” might be used to provide medical institutions with self-testing or secondary dementia diagnosis. The highest accuracy reported by their proposed system was 83.00% [ 105 ]. Facal et al. had studied the role of cognitive reserve in the conversion from MCI to dementia using ML. Nine ML classification algorithms were tried in their study, and seven relevant performance parameters were generated to assess the prediction accuracy for converted and non-converted individuals. The use of ML algorithms on socio-demographic, basic health, and CR proxy data allowed for the prediction of dementia conversion. The Gradient Boosting Classifier (ACC = 0.93; F1 = 0.86 and Cohen’s kappa = 0.82) and RF Classifier (ACC = 92%; F1 = 0.79 and Cohen’s kappa = 0.71) performed the best [ 106 ]. Jin et al. had proposed automatic classification of dementia from learning of clinical consensus diagnosis in India using ML techniques. All viable ML models exhibited remarkable discriminative skills (AUC >90%) as well as comparable accuracy and specificity (both around 95%). The SVM model beat other ML models by obtaining the highest sensitivity (0.81), F1 score (0.72), kappa (.70, showing strong agreement), and accuracy (second highest) (0.65). As a consequence, the SVM was chosen as the best model in their research work [ 107 ]. James et al. had evaluated the performance of ML algorithms for predicting the progression of dementia in memory clinic patients. According to their findings, ML algorithms outperformed humans in predicting incident all-cause dementia within two years. Using all 258 variables, the gradient-boosted trees approach had an overall accuracy of 92% , sensitivity of 0.45, specificity of 0.97, and an AUC of 0.92. Analysis of variable significance had indicated that just 6 variables were necessary for ML algorithms to attain an accuracy of 91% and an AUC of at least 89.00% [ 108 ]. Bougea et al. had investigated the effectiveness of logistic regression (LR), K-nearest neighbours (K-NNs), SVM, the Naive Bayes classifier, and the Ensemble Model to correctly predict PDD or DLB. The K-NN classification model exhibited an overall accuracy of 91.2% based on 15 top clinical and cognitive scores, with 96.42% sensitivity and 81% specificity in distinguishing between DLB and PDD. Based on the 15 best characteristics, the binomial logistic regression classification model had attained an accuracy of 87.5%, with 93.93% sensitivity and 87% specificity. Based on the 15 best characteristics, the SVM classification model had achieved an accuracy of 84.6% of overall instances, 90.62% sensitivity, and 78.58% specificity. A model based on NB classification obtained an accuracy of 82.05%, sensitivity of 93.10%, and a specificity of 74.41%. Finally, an ensemble model, which was constructed by combining the separate ones, attained 89.74% accuracy, 93.75% sensitivity, and 85.73% specificity [ 109 ] (Fig. 6 ).
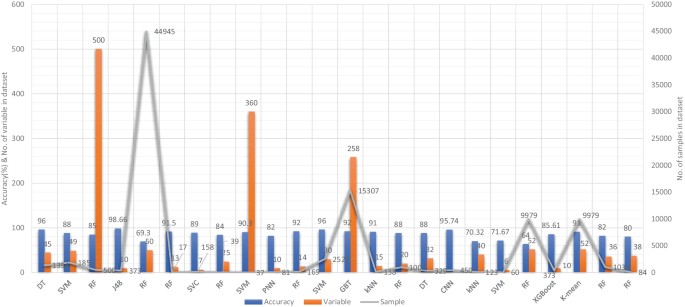
Accuracy comparison of different ML models based on clinical-variable modality
Salem et al. had presented a regression-based ML model for the prediction of dementia. In their proposed method, they had investigated ML approaches for unbalanced learning. In their suggested supervised ML approach, they started by intentionally oversampling the minority class and undersampling the majority class, in order to reduce the bias of the ML model to be trained on the dataset. Furthermore, they had deployed cost-sensitive strategies to penalize the ML models when an instance was misclassified in the minority class. According to their findings, the balanced RF was the most resilient probabilistic model (with just 20 features/variables) with an F1 score of 0.82, a G-Mean of 0.88, and an AUC of 0.88 using ROC. With a F1-score of 0.74 and an AUC of 0.80 by ROC, the calibrated-weighted SVM was their top classification model for the same number of features [ 110 ]. Gutierrez et al. had designed an automated diagnosis system for the detection of AD and FTD by using feature engineering and genetic algorithms. Their proposed system had obtained the accuracy of 84% [ 111 ]. Mirzaei and Adeli had analyzed the state-of-the-art ML techniques used for the detection and classification of AD [ 112 ]. Hsiu et al. had studied ML algorithms for early identification of cognitive impairment. Their proposed model had obtained the accuracy of 70.32% by threefold cross-validation scheme [ 113 ]. Several classification models were constructed using various ML and feature selection methodologies to automate MCI detection using gait biomarkers. They had demonstrated, however, that dual-task walking differentiated between MCI and CN individuals. The ML model used for MCI pre-screening based on inertial sensor-derived gait biomarkers achieved 71.67% accuracy and 83.33% sensitivity, respectively, as reported by Shahzad et al. [ 114 ]. Hane et al. investigated the use of deidentified clinical notes acquired from multiple hospital systems over a 10-year period to enhance retrospective ML models predicting the risk of developing AD. The AUC improved from 85.00% to 94.00% by utilizing clinical notes, and the positive predictive value (PPV) rose from 45.07% (25,245/56,018) to 68.32% (14,153/20,717) in the model at the beginning of disease [ 115 ]. Table 5 provides the overall performance evaluation of the ML models that were presented by the researchers for the prediction of dementia and its subtypes by using clinical-variable data as a modality.
ML based diagnostic models for dementia: Voice modality
Similar to the image and clinical-variable modalities, researchers had also developed automated diagnostic systems based on voice data for the prediction of dementia. Hereby, we have reviewed the research work done by the scientists in detail. For example, Chlasta and Wolk had worked on the computer-based automated screening of dementia patients by spontaneous speech analysis using DL and ML techniques. In their work, they used neural networks to extract the features from the voice data; the extracted features were then fed into a linear SVM for classification purposes. Their SVM model had obtained the accuracy of 59.1% while CNN based ML model had reported the accuracy of 63.6% [ 121 ]. Chien et al. had presented an ML model for the assessment of AD using speech data. Their suggested model included a feature sequence that was used to extract the features from the raw audio data, as well as a recurrent neural network (RNN) for classification. Their proposed ML model had reported an accuracy of 83.80% based on the ROC curve [ 122 ]. Shimoda et al. had designed an ML model that identified the risk of dementia based on the voice feature in telephone conversations. Extreme gradient boosting (XGBoost), RF, and LR based ML models were used, with each audio file serving as one observation. The predictive performance of the constructed ML models was tested by characterizing the ROC curve and determining the AUC, sensitivity, and specificity [ 123 ]. Nishikawa et al. had developed an ensemble discriminating system based on a classifier with statistical acoustic characteristics and a neural network of transformer models, with an F1-score of 90.70% [ 124 ]. Liu et al. had introduced a new technique for recognizing Alzheimer’s disease that used spectrogram features derived from speech data, which aided families in comprehending the illness development of patients at an earlier stage, allowing them to take preventive measures. They used ML techniques to diagnose AD using speech data collected from older adults who displayed the attributes described in the speech. Their proposed method had obtained the maximum accuracy of 84.40% based on LogisticRegressionCV [ 125 ]. Searle et al. had created a ML model to assess spontaneous speech, which might potentially give an efficient diagnostic tool for earlier AD detection. Their suggested model was a fundamental Term Frequency-Inverse Document Frequency (TF-IDF) vectorizer as input into an SVM model, and the top performing models were a pre-trained transformer-based model ’DistilBERT’ when used as an embedding layer into simple linear models. The proposed model had obtained the highest accuracy of 82.00% [ 126 ]. Zhu et al. had suggested an ML model that employed the speech pause as an effective biomarker in dementia detection, with the purpose of reducing the detection, model’s confidence levels by adding perturbation to the speech pauses of the testing samples. They next investigated the impact of the perturbation in training data on the detection model using an adversarial training technique. The proposed model had achieved an accuracy of 84.00% [ 127 ]. Ossewaarde et al. had proposed ML model based on SVM for the classification of spontaneous speech of individuals with dementia based on automatic prosody analysis. Their findings suggest that the classifier can distinguish some dementia types (PPA-NF, AD), but not others (PD) [ 128 ]. Xue et al. had developed an ML model based on DL for the detection of dementia by using voice recordings. In their ML model, long short-term memory (LSTM) network and the convolutional neural network (CNN) utilized audio recordings to categorize whether the recording contained a participant with either NC or only DE and to discriminate between recordings belonging to those with DE and those without DE (i.e., NDE (NC+MCI)) [ 129 ]. Weiner et al. had presented two pipelines of feature extraction for dementia detection: the manual pipeline used manual transcriptions, while the fully automatic pipeline used transcriptions created by automatic speech recognition (ASR). The acoustic and linguistic features that they had extracted need no language specific tools other than the ASR system. Using these two different feature extraction pipelines, they had automatically detect dementia [ 130 ] (Fig. 7 ).
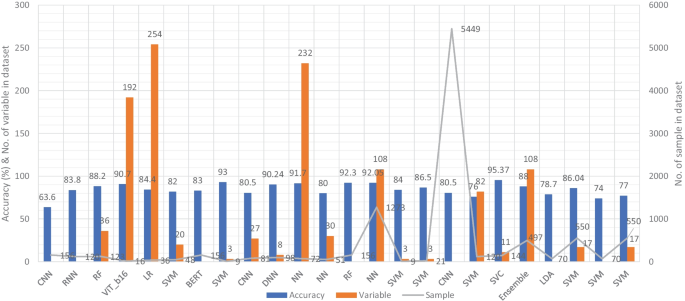
Accuracy comparison of different ML models based on voice modality
Furthermore, Sadeghian et al. had presented the empirical evidence that a combination of acoustic features from speech, linguistic features were extracted from an automatically determined transcription of the speech including punctuation, and results of a mini mental state exam (MMSE) had achieved strong discrimination between subjects with a probable AD versus matched normal controls [ 131 ]. Khodabakhsh et al. had evaluated the linguistic and prosodic characteristics in Turkish conversational language for the identification of AD. Their research suggested that prosodic characteristics outperformed linguistic features by a wide margin. Three of the prosodic features had helped to achieve a classification accuracy of more than 80%, However, their feature fusion experiments did not improve classification performance any more [ 132 ]. Edwards et al. had analyzed the text data at both the word level and phoneme level, which leads to the best-performing system in combination with audio features. Thus, the proposed system was both multi-modal (audio and text) and multi-scale (word and phoneme levels). Experiments with larger neural language models had not resulted in improvement, given the small amount of text data available [ 133 ]. Kumar et al. had identified speech features relevant in predicting AD based on ML. They had deployed neural network for the classification and obtained the accuracy of 92.05% [ 134 ]. Ossewaarde et al. had built ML model based on SVM for the classification from spontaneous speech of individuals with dementia by using automatic prosody [ 128 ]. Luz et al. had developed an ML approach for analyzing patient speech in dialogue for dementia identification. They had designed a prediction model, and the suggested strategy leveraged additive logistic regression (ML boosting method) on content-free data gathered through dialogical interaction. Their proposed model obtained the accuracy of 86.50% [ 135 ]. Sysed et al. had designed a multimodal system that identified linguistic and paralinguistic traits of dementia using an automated screening tool. Their proposed system had used bag-of-deep-feature for feature selection and ensemble model for classification [ 136 ]. Moreover, Sarawgi et al. had used multimodal inductive transfer learning for AD detection and severity. Their proposed system further achieved state-of-the-art AD classification accuracy of 88.0% when evaluated on the full benchmark DementiaBank Pitt database. Table 6 provides the overall performance evaluation of the ML models that were presented by the researchers for the prediction of dementia and its subtypes by using voice-modality data.
In this SLR, we examined the research work that employed ML and DL algorithms to analyze clinical data in order to identify variables that might help predict dementia. We studied 75 research articles that were published in the last 10 years that used image, clinical-variable, and voice data to predict dementia and its subtypes. Nowadays, the healthcare industry creates a vast quantity of data on patients’ health; this data is used by researchers to enhance individual health by utilizing developing technologies such as ML and DL. As a result, researchers can not only distinguish dementia patients from healthy people with high accuracy, but also forecast the disease progression of MCI patients.
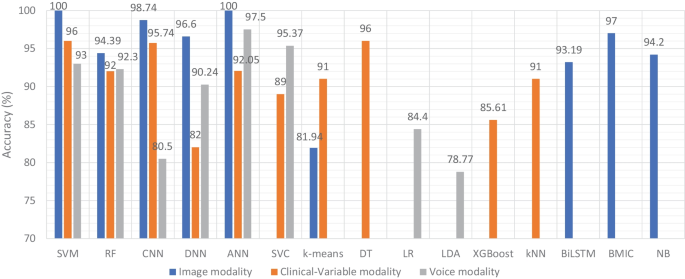
Accuracy comparison of ML models based on data modality
Therefore, researchers have expressed a strong interest in designing and developing automated diagnostic systems based on ML and DL techniques. As seen in Fig. 4 ., there has been an exponential increase in the number of such research publications that use ML algorithms for dementia prediction and detection in the previous four years. We investigated the selected papers using significant performance assessment criteria for ML and DL approaches such as data attributes, computational methodologies, and study emphasis. In this SLR, we have uncovered research gaps in the present literature as well as anticipated future research opportunities. Additionally, in Fig. 8 model comparison, we examined the performance of multiple ML algorithms for dementia prediction based on three types of data modalities: image, clinical-variable, and voice. The accuracy gained by image-based ML algorithms is higher when compared to clinical-variable and voice modalities, as shown in Fig. 8 model comparison. Moreover, the researchers’ suggested SVM, RF, and ANN-based ML techniques outperformed the rest of the ML algorithms in terms of performance. According to Fig. 8 model comparison, voice modality-based ML models show worse accuracy when compared to image and clinical-variable modality data. As a result, there is still a performance gap for researchers to close in order to improve the performance of ML algorithms for the prediction of dementia using voice data. Hence, researchers have shown a strong interest in the creation of automated diagnosis systems for dementia prediction utilizing speech data and ML algorithms, as illustrated in Fig. 4 .
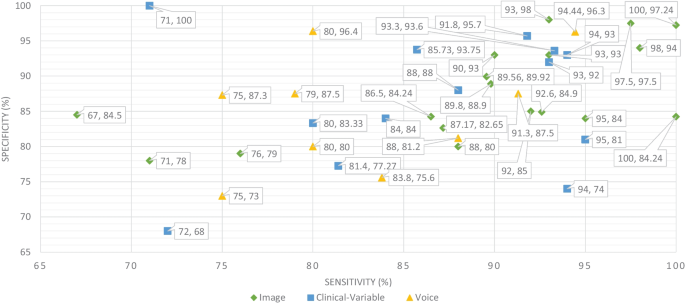
Sensitivity and specificity comparison of ML based on modality
The ML and DL models are likely prone to problems such as poor quality of data, poor selection of ML model, Bias Variance tradeoff and training too complex models. Thus, scientists have developed various evaluation metrics (i.e., ROC, AUC, MCC, F1-score, K-fold) and methods to avoid these problems. The data is a crucial element in ML because ML models work only with numeric data; therefore, poor data quality results in lower performance of ML models. Moreover, imbalance classes in the dataset also cause the bias results from the ML models. Thus, this problem can be overcome by oversampling or undersampling the training data. There are different techniques that are used by the AI engineers for oversampling, such as random oversampling and the synthetic minority oversampling technique (SMOTE). To evaluate the bias researchers’ work, use sensitivity and specificity as an evaluation metric to measure the bias of the ML model. Higher values of sensitivity and specificity means model is free from the biasness while having either one parameter value higher and other one is lower means there is biasness exist. Thus, we have also studied the sensitivity and specificity, along with the accuracy, of the previously proposed ML models for dementia prediction. Figure 9 Comparison provides a brief description of the sensitivity and specificity of the ML models for the detection of dementia based on different data modalities. From Fig. 9 , we can observe that ML models have higher values for sensitivity and specificity when using image data as compared to clinical-variable and voice modality data. In comparison to accuracy from Fig. 8 to sensitivity and specificity from Fig. 9 , we have noted that the results obtained from image based modality are more reliable and precise using ML and DL algorithms in spite of clinical and voice modality.
Furthermore, the correlation between sensitivity and specificity would help us understand the efficacy of the ML models, which are designed for automated disease prediction. The mathematical terms “sensitivity” and “specificity” indicate the accuracy of a test that reports the presence or absence of a disease. Individuals who meet the requirement are labelled “positive,” while those who do not are considered “negative”. The chance of a positive test, conditioned on being actually positive, is referred to as sensitivity (the true positive rate), while specificity (true negative rate) is the likelihood of a negative test if it is actually negative. Sensitivity and specificity are inversely proportional, which means that as sensitivity rises, specificity falls, and vice versa. Mathematically, sensitivity and specificity are given as:
On the other hand, accuracy is a ratio of number of correct assessments / number of all assessments. The proportion of genuine positive outcomes (both true positive and true negative) in the selected population is represented by the numerical value of accuracy. The test result is accurate 99% of the time, whether positive or negative. For the most part, this is right. However, it is worth noting that the equation of accuracy means that even if both sensitivity and specificity are high, say 99%, this does not imply that the test’s accuracy is also high. In addition to sensitivity and specificity, accuracy is determined by the prevalence of the illness in the target population. A diagnosis for a rare ailment in the target group may have high sensitivity and specificity but low accuracy. However, for a balanced dataset, ML models with higher sensitivity and specificity result in higher accuracy. Hence, accuracy must be interpreted carefully. The mathematical formula for accuracy is given as:
where, TP stands for the number of true positives, FP stands for the number of false positives, TN stands for the true negative, and FN stands for the false negative.
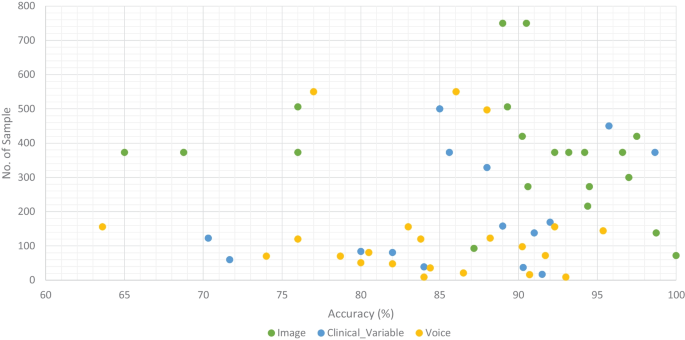
Accuracy comparison of ML models along with number of sample in the dataset based on data modality
We classified all datasets that were used by researchers to test the performance of their proposed ML models for the prediction of dementia (AD, VaD, MCI, and FTD) into three types: image, clinical-variable, and voice. A total of 61 datasets were examined in terms of the number of samples and variables in the datasets. In image modality datasets from the Table 1 , it can be observed that the ADNI dataset has a significant number of samples, which is 750, while the NINDS-AIREN dataset has more variables as compared to the rest of the datasets in the image modality data. Moreover, from the Table 2 of clinical-variable modality datasets, it can be noticed that the ADRD dataset has the highest number of samples (44945) as compared to the rest of the dataset, while the Raman spectral dataset has the highest number of variables (366). In the last, Table 3 of voice data modality elaborated the dataset of voice modality where FHS dataset has highest number of samples of 5449 while VBSD dataset had highest variables of 254 as compared to rest of the datasets in voice modality. The type of data and the size of the dataset are two important factors that have a significant influence on the performance of ML models. Thus, we have also studied this factor by comparing the accuracy along with the number of samples in the dataset with respect to data modalities. From Fig. 10 , it can be observed that the majority of the ML models that used image data have higher accuracy along with a higher number of samples in the dataset. There are few ML models that show poor performance when the number of samples in the dataset is large. While, clinical-variable and voice modalities show prominent performance when the number of samples in the dataset is small.
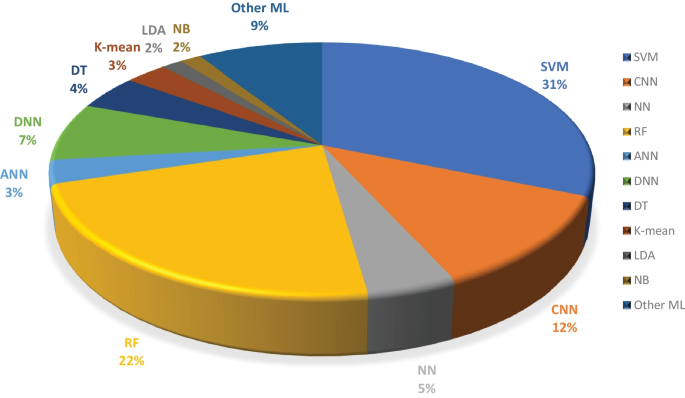
Overall percentage of ML models used in the selected research articles regardless of data modality
Moreover, we examined the effectiveness of ML classifiers utilized by the researchers in their proposed automated diagnostic systems for dementia prediction and classification. According to the selected studies of this SLR, SVM is the most commonly used ML classifier by researchers for the classification of patients and normal subjects using three data modalities (i.e., image, clinical-variable, voice), RF is the second most commonly used ML classifier by researchers, and CNN is the third most commonly used ML classifier by researchers. It can be observed from the Fig. 11 . SVMs are the most powerful tools for the binary classification task, along with RF. From Fig. 8 , we can see that SVM also obtained the highest average accuracy based on three types of data modalities. Hence, this factor also encourages the scientists to employ SVM as a binary classifier for dementia prediction or other disease prediction systems. From Fig. 11 , we can observe the percentage of other ML classifiers that were used by the researchers in selected research articles for the automated diagnosis of dementia.
There are several evaluation metrics that are used for the performance assessment of ML models, such as F1score, AUC, ROC, Matthew’s correlation coefficient (MCC), cross-validation, K-fold, specificity, sensitivity, and accuracy. Each evaluation metric has its own pros and cons. Thus, the selection of appropriate evaluation metrics for the assessment of the ML model is essential to understanding its efficiency and performance. For instance, when data plays a vital role in ML models for decision-making and a dataset has unbalanced classes, it may be possible that results from the ML predictive model might be biassed due to the unbalanced nature of the data in the dataset. Thus, here evaluation metrics help to eliminate the factor of biasness in the results, i.e., the k-fold. The F1-score evolution metric is suitable for the classification of multiple classes in the dataset. while ROC tells us how well the ML model can differentiate binary classes. As a result, AUC and ROC reveal how effectively the probabilities from the positive classes are separated from the probabilities from the negative classes. From Fig. 12 , it can be depicted that cross validation is mostly used in the studies that were selected for this SLR to evaluate the performance of proposed ML models. MCC is the second most used evaluation metric, while ROC is in third place. The proposition of other evaluation metrics used by the researchers to validate the efficiency of their proposed ML models can be observed from Fig. 12 .
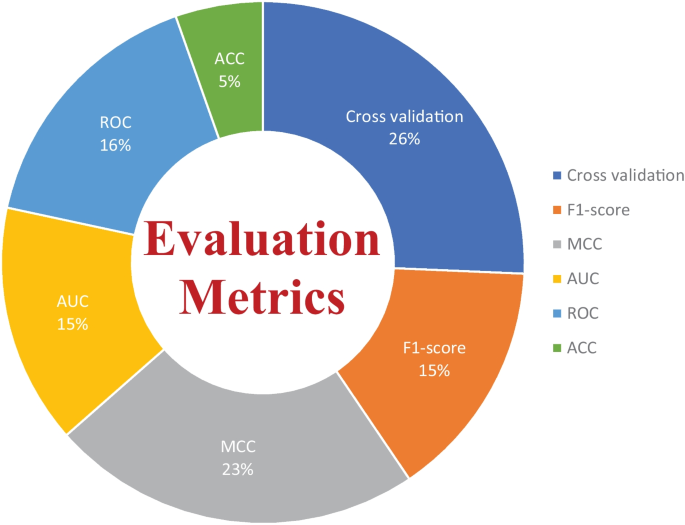
Overall percentage of evaluation metrics of ML models used by the researchers in the selected research articles
Limitations in the previously proposed ML models
ML algorithms have been effectively applied to a broad range of real-world challenges, including banking, cybersecurity, transportation, and robots. They do, however, have fundamental limitations that make them inappropriate for every problem. In the clinical domain, researchers have concentrated on the supervised learning approach, developing various automated diagnostics for AD, MCI, and dementia prediction using supervised machine algorithms. From the Figs. 8 and 11 , It can be noticed that supervised ML classifiers are mostly used by the researchers in the selected past research articles. Because supervised machine learning approaches have various limitations, automated diagnostic methods for dementia prediction based on supervised techniques suffer from some, if not all, of these constraints. In this part, we have examined the drawbacks of supervised ML-based techniques for dementia prediction, which are as follows:
The model overfitting problem affects the performance of ML models. As previously indicated, several researchers have used the k-fold cross-validation approach to evaluate the efficacy of their constructed diagnostic system. However, because of data leaks, it may result in highly biassed findings.
To deal with problem of imbalance classes in the dataset, Researchers and scientists had devised several techniques to eliminate the problem of imbalance classes such as random oversampling example (ROSE), synthetic minority over-sampling technique (SMOTE) and random over sampling (ROS) etc. Unfortunately, in the selected study, the researchers had not considered this factor to deal with the problem of imbalanced classes in the dataset that cause problems of bias.
Supervised ML models require training on a dataset; nevertheless, training on a large quantity of significant data is a hard and time-consuming job, especially for slow learning algorithms like kNN.
For training and testing of the ML models, researchers had used different data partitioning methods, which resulted in inconsistent comparisons of accuracy and other evaluation metrics among the proposed ML models for dementia prediction. Thus, standard data partition schemes should be adopted (holdout) for the comparison of ML models developed by the researcher for dementia prediction.
Another challenge with ML-based automated diagnostic systems for dementia is the time complexity of the proposed ML algorithms. The time complexity means the overall time require to complete all the computational tasks by the ML model for making a prediction. The ML model can forecast results only after it has been trained on the training data, which takes time to analyze. Furthermore, ML models include a large number of parameters that must be manually modified in the case of supervised learning. As a result, it takes a significant amount of effort and time to fine-tune the hyperparameters of the ML model in order to get higher performance.
DL technology has demonstrated cutting-edge performances for the prediction of various diseases in the recent years. However, DL technology needs a massive quantity of data for model training, which is a time-consuming and tough task. Due to the complexity of data models, training is quite costly. Furthermore, DL necessitates the use of pricey GPUs and hundreds of workstations, which are not effective in terms of economics.
Future research directions
In recent years, several ML models have been presented for the prediction of AD and MCI; nevertheless, there are still certain areas that need to be explored by academics and experts. In this section, we have discussed different research areas and the future prospects of ML algorithms for dementia detection. We infer from this study that the following major parameters have a role in the efficient identification of dementia and its forms.
Data is extremely important in the case of ML-based automated detection of dementia, especially when DL models are considered. Many of the publicly available datasets, however, are modest in size. But future research should concentrate on gathering a huge number of samples for the datasets. In this SLR, we studied ML-based automated diagnostic systems for dementia prediction using three different kinds of data modalities (image, clinical_variable, voice). From Fig. 10 , it can be observed that only the image modality based ML model obtained the higher accuracy along with the large size of the dataset, while the voice modality based ML model obtained the higher accuracy on a small dataset. Thus, for the researchers, there is still room available for designing and developing the automated prediction of dementia and its sub-types by using voice data. Therefore, the interest of researchers have been tremendously raised for the development of automated diagnostic systems for dementia prediction using voice data modality and this trend can be confirmed from the Fig. 4 . There is still a lot room available for the improvement in design and construction of automated diagnostic systems for the dementia using clinical-variable data modality for the researchers. Because, the ML model was developed in the past using clinical-variable data, it displays mix performance by using clinical_variable modality, i.e., when the number of samples is lower in the dataset, the ML shows lower accuracy. Thus. In the future, we need to increase the number of samples in the dataset so that we have larger datasets for experimental purposes and the designed ML model can be effectively evaluated.
In selected studies of this SLR, the majority of ML algorithms belong to the supervised category of learning. While few researchers used an unsupervised ML approach for the prediction of dementia and its subtypes, Altough, unspervsied learning approaches suffer from the limitation such as less accuracy, more expensive in term of computational etc. Therefore, it will encourage scientists and researchers to design and construct new techniques and methods using supervised ML algorithms that are more precise and accurate for the prediction of dementia and its subtypes. Moreover, in this SLR, we have analysed the various ML models based on three data modalities (image, clinical-variable, and voice), and we have comprehensively compared previously proposed ML-based systems in terms of various evaluation metrics, but with different data modalities, it would be suggested that multimodal processing techniques based on ML would provide more reliable and efficient results. Hence, in the future, researchers should exploit multimodal approaches based on ML for a better prediction of dementia and its subtypes.
In contrast to earlier SLR studies that examined numerous ML techniques proposed for the automated diagnosis of dementia and its subtypes (AD, VaD, FTD, and MCI) using one type of data modality, this study reviewed ML methods for dementia considering different types of data modalities such as image data, clinical variables, and voice data. The research articles published from 2011 to 2022 were gathered using different databases. It was pointed out that ML approaches based on image data modality has shown better performance compared with ML methods trained on clinical variables based data and voice data modality. Furthermore, this study critically evaluated the previously proposed methods and highlighted limitations in these methods. To overcome these limitations, this study presented future research directions in the domain of automated dementia prediction using ML approaches. We hope that this SLR will be helpful for AI and ML researchers and medical practitioners who are working in the domain of automated diagnostic systems for dementia prediction.
Data Availability
Not applicable.
Code Availability
Menéndez, G.: La revolución de la longevidad: cambio tecnológico, envejecimiento poblacional y transformación cultural. Revista de Ciencias Sociales 30 (41), 159–178 (2017)
Prince, M.J., Wimo, A., Guerchet, M.M., Ali, G.C., Wu, Y.-T., Prina, M.: World alzheimer report 2015-the global impact of dementia: An analysis of prevalence, incidence, cost and trends (2015)
Vrijsen, J., Matulessij, T., Joxhorst, T., de Rooij, S.E., Smidt, N.: Knowledge, health beliefs and attitudes towards dementia and dementia risk reduction among the dutch general population: a cross-sectional study. BMC public health 21 (1), 1–11 (2021)
Widiger, T.A., Costa, P.T., Association, A.P., et al : Personality Disorders and the Five-factor Model of Personality. JSTOR, (2013)
Lo, R.Y.: The borderland between normal aging and dementia. Tzu-Chi Medical Journal 29 (2), 65 (2017)
WHO: Dementia. World Health Organization. https://www.who.int/news-room/fact-sheets/detail/dementia
Budson, A.E., Solomon, P.R.: Memory Loss E-book: A Practical Guide for Clinicians. Elsevier Health Sciences, (2011)
friendly wyoming, D.: Types of dementia. Dementia friendly wyoming. https://www.dfwsheridan.org/types-dementia
Tackenberg, C., Kulic, L., Nitsch, R.M.: Familial alzheimer’s disease mutations at position 22 of the amyloid \(\beta\) -peptide sequence differentially affect synaptic loss, tau phosphorylation and neuronal cell death in an ex vivo system. PloS one 15 (9), 0239584 (2020)
Wang, H., Kulas, J.A., Wang, C., Holtzman, D.M., Ferris, H.A., Hansen, S.B.: Regulation of beta-amyloid production in neurons by astrocyte-derived cholesterol. Proceedings of the National Academy of Sciences 118 (33) (2021)
Vilchez, D., Saez, I., Dillin, A.: The role of protein clearance mechanisms in organismal ageing and age-related diseases. Nature communications 5 (1), 1–13 (2014)
Breijyeh, Z., Karaman, R.: Comprehensive review on alzheimer’s disease: Causes and treatment. Molecules 25 (24), 5789 (2020)
Long, J.M., Holtzman, D.M.: Alzheimer disease: an update on pathobiology and treatment strategies. Cell 179 (2), 312–339 (2019)
Román, G.C.: Vascular dementia may be the most common form of dementia in the elderly. Journal of the neurological sciences 203 , 7–10 (2002)
Gold, G., Bouras, C., Canuto, A., Bergallo, M.F., Herrmann, F.R., Hof, P.R., Mayor, P.-A., Michel, J.-P., Giannakopoulos, P.: Clinicopathological validation study of four sets of clinical criteria for vascular dementia. American Journal of Psychiatry 159 (1), 82–87 (2002)
Lina, R.: Atrial fibrillation in aging; methodological aspects and the relation to dementia and cerebral vascular disease (2022)
Kuźma, E., Lourida, I., Moore, S.F., Levine, D.A., Ukoumunne, O.C., Llewellyn, D.J.: Stroke and dementia risk: a systematic review and meta-analysis. Alzheimer’s & Dementia 14 (11), 1416–1426 (2018)
Tay, J., Morris, R.G., Tuladhar, A.M., Husain, M., de Leeuw, F.-E., Markus, H.S.: Apathy, but not depression, predicts all-cause dementia in cerebral small vessel disease. Journal of Neurology, Neurosurgery & Psychiatry 91 (9), 953–959 (2020)
Morton, R.E., St. John, P.D., Tyas, S.L.: Migraine and the risk of all-cause dementia, alzheimer’s disease, and vascular dementia: A prospective cohort study in community-dwelling older adults. International journal of geriatric psychiatry 34 (11), 1667–1676 (2019)
Sanford, A.M.: Lewy body dementia. Clinics in geriatric medicine 34 (4), 603–615 (2018)
Taylor, J.-P., McKeith, I.G., Burn, D.J., Boeve, B.F., Weintraub, D., Bamford, C., Allan, L.M., Thomas, A.J., T O’Brien, J.: New evidence on the management of lewy body dementia. The Lancet Neurology 19 (2), 157–169 (2020)
McKEITH, I.G., Galasko, D., Wilcock, G.K., Byrne, E.J.: Lewy body dementia–diagnosis and treatment. The British Journal of Psychiatry 167 (6), 709–717 (1995)
Surendranathan, A., Kane, J.P., Bentley, A., Barker, S.A., Taylor, J.-P., Thomas, A.J., Allan, L.M., McNally, R.J., James, P.W., McKeith, I.G., et al.: Clinical diagnosis of lewy body dementia. BJPsych open 6 (4) (2020)
Finger, E.C.: Frontotemporal dementias. Continuum: Lifelong Learning in Neurology 22 (2 Dementia), 464 (2016)
Rabinovici, G.D., Miller, B.L.: Frontotemporal lobar degeneration. CNS drugs 24 (5), 375–398 (2010)
Organization, W.H., et al.: The ICD-10 classification of mental and behavioural disorders. Clinical descriptions and diagnostic guidelines; 2004. Geneva: WHO (2021)
Ghetti, B., Buratti, E., Boeve, B., Rademakers, R.: Frontotemporal Dementias vol. 320. Springer, (2021)
Custodio, N., Montesinos, R., Lira, D., Herrera-Pérez, E., Bardales, Y., Valeriano-Lorenzo, L.: Mixed dementia: A review of the evidence. Dementia & neuropsychologia 11 , 364–370 (2017)
Garcia-Ptacek, S., Kåreholt, I., Cermakova, P., Rizzuto, D., Religa, D., Eriksdotter, M.: Causes of death according to death certificates in individuals with dementia: a cohort from the swedish dementia registry. Journal of the American Geriatrics Society 64 (11), 137–142 (2016)
Arvanitakis, Z., Shah, R.C., Bennett, D.A.: Diagnosis and management of dementia. Jama 322 (16), 1589–1599 (2019)
Ullah, H., Bin Heyat, M.B., AlSalman, H., Khan, H.M., Akhtar, F., Gumaei, A., Mehdi, A., Muaad, A.Y., Islam, M.S., Ali, A., et al.: An effective and lightweight deep electrocardiography arrhythmia recognition model using novel special and native structural regularization techniques on cardiac signal. Journal of Healthcare Engineering 2022 (2022)
Javeed, A., Ali, L., Mohammed Seid, A., Ali, A., Khan, D., Imrana, Y.: A clinical decision support system (cdss) for unbiased prediction of caesarean section based on features extraction and optimized classification. Computational Intelligence and Neuroscience 2022 (2022)
Imrana, Y., Xiang, Y., Ali, L., Abdul-Rauf, Z., Hu, Y.-C., Kadry, S., Lim, S.: \(\chi\) 2-bidlstm: A feature driven intrusion detection system based on \(\chi\) 2 statistical model and bidirectional lstm. Sensors 22 (5), 2018 (2022)
Ali, L., Zhu, C., Zhao, H., Zhang, Z., Liu, Y.: An integrated system for unbiased parkinson’s disease detection from handwritten drawings. In: Advances in Intelligent Systems and Computing, pp. 3–13. Springer, (2022)
Ali, L., Niamat, A., Khan, J.A., Golilarz, N.A., Xingzhong, X., Noor, A., Nour, R., Bukhari, S.A.C.: An optimized stacked support vector machines based expert system for the effective prediction of heart failure. IEEE Access 7 , 54007–54014 (2019)
Ali, L., Zhu, C., Zhou, M., Liu, Y.: Early diagnosis of parkinson’s disease from multiple voice recordings by simultaneous sample and feature selection. Expert Systems with Applications 137 , 22–28 (2019)
Ali, L., Khan, S.U., Golilarz, N.A., Yakubu, I., Qasim, I., Noor, A., Nour, R.: A feature-driven decision support system for heart failure prediction based on statistical model and gaussian naive bayes. Computational and Mathematical Methods in Medicine 2019 (2019)
Mehbodniya, A., Khan, I.R., Chakraborty, S., Karthik, M., Mehta, K., Ali, L., Nuagah, S.J.: Data mining in employee healthcare detection using intelligence techniques for industry development. Journal of Healthcare Engineering 2022 (2022)
Ali, L., Khan, S.U., Arshad, M., Ali, S., Anwar, M.: A multi-model framework for evaluating type of speech samples having complementary information about parkinson’s disease. In: 2019 International Conference on Electrical, Communication, and Computer Engineering (ICECCE), pp. 1–5 (2019). IEEE
Ahmad, F.S., Ali, L., Khattak, H.A., Hameed, T., Wajahat, I., Kadry, S., Bukhari, S.A.C., et al : A hybrid machine learning framework to predict mortality in paralytic ileus patients using electronic health records (ehrs). Journal of Ambient Intelligence and Humanized Computing 12 (3), 3283–3293 (2021)
Ali, L., Wajahat, I., Golilarz, N.A., Keshtkar, F., Bukhari, S.A.C.: Lda–ga–svm: improved hepatocellular carcinoma prediction through dimensionality reduction and genetically optimized support vector machine. Neural Computing and Applications 33 (7), 2783–2792 (2021)
Ali, L., Zhu, C., Zhang, Z., Liu, Y.: Automated detection of parkinson’s disease based on multiple types of sustained phonations using linear discriminant analysis and genetically optimized neural network. IEEE journal of translational engineering in health and medicine 7 , 1–10 (2019)
Ali, L., He, Z., Cao, W., Rauf, H.T., Imrana, Y., Heyat, M.B.B.: Mmdd-ensemble: A multimodal data–driven ensemble approach for parkinson’s disease detection. Frontiers in Neuroscience 15 (2021)
Rehman, A., Khan, A., Ali, M.A., Khan, M.U., Khan, S.U., Ali, L.: Performance analysis of pca, sparse pca, kernel pca and incremental pca algorithms for heart failure prediction. In: 2020 International Conference on Electrical, Communication, and Computer Engineering (ICECCE), pp. 1–5 (2020). IEEE
Ahmed, F.S., Ali, L., Joseph, B.A., Ikram, A., Mustafa, R.U., Bukhari, S.A.C.: A statistically rigorous deep neural network approach to predict mortality in trauma patients admitted to the intensive care unit. Journal of Trauma and Acute Care Surgery 89 (4), 736–742 (2020)
Imrana, Y., Xiang, Y., Ali, L., Abdul-Rauf, Z.: A bidirectional lstm deep learning approach for intrusion detection. Expert Systems with Applications 185 , 115524 (2021)
Akbar, W., Wu, W.-p., Saleem, S., Farhan, M., Saleem, M.A., Javeed, A., Ali, L.: Development of hepatitis disease detection system by exploiting sparsity in linear support vector machine to improve strength of adaboost ensemble model. Mobile Information Systems 2020 (2020)
Ali, L., Bukhari, S.: An approach based on mutually informed neural networks to optimize the generalization capabilities of decision support systems developed for heart failure prediction. Irbm 42 (5), 345–352 (2021)
McInnes, M.D., Moher, D., Thombs, B.D., McGrath, T.A., Bossuyt, P.M., Clifford, T., Cohen, J.F., Deeks, J.J., Gatsonis, C., Hooft, L., et al : Preferred reporting items for a systematic review and meta-analysis of diagnostic test accuracy studies: the prisma-dta statement. Jama 319 (4), 388–396 (2018)
Liberati, A., Altman, D.G., Tetzlaff, J., Mulrow, C., Gøtzsche, P.C., Ioannidis, J.P., Clarke, M., Devereaux, P.J., Kleijnen, J., Moher, D.: The prisma statement for reporting systematic reviews and meta-analyses of studies that evaluate health care interventions: explanation and elaboration. Journal of clinical epidemiology 62 (10), 1–34 (2009)
Martí-Juan, G., Sanroma-Guell, G., Piella, G.: A survey on machine and statistical learning for longitudinal analysis of neuroimaging data in alzheimer’s disease. Computer methods and programs in biomedicine 189 , 105348 (2020)
McGinnis, J.M., Olsen, L., Goolsby, W.A., Grossmann, C., et al : Clinical Data as the Basic Staple of Health Learning: Creating and Protecting a Public Good: Workshop Summary. National Academies Press, (2011)
Vaughn, V.M., Linder, J.A.: Thoughtless design of the electronic health record drives overuse, but purposeful design can nudge improved patient care. BMJ Publishing Group Ltd (2018)
Doody, R., Stevens, J., Beck, C., Dubinsky, R., Kaye, J., Gwyther, L., Mohs, R., Thal, L., Whitehouse, P., DeKosky, S., et al : Practice parameter: Management of dementia (an evidence-based review): Report of the quality standards subcommittee of the american academy of neurology. Neurology 56 (9), 1154–1166 (2001)
Chi, C.-L., Zeng, W., Oh, W., Borson, S., Lenskaia, T., Shen, X., Tonellato, P.J.: Personalized long-term prediction of cognitive function: Using sequential assessments to improve model performance. Journal of biomedical informatics 76 , 78–86 (2017)
Johnson, K.A., Minoshima, S., Bohnen, N.I., Donohoe, K.J., Foster, N.L., Herscovitch, P., Karlawish, J.H., Rowe, C.C., Carrillo, M.C., Hartley, D.M., et al : Appropriate use criteria for amyloid pet: a report of the amyloid imaging task force, the society of nuclear medicine and molecular imaging, and the alzheimer’s association. Alzheimer’s & Dementia 9 (1), 1–16 (2013)
Shaw, L.M., Arias, J., Blennow, K., Galasko, D., Molinuevo, J.L., Salloway, S., Schindler, S., Carrillo, M.C., Hendrix, J.A., Ross, A., et al : Appropriate use criteria for lumbar puncture and cerebrospinal fluid testing in the diagnosis of alzheimer’s disease. Alzheimer’s & Dementia 14 (11), 1505–1521 (2018)
Mills, K.L., Tamnes, C.K.: Methods and considerations for longitudinal structural brain imaging analysis across development. Developmental cognitive neuroscience 9 , 172–190 (2014)
Ali, L., Zhu, C., Golilarz, N.A., Javeed, A., Zhou, M., Liu, Y.: Reliable parkinson’s disease detection by analyzing handwritten drawings: construction of an unbiased cascaded learning system based on feature selection and adaptive boosting model. Ieee Access 7 , 116480–116489 (2019)
Javeed, A., Rizvi, S.S., Zhou, S., Riaz, R., Khan, S.U., Kwon, S.J.: Heart risk failure prediction using a novel feature selection method for feature refinement and neural network for classification. Mobile Information Systems 2020 (2020)
Javeed, A., Zhou, S., Yongjian, L., Qasim, I., Noor, A., Nour, R.: An intelligent learning system based on random search algorithm and optimized random forest model for improved heart disease detection. IEEE Access 7 , 180235–180243 (2019)
Ali, L., Rahman, A., Khan, A., Zhou, M., Javeed, A., Khan, J.A.: An automated diagnostic system for heart disease prediction based on chi2 statistical model and optimally configured deep neural network. IEEE Access 7 , 34938–34945 (2019)
Maddox, T.M., Rumsfeld, J.S., Payne, P.R.: Questions for artificial intelligence in health care. Jama 321 (1), 31–32 (2019)
Chen, P.-H.C., Liu, Y., Peng, L.: How to develop machine learning models for healthcare. Nature materials 18 (5), 410–414 (2019)
Javeed, A., Khan, S.U., Ali, L., Ali, S., Imrana, Y., Rahman, A.: Machine learning-based automated diagnostic systems developed for heart failure prediction using different types of data modalities: A systematic review and future directions. Computational and Mathematical Methods in Medicine 2022 (2022)
Thacker, S.B., Qualters, J.R., Lee, L.M., for Disease Control, C., Prevention, et al : Public health surveillance in the united states: evolution and challenges. MMWR Suppl 61 (3), 3–9 (2012)
Boerma, J.T., Stansfield, S.K.: Health statistics now: are we making the right investments? The Lancet 369 (9563), 779–786 (2007)
Walport, M., Brest, P.: Sharing research data to improve public health. The Lancet 377 (9765), 537–539 (2011)
Van Panhuis, W.G., Paul, P., Emerson, C., Grefenstette, J., Wilder, R., Herbst, A.J., Heymann, D., Burke, D.S.: A systematic review of barriers to data sharing in public health. BMC public health 14 (1), 1–9 (2014)
Dashtipour, K., Taylor, W., Ansari, S., Zahid, A., Gogate, M., Ahmad, J., Assaleh, K., Arshad, K., Imran, M.A., Abbai, Q.: Detecting alzheimer’s disease using machine learning methods. In: EAI (2021)
Helaly, H.A., Badawy, M., Haikal, A.Y.: Deep learning approach for early detection of alzheimer’s disease. Cognitive Computation, 1–17 (2021)
Vandenberghe, R., Nelissen, N., Salmon, E., Ivanoiu, A., Hasselbalch, S., Andersen, A., Korner, A., Minthon, L., Brooks, D.J., Van Laere, K., et al : Binary classification of 18f-flutemetamol pet using machine learning: comparison with visual reads and structural mri. Neuroimage 64 , 517–525 (2013)
Odusami, M., Maskeliūnas, R., Damaševičius, R., Krilavičius, T.: Analysis of features of alzheimer’s disease: Detection of early stage from functional brain changes in magnetic resonance images using a finetuned resnet18 network. Diagnostics 11 (6), 1071 (2021)
Zheng, Y., Guo, H., Zhang, L., Wu, J., Li, Q., Lv, F.: Machine learning-based framework for differential diagnosis between vascular dementia and alzheimer’s disease using structural mri features. Frontiers in Neurology, 1097 (2019)
Basheer, S., Bhatia, S., Sakri, S.B.: Computational modeling of dementia prediction using deep neural network: Analysis on oasis dataset. IEEE Access 9 , 42449–42462 (2021)
Leong, L.K., Abdullah, A.A.: Prediction of alzheimer’s disease (ad) using machine learning techniques with boruta algorithm as feature selection method. In: Journal of Physics: Conference Series, vol. 1372, p. 012065 (2019). IOP Publishing
Battineni, G., Chintalapudi, N., Amenta, F.: Machine learning in medicine: Performance calculation of dementia prediction by support vector machines (svm). Informatics in Medicine Unlocked 16 , 100200 (2019)
Mathotaarachchi, S., Pascoal, T.A., Shin, M., Benedet, A.L., Kang, M.S., Beaudry, T., Fonov, V.S., Gauthier, S., Rosa-Neto, P., Initiative, A.D.N., et al : Identifying incipient dementia individuals using machine learning and amyloid imaging. Neurobiology of aging 59 , 80–90 (2017)
Aruna, S., Chitra, S.: Machine learning approach for identifying dementia from mri images. International Journal of Computer and Information Engineering 9 (3), 881–888 (2016)
Herzog, N.J., Magoulas, G.D.: Brain asymmetry detection and machine learning classification for diagnosis of early dementia. Sensors 21 (3), 778 (2021)
Battineni, G., Chintalapudi, N., Amenta, F., Traini, E.: A comprehensive machine-learning model applied to magnetic resonance imaging (mri) to predict alzheimer’s disease (ad) in older subjects. Journal of Clinical Medicine 9 (7), 2146 (2020)
Ma, D., Yee, E., Stocks, J.K., Jenkins, L.M., Popuri, K., Chausse, G., Wang, L., Probst, S., Beg, M.F.: Blinded clinical evaluation for dementia of alzheimer’s type classification using fdg-pet: A comparison between feature-engineered and non-feature-engineered machine learning methods. Journal of Alzheimer’s Disease 80 (2), 715–726 (2021)
Bidani, A., Gouider, M.S., Travieso-González, C.M.: Dementia detection and classification from mri images using deep neural networks and transfer learning. In: International Work-Conference on Artificial Neural Networks, pp. 925–933 (2019). Springer
Moscoso, A., Silva-Rodríguez, J., Aldrey, J.M., Cortés, J., Fernández-Ferreiro, A., Gómez-Lado, N., Ruibal, Á., Aguiar, P., Initiative, A.D.N., et al : Prediction of alzheimer’s disease dementia with mri beyond the short-term: Implications for the design of predictive models. NeuroImage: Clinical 23 , 101837 (2019)
Khan, A., Zubair, S.: An improved multi-modal based machine learning approach for the prognosis of alzheimer’s disease. Journal of King Saud University-Computer and Information Sciences (2020)
Mohammed, B.A., Senan, E.M., Rassem, T.H., Makbol, N.M., Alanazi, A.A., Al-Mekhlafi, Z.G., Almurayziq, T.S., Ghaleb, F.A.: Multi-method analysis of medical records and mri images for early diagnosis of dementia and alzheimer’s disease based on deep learning and hybrid methods. Electronics 10 (22), 2860 (2021)
Salvatore, C., Cerasa, A., Battista, P., Gilardi, M.C., Quattrone, A., Castiglioni, I.: Magnetic resonance imaging biomarkers for the early diagnosis of alzheimer’s disease: a machine learning approach. Frontiers in neuroscience 9 , 307 (2015)
Katako, A., Shelton, P., Goertzen, A.L., Levin, D., Bybel, B., Aljuaid, M., Yoon, H.J., Kang, D.Y., Kim, S.M., Lee, C.S., et al : Machine learning identified an alzheimer’s disease-related fdg-pet pattern which is also expressed in lewy body dementia and parkinson’s disease dementia. Scientific reports 8 (1), 1–13 (2018)
Gray, K.R., Aljabar, P., Heckemann, R.A., Hammers, A., Rueckert, D.: Random forest-based manifold learning for classification of imaging data in dementia. In: International Workshop on Machine Learning in Medical Imaging, pp. 159–166 (2011). Springer
De Bruijne, M.: Machine learning approaches in medical image analysis: From detection to diagnosis. Elsevier (2016)
Tong, T., Wolz, R., Gao, Q., Hajnal, J.V., Rueckert, D.: Multiple instance learning for classification of dementia in brain mri. In: International Conference on Medical Image Computing and Computer-Assisted Intervention, pp. 599–606 (2013). Springer
Akhila, J., Markose, C., Aneesh, R.: Feature extraction and classification of dementia with neural network. In: 2017 International Conference on Intelligent Computing, Instrumentation and Control Technologies (ICICICT), pp. 1446–1450 (2017). IEEE
Chen, Y., Pham, T.D.: Development of a brain mri-based hidden markov model for dementia recognition. Biomedical engineering online 12 (1), 1–16 (2013)
Patil, M., Yardi, A.: Ann based dementia diagnosis using dct for brain mr image compression. In: 2013 International Conference on Communication and Signal Processing, pp. 451–454 (2013). IEEE
Gulhare, K.K., Shukla, S., Sharma, L.: Deep neural network classification method to alzheimer’s disease detection. International Journals of Advanced Research in Computer Science and Software Engineering 7 (6), 1–4 (2017)
Chiu, P.-Y., Tang, H., Wei, C.-Y., Zhang, C., Hung, G.-U., Zhou, W.: Nmd-12: A new machine-learning derived screening instrument to detect mild cognitive impairment and dementia. PloS one 14 (3), 0213430 (2019)
Stamate, D., Alghamdi, W., Ogg, J., Hoile, R., Murtagh, F.: A machine learning framework for predicting dementia and mild cognitive impairment. In: 2018 17th IEEE International Conference on Machine Learning and Applications (ICMLA), pp. 671–678 (2018). IEEE
Visser, P.J., Lovestone, S., Legido-Quigley, C.: A metabolite-based machine learning approach to diagnose alzheimer-type dementia in blood: Results from the european medical information framework for alzheimer disease biomarker discovery cohort (2019)
Bansal, D., Chhikara, R., Khanna, K., Gupta, P.: Comparative analysis of various machine learning algorithms for detecting dementia. Procedia computer science 132 , 1497–1502 (2018)
Nori, V.S., Hane, C.A., Martin, D.C., Kravetz, A.D., Sanghavi, D.M.: Identifying incident dementia by applying machine learning to a very large administrative claims dataset. PLoS One 14 (7), 0203246 (2019)
Alam, M.A.U., Roy, N., Holmes, S., Gangopadhyay, A., Galik, E.: Automated functional and behavioral health assessment of older adults with dementia. In: 2016 IEEE First International Conference on Connected Health: Applications, Systems and Engineering Technologies (CHASE), pp. 140–149 (2016). IEEE
Gurevich, P., Stuke, H., Kastrup, A., Stuke, H., Hildebrandt, H.: Neuropsychological testing and machine learning distinguish alzheimer’s disease from other causes for cognitive impairment. Frontiers in aging neuroscience 9 , 114 (2017)
Karaglani, M., Gourlia, K., Tsamardinos, I., Chatzaki, E.: Accurate blood-based diagnostic biosignatures for alzheimer’s disease via automated machine learning. Journal of clinical medicine 9 (9), 3016 (2020)
Ryzhikova, E., Ralbovsky, N.M., Sikirzhytski, V., Kazakov, O., Halamkova, L., Quinn, J., Zimmerman, E.A., Lednev, I.K.: Raman spectroscopy and machine learning for biomedical applications: Alzheimer’s disease diagnosis based on the analysis of cerebrospinal fluid. Spectrochimica Acta Part A: Molecular and Biomolecular Spectroscopy 248 , 119188 (2021)
Cho, P.-C., Chen, W.-H.: A double layer dementia diagnosis system using machine learning techniques. In: International Conference on Engineering Applications of Neural Networks, pp. 402–412 (2012). Springer
Facal, D., Valladares-Rodriguez, S., Lojo-Seoane, C., Pereiro, A.X., Anido-Rifon, L., Juncos-Rabadán, O.: Machine learning approaches to studying the role of cognitive reserve in conversion from mild cognitive impairment to dementia. International journal of geriatric psychiatry 34 (7), 941–949 (2019)
Jin, H., Chien, S., Meijer, E., Khobragade, P., Lee, J., et al : Learning from clinical consensus diagnosis in india to facilitate automatic classification of dementia: Machine learning study. JMIR Mental Health 8 (5), 27113 (2021)
James, C., Ranson, J.M., Everson, R., Llewellyn, D.J.: Performance of machine learning algorithms for predicting progression to dementia in memory clinic patients. JAMA network open 4 (12), 2136553–2136553 (2021)
Bougea, A., Efthymiopoulou, E., Spanou, I., Zikos, P.: A novel machine learning algorithm predicts dementia with lewy bodies versus parkinson’s disease dementia based on clinical and neuropsychological scores. Journal of geriatric psychiatry and neurology, 0891988721993556 (2021)
Salem, F.A., Chaaya, M., Ghannam, H., Al Feel, R.E., El Asmar, K.: Regression based machine learning model for dementia diagnosis in a community setting. Alzheimer’s & Dementia 17 , 053839 (2021)
Garcia-Gutierrez, F., Delgado-Alvarez, A., Delgado-Alonso, C., Díaz-Álvarez, J., Pytel, V., Valles-Salgado, M., Gil, M.J., Hernández-Lorenzo, L., Matías-Guiu, J., Ayala, J.L., et al.: Diagnosis of alzheimer’s disease and behavioural variant frontotemporal dementia with machine learning-aided neuropsychological assessment using feature engineering and genetic algorithms. International journal of geriatric psychiatry 37 (2) (2022)
Mirzaei, G., Adeli, H.: Machine learning techniques for diagnosis of alzheimer disease, mild cognitive disorder, and other types of dementia. Biomedical Signal Processing and Control 72 , 103293 (2022)
Hsiu, H., Lin, S.-K., Weng, W.-L., Hung, C.-M., Chang, C.-K., Lee, C.-C., Chen, C.-T.: Discrimination of the cognitive function of community subjects using the arterial pulse spectrum and machine-learning analysis. Sensors 22 (3), 806 (2022)
Shahzad, A., Dadlani, A., Lee, H., Kim, K.: Automated prescreening of mild cognitive impairment using shank-mounted inertial sensors based gait biomarkers. IEEE Access (2022)
Hane, C.A., Nori, V.S., Crown, W.H., Sanghavi, D.M., Bleicher, P.: Predicting onset of dementia using clinical notes and machine learning: case-control study. JMIR medical informatics 8 (6), 17819 (2020)
Aschwanden, D., Aichele, S., Ghisletta, P., Terracciano, A., Kliegel, M., Sutin, A.R., Brown, J., Allemand, M.: Predicting cognitive impairment and dementia: A machine learning approach. Journal of Alzheimer’s Disease 75 (3), 717–728 (2020)
Ryu, S.-E., Shin, D.-H., Chung, K.: Prediction model of dementia risk based on xgboost using derived variable extraction and hyper parameter optimization. IEEE Access 8 , 177708–177720 (2020)
de Langavant, L.C., Bayen, E., Yaffe, K., et al : Unsupervised machine learning to identify high likelihood of dementia in population-based surveys: development and validation study. Journal of medical Internet research 20 (7), 10493 (2018)
Fouladvand, S., Mielke, M.M., Vassilaki, M., Sauver, J.S., Petersen, R.C., Sohn, S.: Deep learning prediction of mild cognitive impairment using electronic health records. In: 2019 IEEE International Conference on Bioinformatics and Biomedicine (BIBM), pp. 799–806 (2019). IEEE
Balea-Fernandez, F.J., Martinez-Vega, B., Ortega, S., Fabelo, H., Leon, R., Callico, G.M., Bibao-Sieyro, C.: Analysis of risk factors in dementia through machine learning. Journal of Alzheimer’s Disease 79 (2), 845–861 (2021)
Chlasta, K., Wołk, K.: Towards computer-based automated screening of dementia through spontaneous speech. Frontiers in Psychology, 4091 (2021)
Chien, Y.-W., Hong, S.-Y., Cheah, W.-T., Yao, L.-H., Chang, Y.-L., Fu, L.-C.: An automatic assessment system for alzheimer’s disease based on speech using feature sequence generator and recurrent neural network. Scientific Reports 9 (1), 1–10 (2019)
Shimoda, A., Li, Y., Hayashi, H., Kondo, N.: Dementia risks identified by vocal features via telephone conversations: A novel machine learning prediction model. PloS one 16 (7), 0253988 (2021)
Nishikawa, K., Akihiro, K., Hirakawa, R., Kawano, H., Nakatoh, Y.: Machine learning model for discrimination of mild dementia patients using acoustic features. Cognitive Robotics (2021)
Liu, L., Zhao, S., Chen, H., Wang, A.: A new machine learning method for identifying alzheimer’s disease. Simulation Modelling Practice and Theory 99 , 102023 (2020)
Searle, T., Ibrahim, Z., Dobson, R.: Comparing natural language processing techniques for alzheimer’s dementia prediction in spontaneous speech. arXiv preprint arXiv:2006.07358 (2020)
Zhu, Y., Tran, B., Liang, X., Batsis, J.A., Roth, R.M.: Towards interpretability of speech pause in dementia detection using adversarial learning. arXiv preprint arXiv:2111.07454 (2021)
Ossewaarde, R., Jonkers, R., Jalvingh, F., Bastiaanse, R.: Classification of spontaneous speech of individuals with dementia based on automatic prosody analysis using support vector machines (svm). In: The Thirty-Second International Flairs Conference (2019)
Xue, C., Karjadi, C., Paschalidis, I.C., Au, R., Kolachalama, V.B.: Detection of dementia on voice recordings using deep learning: a framingham heart study. Alzheimer’s research & therapy 13 (1), 1–15 (2021)
Weiner, J., Engelbart, M., Schultz, T.: Manual and automatic transcriptions in dementia detection from speech. In: INTERSPEECH, pp. 3117–3121 (2017)
Sadeghian, R., Schaffer, J.D., Zahorian, S.A.: Speech processing approach for diagnosing dementia in an early stage (2017)
Khodabakhsh, A., Yesil, F., Guner, E., Demiroglu, C.: Evaluation of linguistic and prosodic features for detection of alzheimer’s disease in turkish conversational speech. EURASIP Journal on Audio, Speech, and Music Processing 2015 (1), 1–15 (2015)
Edwards, E., Dognin, C., Bollepalli, B., Singh, M.K., Analytics, V.: Multiscale system for alzheimer’s dementia recognition through spontaneous speech. In: INTERSPEECH, pp. 2197–2201 (2020)
Kumar, Y., Maheshwari, P., Joshi, S., Baths, V.: Ml-based analysis to identify speech features relevant in predicting alzheimer’s disease. arXiv preprint arXiv:2110.13023 (2021)
Luz, S., de la Fuente, S., Albert, P.: A method for analysis of patient speech in dialogue for dementia detection. arXiv preprint arXiv:1811.09919 (2018)
Syed, Z.S., Syed, M.S.S., Lech, M., Pirogova, E.: Automated recognition of alzheimer’s dementia using bag-of-deep-features and model ensembling. IEEE Access 9 , 88377–88390 (2021)
Orsulic-Jeras, S., Sanders, B., Powers, S., Ejaz, F., Cordell, A., Wilk, C.: Developing a reminiscence therapy platform-lifebio memorytm-with a novel machine-learning-based application that transfers speech to text and generates life stories for nursing home residents with dementia. Alzheimer’s & Dementia 17 , 052281 (2021)
Sarawgi, U., Zulfikar, W., Soliman, N., Maes, P.: Multimodal inductive transfer learning for detection of alzheimer’s dementia and its severity. arXiv preprint arXiv:2009.00700 (2020)
Calzà, L., Gagliardi, G., Favretti, R.R., Tamburini, F.: Linguistic features and automatic classifiers for identifying mild cognitive impairment and dementia. Computer Speech & Language 65 , 101113 (2021)
Haider, F., De La Fuente, S., Luz, S.: An assessment of paralinguistic acoustic features for detection of alzheimer’s dementia in spontaneous speech. IEEE Journal of Selected Topics in Signal Processing 14 (2), 272–281 (2019)
Lopez-de-Ipiña, K., Alonso, J.B., Solé-Casals, J., Barroso, N., Henriquez, P., Faundez-Zanuy, M., Travieso, C.M., Ecay-Torres, M., Martinez-Lage, P., Eguiraun, H.: On automatic diagnosis of alzheimer’s disease based on spontaneous speech analysis and emotional temperature. Cognitive Computation 7 (1), 44–55 (2015)
Orimaye, S.O., Wong, J.S.-M., Golden, K.J.: Learning predictive linguistic features for alzheimer’s disease and related dementias using verbal utterances. In: Proceedings of the Workshop on Computational Linguistics and Clinical Psychology: From Linguistic Signal to Clinical Reality, pp. 78–87 (2014)
Santander-Cruz, Y., Salazar-Colores, S., Paredes-García, W.J., Guendulain-Arenas, H., Tovar-Arriaga, S.: Semantic feature extraction using sbert for dementia detection. Brain Sciences 12 (2), 270 (2022)
Download references
Acknowledgements
The first author’s learning process was supported by the National E-Infrastructure for Aging Research (NEAR), Sweden. NEAR is working on improving the health condition of older adults in Sweden.
Open access funding provided by Blekinge Institute of Technology. This research received no external funding.
Author information
Ashir Javeed and Ana Luiza Dallora contributed equally to this work.
Authors and Affiliations
Aging Research Center, Karolinska Institutet, Tomtebodavagen, Stockholm, 17165, Solna, Sweden
Ashir Javeed
Department of Health, Blekinge Institute of Technology, Valhallavägen 1, Karlskrona, 37141, Blekinge, Sweden
Ashir Javeed, Ana Luiza Dallora, Johan Sanmartin Berglund & Peter Anderberg
Department of Computer Science, University of Science and Technology Bannu, Township, Bannu, 28100, Khyber-Pakhtunkhwa, Pakistan
Department of Electrical Engineering, University of Science and Technology Bannu, Township, Bannu, 28100, Khyber-Pakhtunkhwa, Pakistan
Liaqata Ali
School of Health Sciences, University of Skovde, Högskolevägen 1, Skövde, SE-541 28, Skövde, Sweden
Peter Anderberg
You can also search for this author in PubMed Google Scholar
Contributions
Conceptualization by Peter Anderber, Data curation by Liaqat ALi, Formal analysis by Ana Luiza Dallora, Write up and Methodology by Ashir Javeed, Proofread by Arif Ali, Supervised by Johan Sanmartin Berglund. If any of the sections are not relevant to your manuscript.
Corresponding author
Correspondence to Johan Sanmartin Berglund .
Ethics declarations
Ethics approval.
This study was carried out in accordance with the Declaration of Helsinki and was approved by the Research Ethics Committee at Blekinge Institute of Technology (BTH).
Consent to Participate
Consent for publication, conflict of interest.
The authors declare no conflict of interest.
Additional information
Publisher’s note.
Springer Nature remains neutral with regard to jurisdictional claims in published maps and institutional affiliations.
Rights and permissions
Open Access This article is licensed under a Creative Commons Attribution 4.0 International License, which permits use, sharing, adaptation, distribution and reproduction in any medium or format, as long as you give appropriate credit to the original author(s) and the source, provide a link to the Creative Commons licence, and indicate if changes were made. The images or other third party material in this article are included in the article's Creative Commons licence, unless indicated otherwise in a credit line to the material. If material is not included in the article's Creative Commons licence and your intended use is not permitted by statutory regulation or exceeds the permitted use, you will need to obtain permission directly from the copyright holder. To view a copy of this licence, visit http://creativecommons.org/licenses/by/4.0/ .
Reprints and permissions
About this article
Javeed, A., Dallora, A.L., Berglund, J.S. et al. Machine Learning for Dementia Prediction: A Systematic Review and Future Research Directions. J Med Syst 47 , 17 (2023). https://doi.org/10.1007/s10916-023-01906-7
Download citation
Received : 16 July 2022
Accepted : 03 January 2023
Published : 01 February 2023
DOI : https://doi.org/10.1007/s10916-023-01906-7
Share this article
Anyone you share the following link with will be able to read this content:
Sorry, a shareable link is not currently available for this article.
Provided by the Springer Nature SharedIt content-sharing initiative
- Dementia prediction
- Feature selection
- Machine learning
- Deep learning
- Find a journal
- Publish with us
- Track your research
A cultural approach to dementia prevention
- An Introduction to Alzheimer’s Disease: What is it?

By: Adrianna Fusco
Introduction: Alzheimer’s disease, something we hear about online, in commercials, on news stations, and in many other parts of life. However, we are never told much about Alzheimer’s disease other than the devastating impacts it has. What is Alzheimer’s disease? What are the symptoms or signs to look out for? How does it progress? What causes it? How can it be prevented?
What is it? Alzheimer’s disease is a form of dementia, which is just an umbrella term used to describe loss of memory, language, problem solving, and other thinking abilities. More specifically, Alzheimer’s diseaseis a progressive, neurodegenerative disease that is categorized by a loss of memory, along with basic life skills like eating, bathing, talking, etc.
Symptoms: Common symptoms include: memory loss, paranoia, depression, anger, aggression, anxiety, apathy, loneliness, and psychosis. These symptoms vary from person to person.
Progress: As mentioned above, Alzheimer’s disease is a progressive disease. This means that it develops and gets worse over time. In the first stages of Alzheimer’s disease, there is usually very mild memory loss or problems with thinking abilities. The person may have a hard time remembering where they placed something or have a hard time recalling the right word to say. However, they still are independent, meaning they can still take care of themselves and do things like driving.
During the middle stages of Alzheimer’s disease, the cognitive processes get worse. Now the person may not be able to remember their personal history, like their address or phone number. They also may have a hard time recalling memories or remembering something from their past. The person is no longer able to take care of themselves because in this stage, they tend to forget where they are and often have a hard time using the bathroom or getting dressed appropriately for the day. An example of this is the person wearing shorts in the winter. Along with the cognitive changes, the person may begin to feel sad, lonely, anxious, and paranoid. The symptoms vary from person to person.
When the person hits stage 2, they will need a caregiver to assist them with their tasks and the caregiving will increase as the disease progresses. However, it’s important to help them without trying to do everything for them. They are still adults and they want to be treated as such, so it’s important to still let them have at least some control over their life. Whether that’s letting them do simply chores, like folding clothes, or doing activities, like arts and crafts. This will help provide a sense of normalcy.
The final stage of Alzheimer’s disease is when people begin to lose sense and control of the environment around them. By this point, the cognitive abilities of the individual have tremendously decreased. They can no longer speak in long formulated sentences, instead they speak in short fragments or words. They have trouble completing everyday tasks like walking, sitting, eating, and drinking. This means that they require around the clock assistance to make sure that they are remembering to eat and to help them eat. In general, the assistance is meant to make sure the person is safe and is living to their best ability. At this point, the individuals are very susceptible to infections. When the symptoms and daily conditions get really bad, usually, families turn to hospice care, so that the patient is comfortable at the end of their life. Hospice care also provides emotional support to loved ones, which is vital. Losing a loved one can cause serious emotional and mental strain, so that support is important.
The cause of Alzheimer’s disease is still being researched, but researchers have identified what they believe to be the main culprits of the disease: plaques and tangles.
Plaques are deposits of amyloid beta that forms between nerve cells that blocks the signals and stops the right materials from being sent to the nerve for survival. In a healthy brain, amyloid beta is used to help support neural repair and growth. However, in Alzheimer’s disease, there is an overproduction of this amyloid beta protein that disturbs these cells and eventually causes the death of the cells. The death of the old cells causes the loss of old memories and information. The blocking of nerve cells can stop the production of new connections, which means short term memories are not being accurately encoded in the brain to become long term memories.
Tangles are made up of twisted tau that builds up between cells. In a healthy brain, tau is used to help support neural strength and is important in keeping stability in the cells. However, a build up leads to the cells not being able to receive signals and the supplies it needs to function (i.e. energy). These lead to death of the cells, leading to loss of information and life skills.
There is also a biomarker known as APOE-4, that is thought to predispose people to Alzheimer’s disease. This gene along with some environmental stressors could affect whether someone gets the disease and the progression of it. However, a lot of research is still being conducted on this topic and we are constantly rerouting what we know, as new information is found.
Alzheimer’s disease is a terrible disease that claims the lives of a lot of people every year. It’s important to know the signs and to check up with your doctor when anything seems unusual. Alzheimer’s disease and dementia are not a normal part of aging, so see your doctor if you notice any issues with your memory. The earlier the disease is detected, the better it can be treated.
Stay tuned for more blog posts about Alzheimer’s disease, including a look into the mental health of caregivers, prevention, treatment, and more! We also will be writing posts about interviews with doctors, as well as posts about brain health!
Thank you for reading!
References:
“Alzheimer’s Caregivers: 8 Tips for People Caring for a Loved One With Alzheimer’s Disease or Dementia: Caregivers.” 30Seconds Health ,
30seconds.com/health/tip/14389/Alzheimers-Caregivers-8-Tips-for-People-Caring-for-a-Loved-One-With -Alzheimers-Disease-or-Dementia.
Mayeux, Richard, et al. “Treatment of Alzheimer’s Disease: NEJM.” Edited by Alastair J.J. Wood, New England Journal of Medicine , 16 Mar. 2000, www.nejm.org/doi/pdf/10.1056/NEJM199911253412207.
NHS Choices, NHS, 10 May 2018,
www.nhs.uk/conditions/alzheimers-disease/causes/#:~:text=Alzheimer’s%20disease%20is%20thought%2 0to,form%20tangles%20within%20brain%20cells.
Porsteinsson, Anton P., et al. “Neuropsychiatric Symptoms in Dementia: A Cause or Consequence?” American Journal of Psychiatry , American Psychiatric Association Publishing, 30 Apr. 2015, ajp.psychiatryonline.org/doi/10.1176/appi.ajp.2015.15030277#:~:text=The%20term%20neuropsychiatric %20symptoms%20describes%20heterogeneous%20behavioral%20or,agitation%2C%20anxiety%2C%20 apathy%2C%20depression%2C%20psychosis%2C%20and%20sleep%20disturbance.
“Stages of Alzheimer’s.” Alzheimer’s Disease and Dementia , www.alz.org/alzheimers-dementia/stages.
“What Is Alzheimer’s?” Alzheimer’s Disease and Dementia ,
www.alz.org/alzheimers-dementia/what-is-alzheimers.
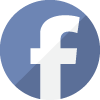
- Introduction
- Conclusions
- Article Information
Models were stratified by age, cohort (sex), and calendar time, and adjusted for Southern European/Mediterranean ancestry (yes/no), married (yes/no), living alone (yes/no), smoking status (never, former, current smoker 1-14 cigarettes/d, 15-24 cigarettes/d, or ≥25 cigarettes/d), physical activity (<3.0, 3.0-8.9, 9.0-17.9, 18.0-26.9, ≥27.0 metabolic equivalent of task–h/wk), multivitamin use (yes/no), history of hypertension (yes/no), history of hypercholesterolemia (yes/no), history of diabetes (yes/no), in women postmenopausal status and menopausal hormone use (premenopausal, postmenopausal [no, past, or current hormone use]), total energy intake (kcal/d), family history of dementia (yes/no), history of depression (yes/no), census socioeconomic status (9-variable score, in quintiles), and body mass index calculated as weight in kilograms divided by height in meters squared (<23, 23-25, 25-30, 30-35, ≥35). Pooled results were obtained by pooling the datasets of the cohorts. AMED score is without monounsaturated:saturated fats intake ratio component. AHEI score is without polyunsaturated fats intake component. HR indicates hazard ratio.
a Reference value.
b P < .05.
Substitution analysis of 5 g/d intake of olive oil for the equivalent amount of butter, other vegetable oils, mayonnaise, and margarine. All Cox proportional hazards models were stratified by age and calendar time. Models were adjusted for Southern European/Mediterranean ancestry (yes/no), married (yes/no), living alone (yes/no), smoking status (never, former, current smoker 1-14 cigarettes/d, 15-24 cigarettes/d, or ≥25 cigarettes/d), alcohol intake (0, 0.1-4.9, 5.0-9.9, 10.0-14.9, and ≥15.0 g/d), physical activity (<3.0, 3.0-8.9, 9.0-17.9, 18.0-26.9, ≥27.0 metabolic equivalent of task–h/wk), multivitamin use (yes/no), history of hypertension (yes/no), history of hypercholesterolemia (yes/no), in women postmenopausal status and menopausal hormone use (premenopausal, postmenopausal [no, past, or current hormone use]), total energy intake (kcal/d), family history of dementia (yes/no), history of depression (yes/no), census socioeconomic status (9-variable score, in quintiles), body mass index calculated as weight in kilograms divided by height in meters squared (<23, 23-25, 25-30, 30-35, ≥35), red meat, fruits and vegetables, nuts, soda, whole grains intake (in quintiles), and trans-fat. Pooled results were obtained by pooling the data sets of the cohorts and Cox proportional hazards model 3 was further stratified by cohort (sex). HR indicates hazard ratio.
eTable 1. Odds Ratios for Dementia-Related Mortality by APOE4 Allelic Dosage
eTable 2. Risk of Death With Dementia (Composite Outcome) According to Categories of Total Olive Oil
eTable 3. Joint Associations of Olive Oil Intake and AMED (A), and AHEI (B) With Dementia-Related Mortality Risk
eTable 4. Risk of Dementia-Related Mortality According to Categories of Total Olive Oil in the Genomic DNA Subsample
eFigure. Subgroup Analyses for 5g/d Increase in Olive Oil Intake With Dementia-Related Mortality Risk
eTable 5. Risk of Dementia-Related Mortality According to Categories of Total Olive Oil Without Stopping Diet Update Upon Report of Intermediate Non-Fatal Events
eTable 6. Risk of Dementia Mortality According to Categories of Total Olive Oil Applying a 4-Year Lag Period Between Dietary Intake and Dementia Mortality
eTable 7. Risk of Dementia-Related Mortality According to Categories of Total Olive Oil Adjusting for Other Covariates
eTable 8. Risk of Mortality From Dementia and Other Causes of Death According to Categories of Total Olive Oil Applying a Competing Risk Model
eReferences
Data Sharing Statement
See More About
Sign up for emails based on your interests, select your interests.
Customize your JAMA Network experience by selecting one or more topics from the list below.
- Academic Medicine
- Acid Base, Electrolytes, Fluids
- Allergy and Clinical Immunology
- American Indian or Alaska Natives
- Anesthesiology
- Anticoagulation
- Art and Images in Psychiatry
- Artificial Intelligence
- Assisted Reproduction
- Bleeding and Transfusion
- Caring for the Critically Ill Patient
- Challenges in Clinical Electrocardiography
- Climate and Health
- Climate Change
- Clinical Challenge
- Clinical Decision Support
- Clinical Implications of Basic Neuroscience
- Clinical Pharmacy and Pharmacology
- Complementary and Alternative Medicine
- Consensus Statements
- Coronavirus (COVID-19)
- Critical Care Medicine
- Cultural Competency
- Dental Medicine
- Dermatology
- Diabetes and Endocrinology
- Diagnostic Test Interpretation
- Drug Development
- Electronic Health Records
- Emergency Medicine
- End of Life, Hospice, Palliative Care
- Environmental Health
- Equity, Diversity, and Inclusion
- Facial Plastic Surgery
- Gastroenterology and Hepatology
- Genetics and Genomics
- Genomics and Precision Health
- Global Health
- Guide to Statistics and Methods
- Hair Disorders
- Health Care Delivery Models
- Health Care Economics, Insurance, Payment
- Health Care Quality
- Health Care Reform
- Health Care Safety
- Health Care Workforce
- Health Disparities
- Health Inequities
- Health Policy
- Health Systems Science
- History of Medicine
- Hypertension
- Images in Neurology
- Implementation Science
- Infectious Diseases
- Innovations in Health Care Delivery
- JAMA Infographic
- Law and Medicine
- Leading Change
- Less is More
- LGBTQIA Medicine
- Lifestyle Behaviors
- Medical Coding
- Medical Devices and Equipment
- Medical Education
- Medical Education and Training
- Medical Journals and Publishing
- Mobile Health and Telemedicine
- Narrative Medicine
- Neuroscience and Psychiatry
- Notable Notes
- Nutrition, Obesity, Exercise
- Obstetrics and Gynecology
- Occupational Health
- Ophthalmology
- Orthopedics
- Otolaryngology
- Pain Medicine
- Palliative Care
- Pathology and Laboratory Medicine
- Patient Care
- Patient Information
- Performance Improvement
- Performance Measures
- Perioperative Care and Consultation
- Pharmacoeconomics
- Pharmacoepidemiology
- Pharmacogenetics
- Pharmacy and Clinical Pharmacology
- Physical Medicine and Rehabilitation
- Physical Therapy
- Physician Leadership
- Population Health
- Primary Care
- Professional Well-being
- Professionalism
- Psychiatry and Behavioral Health
- Public Health
- Pulmonary Medicine
- Regulatory Agencies
- Reproductive Health
- Research, Methods, Statistics
- Resuscitation
- Rheumatology
- Risk Management
- Scientific Discovery and the Future of Medicine
- Shared Decision Making and Communication
- Sleep Medicine
- Sports Medicine
- Stem Cell Transplantation
- Substance Use and Addiction Medicine
- Surgical Innovation
- Surgical Pearls
- Teachable Moment
- Technology and Finance
- The Art of JAMA
- The Arts and Medicine
- The Rational Clinical Examination
- Tobacco and e-Cigarettes
- Translational Medicine
- Trauma and Injury
- Treatment Adherence
- Ultrasonography
- Users' Guide to the Medical Literature
- Vaccination
- Venous Thromboembolism
- Veterans Health
- Women's Health
- Workflow and Process
- Wound Care, Infection, Healing
Get the latest research based on your areas of interest.
Others also liked.
- Download PDF
- X Facebook More LinkedIn
Tessier A , Cortese M , Yuan C, et al. Consumption of Olive Oil and Diet Quality and Risk of Dementia-Related Death. JAMA Netw Open. 2024;7(5):e2410021. doi:10.1001/jamanetworkopen.2024.10021
Manage citations:
© 2024
- Permissions
Consumption of Olive Oil and Diet Quality and Risk of Dementia-Related Death
- 1 Department of Nutrition, Harvard T.H. Chan School of Public Health, Boston, Massachusetts
- 2 School of Public Health, the Second Affiliated Hospital, Zhejiang University School of Medicine, Hangzhou, China
- 3 Department of Epidemiology, Harvard T.H. Chan School of Public Health, Boston, Massachusetts
- 4 Channing Division of Network Medicine, Department of Medicine, Brigham and Women’s Hospital and Harvard Medical School, Boston, Massachusetts
- 5 Department of Public Health and Novo Nordisk Foundation Center for Basic Metabolic Research, Faculty of Health and Medical Sciences, University of Copenhagen, Copenhagen, Denmark
Question Is the long-term consumption of olive oil associated with dementia-related death risk?
Findings In a prospective cohort study of 92 383 adults observed over 28 years, the consumption of more than 7 g/d of olive oil was associated with a 28% lower risk of dementia-related death compared with never or rarely consuming olive oil, irrespective of diet quality.
Meaning These results suggest that olive oil intake represents a potential strategy to reduce dementia mortality risk.
Importance Age-standardized dementia mortality rates are on the rise. Whether long-term consumption of olive oil and diet quality are associated with dementia-related death is unknown.
Objective To examine the association of olive oil intake with the subsequent risk of dementia-related death and assess the joint association with diet quality and substitution for other fats.
Design, Setting, and Participants This prospective cohort study examined data from the Nurses’ Health Study (NHS; 1990-2018) and Health Professionals Follow-Up Study (HPFS; 1990-2018). The population included women from the NHS and men from the HPFS who were free of cardiovascular disease and cancer at baseline. Data were analyzed from May 2022 to July 2023.
Exposures Olive oil intake was assessed every 4 years using a food frequency questionnaire and categorized as (1) never or less than once per month, (2) greater than 0 to less than or equal to 4.5 g/d, (3) greater than 4.5 g/d to less than or equal to 7 g/d, and (4) greater than 7 g/d. Diet quality was based on the Alternative Healthy Eating Index and Mediterranean Diet score.
Main Outcome and Measure Dementia death was ascertained from death records. Multivariable Cox proportional hazards regressions were used to estimate hazard ratios (HRs) and 95% CIs adjusted for confounders including genetic, sociodemographic, and lifestyle factors.
Results Of 92 383 participants, 60 582 (65.6%) were women and the mean (SD) age was 56.4 (8.0) years. During 28 years of follow-up (2 183 095 person-years), 4751 dementia-related deaths occurred. Individuals who were homozygous for the apolipoprotein ε4 ( APOE ε4 ) allele were 5 to 9 times more likely to die with dementia. Consuming at least 7 g/d of olive oil was associated with a 28% lower risk of dementia-related death (adjusted pooled HR, 0.72 [95% CI, 0.64-0.81]) compared with never or rarely consuming olive oil ( P for trend < .001); results were consistent after further adjustment for APOE ε4 . No interaction by diet quality scores was found. In modeled substitution analyses, replacing 5 g/d of margarine and mayonnaise with the equivalent amount of olive oil was associated with an 8% (95% CI, 4%-12%) to 14% (95% CI, 7%-20%) lower risk of dementia mortality. Substitutions for other vegetable oils or butter were not significant.
Conclusions and Relevance In US adults, higher olive oil intake was associated with a lower risk of dementia-related mortality, irrespective of diet quality. Beyond heart health, the findings extend the current dietary recommendations of choosing olive oil and other vegetable oils for cognitive-related health.
One-third of older adults die with Alzheimer disease or another dementia. 1 While deaths from diseases such as stroke and heart disease have been decreasing over the past 20 years, age-standardized dementia mortality rates have been on the rise. 2 The Mediterranean diet has gained in popularity owing to its recognized, multifaceted health benefits, particularly on cardiovascular outcomes. 3 Accruing evidence suggests this dietary pattern also has a beneficial effect on cognitive health. 4 As part of the Mediterranean diet, olive oil may exert anti-inflammatory and neuroprotective effects due to its high content of monounsaturated fatty acids and other compounds with antioxidant properties such as vitamin E and polyphenols. 5 A substudy conducted as part of the Prevencion con Dieta Mediterranea (PREDIMED) randomized trial provided evidence that higher intake of olive oil for 6.5 years combined with adherence to a Mediterranean diet was protective of cognitive decline when compared with a low-fat control diet. 6 - 8
Given that most previous studies on olive oil consumption and cognition were conducted in Mediterranean countries, 7 - 10 studying the US population, where olive oil consumption is generally lower, could offer unique insights. Recently, we showed that olive oil consumption was associated with a lower risk of total and cause-specific mortality in large US prospective cohort studies, including a 29% (95% CI, 22%-36%) lower risk for neurodegenerative disease mortality in participants who consumed more than 7 g/d of olive oil compared with little or none. 11 However, this previous analysis was not designed to examine the association of olive oil and diet quality with dementia-related mortality, and therefore the latter remains unclear.
In this study, we examined the association between total olive oil consumption and the subsequent risk of dementia-related mortality in 2 large prospective studies of US women and men. Additionally, we evaluated the joint associations of diet quality (adherence to the Mediterranean diet and Alternative Healthy Eating Index [AHEI] score) and olive oil consumption with the risk of dementia-related mortality. We also estimated the difference in the risk of dementia-related mortality when other dietary fats were substituted with an equivalent amount of olive oil.
Analyses were performed in 2 large US prospective cohorts: the Nurses’ Health Study I (NHS) and the Health Professionals Follow-Up Study (HPFS). The NHS was initiated in 1976 and recruited 121 700 US female registered nurses aged 30 to 55 years. 12 The HPFS was established in 1986 and included 51 525 male health professionals aged 40 to 75 years. 13 The cohorts have been described elsewhere. 12 , 13 Lifestyle factors and medical history were assessed biennially through mailed questionnaires, with a follow-up rate greater than 90%. Baseline for this analysis was 1990, which is when the food frequency questionnaires (FFQs) first included information on olive oil consumption.
Participants with a history of cardiovascular disease (CVD) or cancer at baseline, with missing data on olive oil consumption, or who reported implausible total energy intakes (<500 or >3500 kcal/d for women and <800 or >4200 kcal/d for men) were excluded. The completion of the questionnaire self-selected cognitively highly functioning women and men. In total, 60 582 women and 31 801 men were included. The study protocol was approved by the institutional review boards of the Brigham and Women’s Hospital and Harvard T.H. Chan School of Public Health, which deemed the participants’ completion of the questionnaire to be considered as implied consent. This report followed the Strengthening the Reporting of Observational Studies in Epidemiology ( STROBE ) reporting guideline.
Dietary intake was measured using a validated greater than 130-item FFQ administered in 1990 and every 4 years thereafter. The validity and reliability of the FFQ have been described previously. 14 Participants were asked how frequently they consumed specific foods, including types of fats and oils used for cooking or added to meals in the past 12 months. Total olive oil intake was determined by summing up answers to 3 questions related to olive oil consumption (ie, olive oil used for salad dressings, olive oil added to food or bread, and olive oil used for baking and frying at home). The equivalent of 1 tablespoon of olive oil was considered to be 13.5 g. Intakes of other fats and nutrients were calculated using the United States Department of Agriculture and Harvard University Food Composition Database, 15 and biochemical analyses. The nutritional composition of olive oil and other types of fat, as well as trends of types of fat intake in the NHS and HPFS, have been reported previously. 11
Adherence to the Mediterranean diet was assessed using a modified version of the 9-point Alternative Mediterranean index (AMED) score. 16 Adherence to the AHEI (0-110), previously associated with lower risk of chronic disease, was also computed. 17 Higher scores indicated better overall diet quality.
The apolipoprotein E ε4 ( APOE ε4 ) allele is known to interfere with lipid and glucose metabolism such that it increases the risk of dementia. 18 APOE genotyping was conducted in a subset of 27 296 participants. Blood samples were collected between 1989 and 1990 in the NHS and between 1993 and 1995 in the HPFS. NHS participants who had not provided blood samples were invited to contribute buccal samples from 2002 to 2004. DNA was extracted with the ReturPureGene DNA Isolation Kit (Gentra Systems). The APOE genotype was determined using a Taqman Assay (Applied Biosystems) 19 in 5069 participants, and through imputation from multiple genome-wide association studies, 20 which has shown high accuracy, 20 in the remaining subset.
Deaths were ascertained from state vital statistics records and the National Death Index or by reports from next of kin or the postal authorities. The follow-up for mortality exceeded 98% in these cohorts. Dementia deaths were determined by physician review of medical records, autopsy reports, or death certificates. Dementia deaths were those in which dementia was listed as the underlying cause of death, or as a contributing cause of death, or as reported by the family, in the absence of a more likely cause. The International Classification of Diseases, Eighth Revision (ICD-8) was used in the NHS and ICD-9 in the HPFS, which were the revisions used at the inception of those cohorts. Dementia deaths included codes 290.0 (senile dementia, simple type), 290.1 (presenile dementia), and 331.0 (Alzheimer disease). To test the validity of the dementia mortality outcome, we examined the likelihood of dementia mortality by APOE ε4 allelic dosage (eTable 1 in Supplement 1 ). 18 A composite outcome was also created including both participants who reported having dementia during follow-up and later died, with those who had dementia reported on their death certificate.
Participants completed biennial questionnaires reporting updates on body weight, smoking, physical activity, multivitamin use, menopausal status, and postmenopausal hormone use in women, family history of dementia, self-report of chronic diseases, and ancestry. History of depression was identified based on antidepressive medication use and self-report of depression. Socioeconomic status (SES) was established through a composite score derived from home address details and various factors such as income, education, and housing; the composite score methods are described in a previous report. 21 Body mass index (BMI) was obtained by dividing the weight in kilograms by the height in meters squared.
In each cohort, age-stratified Cox proportional hazard models were used to evaluate the association of olive oil intake with dementia-related mortality. Participant person-time was calculated from baseline until end of follow-up (June 30, 2018, in NHS; January 31, 2018, in HPFS), loss to follow-up, or death, whichever came first. The cumulative average (mean) of olive oil intake from all available FFQs, from baseline until 2014 (or loss to follow-up or death), was used as the exposure. Because potential diet modifications following cancer or CVD diagnosis may not represent long-term diet, we ceased updating dietary variables upon report of these conditions. For missing covariates, we carried forward nonmissing values from previous questionnaires and assigned median values for continuous variables.
Participants were categorized by olive oil intake frequency: never or less than once per month (reference group), greater than 0 to less than or equal to 4.5 g/d, greater than 4.5 g/d to less than or equal to 7 g/d, and greater than 7 g/d. P values for linear trends were obtained using the Wald test on a continuous variable represented by the median intake of each category. Multivariable Cox proportional hazard models were used to estimate the hazard ratios (HRs) and 95% CIs for dementia mortality according to categories of olive oil intake, separately in each cohort. Participants were censored at death from causes other than dementia. Model 1 was stratified for age and calendar time. Multivariable model 2 was adjusted for Southern European/Mediterranean ancestry, married, living alone, smoking, alcohol intake, physical activity, multivitamin use, history of hypertension and hypercholesterolemia, in women postmenopausal status and menopausal hormone use, total energy intake, family history of dementia, history of depression, census SES, and BMI. Multivariable model 3 was further adjusted for intake of red meat, fruits and vegetables, nuts, soda, whole grains, and trans-fat, all indicative of diet quality.
In a secondary analysis we used the composite outcome for dementia-related deaths. We also repeated the main analysis in the genotyping subsample. We carried out mediation analyses to calculate the percentage of the association between olive oil intake and dementia-related mortality that is attributable to CVD, hypercholesterolemia, hypertension, and diabetes. We also performed stratified analyses by prespecified subgroups (eMethods in Supplement 1 ).
A joint analysis was performed to test whether olive oil intake (never or <1/mo, >0 to ≤7g/d, and >7g/d) and the AMED or the AHEI score (tertiles) combined as the exposure was associated with dementia mortality. In substitution analyses, we assessed the risk of dementia-related mortality by replacing 5 g/d of different fat sources, including margarine, mayonnaise, butter, and a combination of other vegetable oils (corn, safflower, soybean, and canola), with olive oil. Both continuous variables as 5-g/d increments were included in a multivariable model 3, mutually adjusted for other types of fat. The difference in the coefficients obtained for olive oil and the substituted fat provided the estimated HR and 95% CI for substituting 5 g/d of olive oil for an equivalent amount of the other fats.
Several exploratory sensitivity analyses were performed including a 4-year lagged analysis, analyses adjusting for other covariates, a cause-specific competing risk model and analyses excluding participants who self-reported having dementia at baseline (n = 12) (eMethods in Supplement 1 ). Analyses were performed from May 2022 to July 2023 using SAS version 9.4 (SAS Institute). All statistical tests were 2-sided with an α = .05.
Over 2 183 095 person-years of follow-up, this study documented a total of 4751 dementia deaths (3473 in NHS and 1278 in HPFS; 37 649 total deaths). Among 92 383 participants included at baseline in 1990, 60 582 (65.6%) were women, and the mean (SD) age was 56.4 (8.0) years. Mean (SD) olive oil intake was 1.3 (2.5) g/d in both NHS and HPFS; the mean (SD) adherence score for the Mediterranean diet was 4.5 (1.9) points in the NHS and 4.2 (1.9) points in the HPFS; and the mean (SD) AHEI diet quality score was 52.5 (11.1) points in the NHS and 53.4 (11.6) points in the HPFS.
Table 1 shows baseline characteristics of participants categorized by total olive oil intake. Participants with a higher olive oil intake (>7 g/d) at baseline had an overall higher caloric intake, but not a higher BMI, had better diet quality, had higher alcohol intake, were more physically active, and were less likely to smoke compared with those never consuming olive oil or less than once per month ( Table1 ). Individuals who were homozygous for the APOE ε4 allele were 5.5 to 9.4 times more likely to die with dementia compared with noncarriers for the APOE e4 allele (χ 2 P < .001) (eTable 1 in Supplement 1 ).
Olive oil intake was inversely associated with dementia-related mortality in age-stratified and multivariable-adjusted models ( Table 2 ). Compared with participants with the lowest olive oil intake, the pooled HR for dementia-related death among participants with the highest olive oil intake (>7 g/d) was 0.72 (95% CI, 0.64-0.81), after adjusting for sociodemographic and lifestyle factors. The association between each 5-g increment in olive oil consumption with dementia-related death was also inverse and significant in the pooled analysis. The multivariable-adjusted HR for dementia-related death for the highest compared with the lowest olive oil intake (>7 g/d) was 0.67 (95% CI, 0.59-0.77) for women and 0.87 (95% CI, 0.69-1.09) for men ( Table 2 ). Olive oil intake in 5-g increments was inversely associated with dementia-related mortality in women (HR, 0.88 [95% CI, 0.84-0.93]), but not in men (HR, 0.96 [95% CI, 0.88-1.04]). Analyses remained consistent when using the composite outcome for death with dementia (eTable 2 in Supplement 2 ). In the genotyping subsample, the results remained unchanged after further adjusting for the APOE ε4 allelic genotype (multivariable-adjusted pooled HR comparing high vs low olive oil intake, 0.66 [95% CI, 0.54-0.81]; P for trend < .001) (eTable 4 in Supplement 1 ). Pooled mediation analyses found that CVD, hypercholesterolemia, hypertension, and diabetes did not significantly attenuate the association (unchanged HRs with and without adjusting for the intermediate; data not shown).
In joint analyses, participants with the highest olive oil intake had a lower risk for dementia-related mortality, irrespective of their AMED score (28% to 34% lower risk compared with participants in the combined low olive oil and high AMED) ( Figure 1 A; eTable 3 in Supplement 1 ) and of their AHEI (27% to 38% lower risk compared with participants with low olive oil and high AHEI) ( Figure 1 B; eTable 3 in Supplement 1 ).
Replacing 5 g/d of mayonnaise with 5 g/d of olive oil was associated with a 14% (95% CI, 7%-20%) lower risk of dementia-related mortality in pooled multivariable-adjusted models ( Figure 2 ). As for the substitution of 5 g/d of margarine with the equivalent amount of olive oil, we estimated an 8% (95% CI, 4%-12%) lower risk. Substitutions of other vegetable oils or butter with olive oil were not statistically significant.
Exploratory subgroup analyses (eFigure in Supplement 1 ) showed associations between higher olive oil intake and lower risk of dementia-related mortality across most subgroups. No statistically significant associations were found in participants with a family history of dementia, living alone, using a multivitamin, and in non– APOE ε4 carriers. Results from exploratory sensitivity analyses (eTables 5-8 in Supplement 1 ) were comparable with the findings from the main analysis (eResults in Supplement 1 ).
In 2 large US prospective cohorts of men and women, we found that participants who consumed more than 7 g/d of olive oil had 28% lower risk of dying from dementia compared with participants who never or rarely consumed olive oil. This association remained significant after adjustment for diet quality scores including adherence to the Mediterranean diet. We estimated that substituting 5 g/d of margarine and mayonnaise with olive oil was associated with significantly lower dementia-related death risk, but not when substituting butter and other vegetable oils. These findings provide evidence to support dietary recommendations advocating for the use of olive oil and other vegetable oils as a potential strategy to maintain overall health and prevent dementia.
In the NHS and HPFS, a lower risk of neurodegenerative disease mortality, including dementia mortality, was observed with higher olive oil consumption (HR, 0.81 [95% CI, 0.78-0.84]). 11 Evidence that pertains to cognitive decline or incident dementia is more widely available than it is for dementia mortality. 6 , 22 In the French Three-City Study (n = 6947), participants with the highest olive oil intake were 17% (95% CI, 1%-29%) less likely to experience a 4-year cognitive decline related to visual memory, but no association was found for verbal fluency (odds ratio [OR], 0.85 [95% CI, 0.70-1.03]). 22 Furthermore, participants with a higher intake of olive oil (moderate or intensive vs never) had a lower risk of verbal fluency and visual memory cognitive impairment. Potential sex differences were not investigated. In the PREDIMED trial, which supplemented a Mediterranean-style diet with extra-virgin olive oil (1 L/wk/household) or nuts (30 g/d), 23 the authors investigated cognitive effects and status in 285 and 522 cognitively healthy participants using global and in-depth neuropsychological battery testing. Although the study was not originally designed for cognitive outcomes and the effect of olive oil cannot be isolated, after 6.5 years, the olive oil group exhibited improved cognitive performance in verbal fluency and memory tests compared with a low-fat diet (control), and they were less prone to develop mild cognitive impairment (OR, 0.34 [95% CI, 0.12-0.97]; n = 285). 6 Global cognitive performance was higher in both the olive oil and the nut groups compared with the control post trial (n = 522). 8 These studies were conducted in Europe, in populations with typically higher olive oil intake compared with US populations.
Observational studies and some trials have consistently found associations between following diets such as the Mediterranean, DASH, MIND, and AHEI, and prudent patterns to healthier brain structure, 24 reduced cognitive impairment and Alzheimer risk, and improved cognitive function. 4 In our study, those with the highest olive oil intake (>7 g/d) had the lowest dementia-related death risk compared with those with minimal intake (never or less than once per month), regardless of diet quality. This highlights a potentially specific role for olive oil. Still, the group with both high AHEI scores and high olive oil intake exhibited the lowest dementia mortality risk (HR, 0.68 [95% CI, 0.58-0.79]; reference: low AHEI score and low olive oil intake), suggesting that combining higher diet quality with higher olive oil intake may confer enhanced benefit.
Olive oil consumption may lower dementia mortality by improving vascular health. 18 Several clinical trials support the effect of olive oil in reducing CVD via improved endothelial function, coagulation, lipid metabolism, oxidative stress, platelet aggregation and decreased inflammation. 25 Nonetheless, the results of our study remained independent of hypertension and hypercholesterolemia. Mild cognitive impairment, Alzheimer disease, and related dementias were associated with abnormal blood brain barrier permeability, possibly allowing the crossing of neurotoxic molecules into the brain. 26 Mechanistical evidence from animal 27 - 29 and human studies 9 , 30 have shown that phenolic compounds in olive oil, particularly extra-virgin olive oil, may attenuate inflammation, oxidative stress and restore blood brain barrier function, thereby reducing brain amyloid-β and tau-related pathologies and improving cognitive function. However, incident CVD, hypercholesterolemia, hypertension, and diabetes were not significant mediators of the association between olive oil intake and dementia-related death in our study.
The association was significant in both sexes but did not remain in men after full adjustment of the model. Some previous research has reported cognitive-related sex differences. Evidence from trials also showed sex- and/or gender-specific responses to lifestyle interventions for preventing cognitive decline, possibly due to differences in brain structure, hormones (sex) and social factors (gender). 31 Olive oil intake may be protective of dementia and related mortality, particularly in women. Nonetheless, we did not observe significant heterogeneity or interaction of cohort by olive oil intake on the risk of fatal dementia. Sex and gender differences should be carefully considered in future studies examining the association or effect of olive oil on cognitive-related outcomes to improve our understanding.
We found that using olive oil instead of margarine and mayonnaise, but not butter and other vegetable oils, was associated with a lower risk of dementia-related death. At the time of the study, margarine and mayonnaise contained considerable levels of hydrogenated trans-fats. The latter were strongly associated with all-cause mortality, CVD, type 2 diabetes, and dementia, 32 , 33 which may explain the lower dementia-related death risk observed when replacing it with olive oil. The US Food and Drug Administration banned manufacturers from adding partially hydrogenated oils to foods in 2020. 34 Future studies examining intake of trans-fat–free margarine will be informative. Although the substitution of butter with olive oil was found to be associated with a lower risk of type 2 diabetes, CVD, and total mortality, 11 we did not find an association with the risk of dementia mortality. Although these previous studies did not examine the associations for butter per se, intake of regular fat dairy products, including cheese, yogurt, and milk, was reported to be either not associated or inversely associated with lower cognitive function, cognitive decline, and dementia. 35 - 37
Our cohort analyses include several strengths, namely the long follow-up period and large sample size with a high number of dementia death cases. Also, we included genotyping of the APOE ε4 allele in a large subsample of participants to reduce potential confounding attributed to this well-known risk factor for Alzheimer disease. Additionally, our repeated diet measurements, weight, and lifestyle variables permitted us to account for long-term olive oil intake and confounding factors. Furthermore, the use of dietary cumulative average updates reduced random measurement error by considering within-person variations in intake.
This study has limitations. The possibility of reverse causation cannot be excluded due to the observational nature of our study. However, the 4-year lagged analysis results, consistent with the primary analysis, suggest that olive oil intake is predictive of dementia mortality rather than a consequence of premorbid dementia. While it is plausible that higher olive oil intake could be indicative of a healthier diet and higher SES, our results remained consistent after accounting for the latter. Despite adjusting for key covariates, residual confounding may remain due to unmeasured factors. Also, our study was conducted among health professionals. While this minimizes the potential confounding effects of socioeconomic factors and likely increases reporting due to a high level of education, this may also limit generalizability. Our population was predominantly of non-Hispanic White participants, limiting generalizability to more diverse populations. Additionally, we could not differentiate among various types of olive oil that differ in their polyphenols and other nonlipid bioactive compounds content.
This study found that in US adults, particularly women, consuming more olive oil was associated with lower risk of dementia-related mortality, regardless of diet quality. Substituting olive oil intake for margarine and mayonnaise was associated with lower risk of dementia mortality and may be a potential strategy to improve longevity free of dementia. These findings extend the current dietary recommendations of choosing olive oil and other vegetable oils to the context of cognitive health and related mortality.
Accepted for Publication: March 6, 2024.
Published: May 6, 2024. doi:10.1001/jamanetworkopen.2024.10021
Open Access: This is an open access article distributed under the terms of the CC-BY License . © 2024 Tessier AJ et al. JAMA Network Open .
Corresponding Authors: Anne-Julie Tessier, RD, PhD ( [email protected] ), and Marta Guasch-Ferré, PhD ( [email protected] ), Department of Nutrition, Harvard T.H. Chan School of Public Health, 655 Huntington Ave, Bldg 2, Boston, MA 02115.
Author Contributions: Drs Tessier and Guasch-Ferré had full access to all of the data in the study and take responsibility for the integrity of the data and the accuracy of the data analysis.
Concept and design: Tessier, Chavarro, Hu, Willett, Guasch-Ferré.
Acquisition, analysis, or interpretation of data: Tessier, Cortese, Yuan, Bjornevik, Ascherio, Wang, Chavarro, Stampfer, Willett, Guasch-Ferré.
Drafting of the manuscript: Tessier.
Critical review of the manuscript for important intellectual content: Tessier, Cortese, Yuan, Bjornevik, Ascherio, Wang, Chavarro, Stampfer, Hu, Willett, Guasch-Ferré.
Statistical analysis: Tessier, Cortese, Wang, Willett, Guasch-Ferré.
Obtained funding: Chavarro, Stampfer, Hu, Guasch-Ferré.
Administrative, technical, or material support: Cortese, Yuan, Stampfer, Hu.
Supervision: Chavarro, Hu, Guasch-Ferré.
Conflict of Interest Disclosures: Dr Cortese reported a speaker honorarium from Roche outside the submitted work. Dr Ascherio reported receiving speaker honoraria from WebMD, Prada Foundation, Biogen, Moderna, Merck, Roche, and Glaxo-Smith-Kline. No other disclosures were reported.
Funding/Support: This study is supported by the research grant R21 AG070375 from the National Institutes of Health to Dr Guasch-Ferré. The NHS, NHSII and HPFS are supported by grants from the National Institutes of Health (UM1 CA186107, P01 CA87969, U01 CA167552, P30 DK046200, HL034594, HL088521, HL35464, HL60712). Dr Tessier is supported by the Canadian Institutes of Health Research (CIHR) Postdoctoral Fellowship Award. Dr Guasch-Ferré is supported the Novo Nordisk Foundation grant NNF23SA0084103.
Role of the Funder/Sponsor: The funders had no role in the design and conduct of the study; collection, management, analysis, and interpretation of the data; preparation, review, or approval of the manuscript; and decision to submit the manuscript for publication.
Data Sharing Statement: See Supplement 2 .
- Register for email alerts with links to free full-text articles
- Access PDFs of free articles
- Manage your interests
- Save searches and receive search alerts
- Homework Help
- Essay Examples
- Citation Generator
- Writing Guides
- Essay Title Generator
- Essay Topic Generator
- Essay Outline Generator
- Flashcard Generator
- Plagiarism Checker
- Paraphrasing Tool
- Conclusion Generator
- Thesis Statement Generator
- Introduction Generator
- Literature Review Generator
- Hypothesis Generator
- Editing Service
- Dementia Essays
Dementia Essays (Examples)
413+ documents containing “dementia” .


Filter by Keywords:(add comma between each)
Dementia and normal ageing old age comes.
Dementia and Normal Ageing Old age comes with quite a number of complications and change of behavior as well as physical changes. On the other hand, dementia also comes in with several symptoms that are closely related or similar to those displayed by people in old age. This makes it quite tricky for the family members of an ageing individual to tell whether the person is undergoing normal ageing or has been affected by dementia. This is made even harder to tell apart by the fact that dementia is more frequent among people of old age than the young people. The research paper looks at the two aspects and strives to contrast the two taking into account the biological, psychological, physiological as well as the sociological perspectives towards the two concepts. Normal ageing As we advance in years, the body gives way to several other signs that we did not have previously and….
Barry W. Roney et.al (2005). The Prevalence and Management of Dementia and Other
Psychiatric Disorders in Nursing Homes. International Psychogeriatrics-Cambridge Journals. Vol.2 Issue 1. Retrieved October 14, 2012 from http://journals.cambridge.org/action/displayAbstract?fromPage=online&aid=271878
Pieter J. Visser et.al, (1999:2). Medial temporal lobe atrophy and memory dysfunction as predictors for dementia in subjects with mild cognitive impairment. J Neurol. Vol.245. Pp478
Guo S. At al., (2012). Florbetaben PET in the Early Diagnosis of Alzheimer's Disease: A
Dementia There Are a Number
Since individuals who are included in those demographics normally will have less in resources that can be used to seek out treatments, the burden will fall on society. If an efficient and cost-effective treatment can be found then society, as well as the individuals affected would benefit greatly. orks Cited Akamine, D., Filho, M.K., Peres, C.M. (2007) Drug-nutrient Interactions in Elderly People, Current Opinion in Clinical Nutrition and Metabolic Care, Vol. 10, Issue 3, pp. 304-10 Dorey, Emma, (2007) Memory Loss Reversed in Mice (Alzheimer's Disease), Chemistry and Industry, Vol 11 Issue 1, Expanded Academic ASAP. Thomson Gale. Salt Lake City Public Library. Retrieved June 29, 2007 at http://0-find.galegroup.com.salty.slcpl.lib.ut.us:80/ips/infomark.do?&contentSet=IAC-Documents&type=retrieve&tabID=T003&prodId=IPS&docId=A165692154&source=gale&srcprod=EAIM&userGroupName=slpl&version=1.0 Husain, M.M., Garrett, R.K., (2005) Clinical Diagnosis and Management of Alzheimer's Disease, Neuroimaging Clinics of North America, Vol. 15, Issue 4, pp 767-77 Kawas, Claudia (2003) Early Alzheimer's Disease, New England Journal of Medicine, Vol. 349 pp 1056-1063 Levine, Robert (2006) Defying Dementia: Understanding and Preventing….
Works Cited
Lin, P.W., Chan, W.C., Ng, B.F., Lam, L.C. (2007) Efficacy of Aromatherapy (Lavandula Angustifolia) as an Intervention for Agitated Behaviours in Chinese Older Persons with Dementia: a cross-over randomized trial, International Journal of Geriatric Psychiatry, Vol. 22, Issue 5, pp 405-10
Dementia Deficiency of Mental Ability
(Transforming Care for People with Dementia across the Continuum) Dementia can be cured with a mixture of psychotherapy, environmental modifications, and medicines. Drug treatment can be problematic by forgetfulness, mainly if the advised drug is to be taken a number of times a day. Behavioral problems are due to irritation or over stimulation. Enjoyable activities, like crafts, games, and music, can give therapeutic motivation and enhance mood. Changing the environment can increase protection and consolation while lessening tension. (What's the treatment for dementia?) Often head injuries bring a sudden coping crisis. The sudden unpleasant changes that go with a head injury certainly cause many emotions. The injured individual's atmosphere must be neither too quiet nor too confused. The atmosphere must be made secure by taking away area mats to decrease falls, removing danger, giving tub bars, and putting child locks on cabinets or stove knobs if required. If the patient is….
Behavioral Management in Persons with Dementia. Retrieved at http://www.info.gov.hk/elderly/english/healthinfo/elderly/behavioralmanagement-e.htm . Accessed on 26 June 2005
Braun, Mark. E; Braun, Ursula, K. (December, 2004) Behavioral disturbances in dementia: finding the cause" Geriatrics. Retrieved at http://www.findarticles.com/p/articles/mi_m2578/is_12_59/ai_n9538490Accessed on 26 June 2005.
Byrnes, Glenn. "Dealing with Dementia: Help for Relatives, Friends and Caregivers" Retrieved at http://www.ncpamd.com/dementia.htm . Accessed on 26 June 2005
Dementia in Head Injury. Retrieved at http://www.emedicinehealth.com/articles/38577-6.asp . Accessed on 26 June 2005.
Dementia Training the Most Frequent
(Alzheimer's Society, 2007). As affirmed that fifty five staff members were presented at all four sittings of the certificate training. Members who focused on all four sessions were alike to those who did not, in age, sum of years finished in school, employee's position and other individuality. As predictable, those who worked most to all of their time on the individual care unit were more probable to complete all four components. However, unpredictably, those who had no previous training were less likely to go to for all four than those who had some training. Training members were asked their view on twenty eight statements related to dementia and caring for people with dementia, together with such statements as spending time with people with dementia can be very enjoyable, good dementia care involves caring for a person's emotional needs as well as their physical needs, family members can provide valuable information….
Bibliography
An evaluation of Dementia-Specific Training for Professionals. Raising the Bar: An evaluation of Dementia-Specific Training for Professionals. Retrieved on December 26, 2007 at http://www.slu.edu/colleges/SOCSVC/CtrSocJustice/Raising%20the%20bar%20report%20v.7pdf.pdf
MERCK. (February 2003). Dementia. Retrieved on December 26, 2007 at http://www.merck.com/mmhe/sec06/ch083/ch083c.html
Alzheimer's Society. (2007). Home From Home: A Report Highlighting Opportunities for Improving Standards of Dementia Care in Care Homes. Retrieved on December 26, 2007 at http://www.alzheimers.org.uk
Dementia Alzheimer's Disease Is a Type of
Dementia Alzheimer's disease is a type of dementia, of which there are many types. Dementia is a term that describes a number of conditions that lead to a loss of intellectual capacities but initially occurring with clear consciousness. The area most often first affected is memory; however, with some types of dementia this may not be the case. The DSM-IV-T diagnostic criteria for dementia consist of memory loss and the loss of one other cognitive domain. The disorder must result in significant impairment or distress either social, occupational or some other area of the person's functioning and must also constitute a marked change from the person's prior functioning (American Psychiatric Association [APA], 2000). Alzheimer's Disease (AD) Alzheimer's disease (AD) accounts for majority of dementia cases (50-60%) followed by vascular dementia (between 15-30%). Other common forms of dementia include Lewy body dementia and dementia associated with Parkinson's disease, but there are many different causes….
American Psychiatric Association. (2000). Diagnostic and Statistical Manual of Mental
Disorders- 4th edition- Text Revision. Washington, D.C.: American Psychiatric Association
Launer, L.J., Andersen, K., Dewey, M.E., & Letenneur, L. (1999). Rates and risk factors for dementia and Alzheimer's disease: Results from EURODEM pooled analyses. Neurology, 52, 78-84.
Miller, B.L. & Boeve, B.F. (2009). The Behavioral Neurology of Dementia. New York:
Dementia What Happens to a Family When
Dementia hat happens to a family when one member -- the husband -- slides into the terrible and mysterious illness called frontotemporal dementia? How does his wife deal with his disease? An article in The New York Times delves deeply into the life of a New York engineer named Michael French, who began acting in bizarre, unexplainable ways in his 50s, and now, at 71, can no longer speak, write, read or walk. This paper reviews that article and reports on recommended caregiving strategies for patients with dementia. A Rare Form of Dementia Tests a Vow of "For Better, for orse" Michael French began acting a little weird when he was in his 50s, about ten years before he was diagnosed with the rare form of dementia noted in the introduction, according to his wife Ruth. This was totally out of character because Michael had run marathons, lectured at conventions, did volunteer work,….
Family Caregiver Alliance. (2011). Fact Sheet: Caregiver's Guide to Understanding Dementia
Behaviors. Retrieved May 20, 2012, from http://www.caregiver.org .
Grady, Denise. (2012). When Illness Makes a Spouse a Stranger. The New York Times.
Retrieved May 20, 2012, from http://www.nytimes.com .
Dementia Case Study Review A Basis for
Dementia Case Study Review: A basis for interpersonal Practice Communication is the process of conveying opinions and exchanging ideas in a way which enhances the interpersonal relationship between individuals. It is a skill that enables us to share ideas and solve the problems that people encounter in their everyday life to live meaningfully. Communication is verbal and non- verbal and everything that anyone does is part of communication and conveys some impression to another party. It helps in connecting individuals and improving the relationship between people in any situation. Good interpersonal skills makes it easy for feelings, thoughts and needs of others to be known by others as they are listened to and understood in the best way. (Koprowska, 2008) Larry is experiencing the consequences of poor communication. The inability to share information with his family or neighbours in the belief that they will avoid his wife is based on the perception of….
However, we can not forget that the care workers also have rights. Under the Carers (Equal Opportunities) Act 2004, care givers are to be able to have access to training, work and leisure opportunities just as any other worker has the right to. The Act requires that employers provide job assessments be offered to care givers, that they consider the needs of care givers with regard to leisure, education, training and work. Not all care givers will wish to pursue all of the above options. However, practitioners completing assessments with the caregiver should be able to signpost them to other relevant agencies (Legislation.gov.uk 2004).
Refer to (IR (ME)R 2000) (Ionisation Radiation Regulations)
Caregivers, especially live-in and full-time caregivers, are very frequently exposed to the same environmental conditions. These can include x-ray and other equipment that gives out hazardous radiations in both medical and dental environments, as well as radioactive wastes. The Medical Exposures Directive requires that all medical exposures to ionising radiation have to be justified prior to the exposure being made. This Directive refers to two levels of justification; 1) justification
Dementia an Inevitable Part of the Aging
Dementia an Inevitable Part of the Aging Process? Dementia is a chronic and usually progressive deterioration of mental abilities and intellectual capacity due to changes in the brain such as widespread loss of nerve cells and the shrinkage of brain tissue. Since dementia is most commonly seen in the elderly, it is often erroneously considered to be part of the normal aging process. However, it is important to remember that dementia is a condition that is a result of a disease (or trauma) and does not occur in a healthy person simply because of old age. In this essay I shall discuss why dementia is not an inevitable part of the aging process; describe the types of dementia and the ways in which they affect the cognitive functions of the afflicted person; the importance of diagnosing dementia and the role of the cognitive psychologist in diagnosis; and how people with….
"Dementia" (2004). Neurology Channel. Retrieved on February 12, 2005 from http://www.neurologychannel.com/dementia / 'Forgetfulness: It's Not Always What You Think." (2004). National Institute on Aging:
U.S. Department of Health and Human Services. Retrieved on February 12, 2005 from http://www.niapublications.org/engagepages/forgetfulness.asp
"Guidelines for the Evaluation of Dementia and Age-Related Cognitive Decline." (1998).
American Psychological Association. Retrieved on February 12, 2005 from http://www.apa.org/practice/dementia.html
Dementia A Growing and Serious Psychological Issue
dementia disorder and how healthcare professionals embrace theories, research and practical strategies, in order to help dementia sufferers cope with their difficulties. Behavioral and Psychological symptoms in primary care Doctors, nurses, other healthcare professionals and caregivers are challenged when it comes to the care of patients suffering from dementia. That is because dementia is frequently accompanied by a variety of neuropsychiatric symptoms, according to a peer-reviewed article in the journal Mental Health in Family Medicine. Those symptoms include "disturbed behavior, thought, mood and perception" (Buhagiar, et al., 2011). The point of the article was to find out how much confidence that doctors (general practitioners) have as regards their ability to identify and manage the various behavioral and psychological symptoms of dementia. The authors designed a two-page questionnaire and sent it to 160 general practitioners in north Dublin, Ireland. About 109 general practitioners returned the questionnaires (that is a 68% rate of response),….
Buhagiar, K., Arzal, N., and Cosgrave, M. (2011). Behavioral and psychological symptoms
Of dementia in primary care: a survey of general practitioners in Ireland. Mental Health in Family Medicine, Volume 8, 227-234.
Nordgren, L., and Engstrom, G. (2014). Effects of dog-assisted intervention on behavioral and psychological symptoms of dementia. Nursing Older People, 26(3), 31-38.
Ragdale, S. (2014). Dementia and its relationship with food. British Journal of Community
Dementia Inevitable or Preventable
Introduction Dementia is a degenerative cognitive health issue that primarily affects the elderly population and is characterized by “impairments in cognitive and intellectual ability, memory, language, reasoning, and judgment,” all of which interfere with the individual’s ability to function in everyday life (Agency for Healthcare Research and Quality, 2014). The two most common forms of dementia are Alzheimer’s Disease and vascular dementia (Livingston et al., 2017). While not much is known about the etiology of dementia other than that it is related to cellular damage in the brain, there has been some success in identifying possible ways to prevent it. This paper will define dementia, its manifestations and types, discuss three research articles that deal with preventing dementia, and provide suggestions for future research on this topic. Defining Dementia Dementia is a neurodegenerative disorder that results in the irreversible loss of brain functionality. Alzheimer’s Disease is the most common form and results from….
Dementia Prescribing for Older Adults
Prescribing for Older AdultsSome psychiatric disorders are more prevalent in older generations as compared to the younger generations. This is due to cerebral-neural degeneration. Often, these disorders are not recognized in good time and are thus not treated properly despite their prevalence. Mental disorders in general can lead to disability, suicide or even a poor quality of life. In this section, I will discuss one of the disorders from the DSM-5 that affect older adults.DementiaDementia is a disorder that is characterized by inability to make sound decisions, think, or even remember crucial aspects that were easy to recall earlier on. Many people may think that dementia is a normal stage in the ageing process but it is not the case. The early signs of dementia include: forgetting to take a meal or even the process of making that meal, getting disoriented whereby the person suffering from this disorder tends to….
Centers for Disease Control and Prevention – CDC (2019). Alzheimer’s disease and Healthy Aging. https://www.cdc.gov/aging/index.html
Hugo, J. & Ganguli, M. (2014). Dementia and Cognitive Impairment. Epidemiology, Diagnosis, and Treatment, 30(3), 421-442.
Westphalen, D. & Pharm. D. (2019). A List of Common Dementia Medications. https://www.healthline.com/health/dementia-drugs-and-medication
dementia care evidence based practice
The Alzheimer’s Association (2018) offers healthcare administrators and practitioners a set of recommendations for dementia care. These evidence-based practice guidelines and protocols are designed to improve overall standards and quality of care, and also to help standardize and regulate care across the healthcare spectrum. The patient population targeted in the Alzheimer’s Association (2018) dementia care practice recommendations includes all individuals with dementia, and includes input from a wide range of research areas and areas of specialization within gerontology. Furthermore, the care guidelines are written in accordance with evidence published in associated peer-reviewed journals like The Gerontologist. For example, Hirschman & Hodgson (2018) found seven evidence-based interventions that are specifically designed to promote best practices including education, communication, and inter-professional team building. The Alzheimer’s Association (2018) guidelines use criteria related to these and similar care objectives, focusing on factors like care transition, long-term care goals, workforce implications, informational and psychosocial supports….
Dementia and the Elderly
Alzheimer's disease afflicts more than 16 million individuals in the United States, but the etiology of this condition remains unclear (Sullivan, 2010). What is known is that Alzheimer's affects black Americans twice as often as whites and Hispanics 1.5 times as often, and this discrepancy is believed to be attributed to lower socioeconomic status and higher rates of chronic illness among minority populations (Sullivan, 2010). The case study in question concerns a retired postal worker, "Gene," who is a 100% disabled 73-year-old African-American veteran living in Cleveland. In 2011, Gene's wife of 50 years, "Shirley," died leaving him living alone for the first time in his adult life. At the time of Shirley's death, Gene had been diagnosed with early stage dementia but had been able to remain active and living independently for a period of one year in a long-term care retirement center. During his military service in….
Sullivan, M.G. (2010, April). Alzheimer's disease risk in blacks is twice that of whites. Clinical Psychiatry News, 38(4), 3.
Zimmerman, S.I. & Sloane, P.D. (2009, Fall). Optimum residential care for people with dementia. Generations, 23(3), 62-69.
Ability of an Individual With Dementia
Dementia is not a disease that is distinct and particular. ather, dementia encompasses a disease that is collective of symptoms that have an impact on memory, thinking as well as social capabilities in a rigorous and severe manner to the extent that it hampers with functioning on an everyday basis. Dementia points toward issues with at least two functionalities of the brain such as loss of memory and impaired decision making or language and the incapacity to undertake a number of daily activities such as getting lost while walking or driving and even incapability to pay bills (Dementia). However, it is imperative to note that in as much as memory loss is a part of dementia it does not necessarily imply that having memory loss alone is suffering from dementia. Memory loss to some extent is part and parcel of old-age or advanced years. The symptoms of dementia differ with regards….
Dementia - Treatment Overview. (n.d.). Retrieved March 21, 2015, from http://www.webmd.com/alzheimers/tc/dementia-treatment-overview
Dementia. (n.d.). Retrieved March 21, 2015, from http://www.mayoclinic.org/diseases-conditions/dementia/basics/risk-factors/con-20034399
Memory Loss and Dementia. (n.d.). Retrieved March 21, 2015, from http://www.patient.co.uk/health/Memory-Loss-and-Dementia
Hardy, M. (n.d.). Ways to Help Dementia. Retrieved March 21, 2015, from http://www.ehow.com/way_5645230_ways-dementia.html
Unlicensed Caregiver Experience in Dementia Care Dementia
Unlicensed Caregiver Experience in Dementia Care Dementia Care Nearly 5.4 million people in the United States suffer from Alzheimer's disease, the most common form of dementia (Chodosh et al., 2012, p. 85). The health care burden this places on nursing homes, assisted living facilities, and hospices is significant, especially since some dementia sufferers exhibit disruptive and sometimes dangerous behaviors. These behaviors include verbal and physical aggression, wandering, restlessness, insomnia, irritability, and repetitive vocalizations (McKenzie, Teri, Pike, LaFazia, and van Leynseele, 2012, p. 96). Nearly 1 million Americans currently reside in assisted living facilities and an estimated 45 to 67% suffer from dementia. Of these, 34 to 56% exhibit behavioral problems. The impact of dementia care on professional caregivers is therefore significant, especially when dealing with patients with more advanced forms of the disease. Early studies suggest that dementia care training can have a positive impact on both the patient's health and the care….
Chodosh, Joshua, Pearson, Marjorie L., Connor, Karen I., Vassar, Stefanie D., Kaisey, Marwa, Lee, Martin L. et al. (2012). A dementia care management intervention: Which components improve quality? American Journal of Managed Care, 18, 85-94.
McKenzie, Glenise, Teri, Linda, Pike, Kenneth, LaFazia, David, and van Leynseele, June. (2012). Reactions of assisted living staff to behavioral and psychological symptoms of dementia. Geriatric Nursing, 33, 96-104.
Ragin, C.C., Nagel, J., and White, P. (2004). Workshop on Scientific Foundations of Qualitative Research. Washington, DC: National Science Foundation. Retrieved 3 May, 2012 from http://www.nsf.gov/pubs/2004/nsf04219/nsf04219.pdf .
I am having to write a research paper on traumatic brain injuries, and I have no idea what direction I want to go with it.
Traumatic brain injuries , or TBIs as they often called, are injuries to the brain that are caused by outside sources, such as a fall or a blow to the head. This differentiates them from brain injuries that are caused by internal situations, such as a stroke. TBIs include a broad group of different types of injuries, from seemingly minor concussions to injuries that clearly and significantly impact the ability to function. Because they are so varied, there are many different directions you could take in a research paper about TBIs. To help you decide what direction....
Can you help me with some resources and bibliography for essay on assisted living
Example Bibliography for an essay on assisted living.
Writing about assisted living facilities can be challenging, because many of the sources that you will find online will be advertising for assisted living , rather than factual and informative pieces. An assisted living facility is a housing facility for people who cannot or choose not to live independently. They can range from facilities where there is a minimum amount of help to full-care nursing facilities. In fact, many facilities offer a range of housing options, which are adaptable as a person’s ability to live independently....
Do you have any tips for outlining an essay specifically on the subject of forming meaningful connections may enrich lives
Outline for an Essay on: Forming Meaningful Connections Enriches Lives I. Introduction A. Hook: Begin with a compelling anecdote or statistic that highlights the importance of meaningful connections. B. Thesis Statement: Clearly state the central argument that meaningful connections enrich lives. II. Personal Fulfillment A. Emotional Well-being: Discuss how meaningful connections provide emotional support, reduce stress, and promote happiness. B. Purpose and Meaning: Explain how relationships give individuals a sense of purpose and meaning in life. C. Personal Growth: Describe how interacting with diverse individuals fosters personal growth and self-discovery. III. Social Benefits A. Reduced Loneliness and Isolation: Emphasize the role of meaningful....
I\'ve seen the common essay topics on type 2 diabetese. Any lesser-known but interesting ones you can recommend?
Lesser-Known but Intriguing Essay Topics on Type 2 Diabetes 1. The Interplay between Gut Microbiota and Type 2 Diabetes Explore the role of gut microorganisms in the development and progression of type 2 diabetes. Discuss the potential for modulating gut microbiota as a therapeutic approach. Examine the impact of diet, prebiotics, and probiotics on gut health in relation to type 2 diabetes. 2. Precision Medicine for Type 2 Diabetes Management Analyze the application of genetic testing and biomarkers to personalize treatment strategies. Discuss the role of pharmacogenomics in tailoring medication selection and dosage. Explore the ethical and practical considerations of using precision....
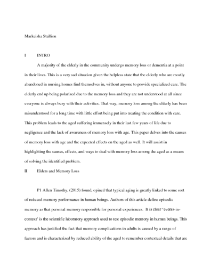
Death and Dying (general)
Dementia and Normal Ageing Old age comes with quite a number of complications and change of behavior as well as physical changes. On the other hand, dementia also comes in…
Since individuals who are included in those demographics normally will have less in resources that can be used to seek out treatments, the burden will fall on society.…
(Transforming Care for People with Dementia across the Continuum) Dementia can be cured with a mixture of psychotherapy, environmental modifications, and medicines. Drug treatment can be problematic by forgetfulness,…
(Alzheimer's Society, 2007). As affirmed that fifty five staff members were presented at all four sittings of the certificate training. Members who focused on all four sessions were alike…
Dementia Alzheimer's disease is a type of dementia, of which there are many types. Dementia is a term that describes a number of conditions that lead to a loss of…
Dementia hat happens to a family when one member -- the husband -- slides into the terrible and mysterious illness called frontotemporal dementia? How does his wife deal with his…
Family and Marriage
Dementia Case Study Review: A basis for interpersonal Practice Communication is the process of conveying opinions and exchanging ideas in a way which enhances the interpersonal relationship between individuals. It is…
Dementia an Inevitable Part of the Aging Process? Dementia is a chronic and usually progressive deterioration of mental abilities and intellectual capacity due to changes in the brain such…
dementia disorder and how healthcare professionals embrace theories, research and practical strategies, in order to help dementia sufferers cope with their difficulties. Behavioral and Psychological symptoms in primary care Doctors,…
Research Paper
Introduction Dementia is a degenerative cognitive health issue that primarily affects the elderly population and is characterized by “impairments in cognitive and intellectual ability, memory, language, reasoning, and judgment,” all…
Prescribing for Older AdultsSome psychiatric disorders are more prevalent in older generations as compared to the younger generations. This is due to cerebral-neural degeneration. Often, these disorders are not…
The Alzheimer’s Association (2018) offers healthcare administrators and practitioners a set of recommendations for dementia care. These evidence-based practice guidelines and protocols are designed to improve overall standards and…
Alzheimer's disease afflicts more than 16 million individuals in the United States, but the etiology of this condition remains unclear (Sullivan, 2010). What is known is that Alzheimer's…
Dementia is not a disease that is distinct and particular. ather, dementia encompasses a disease that is collective of symptoms that have an impact on memory, thinking as well…
Unlicensed Caregiver Experience in Dementia Care Dementia Care Nearly 5.4 million people in the United States suffer from Alzheimer's disease, the most common form of dementia (Chodosh et al., 2012, p.…
- Share full article
Advertisement
Supported by
Study Suggests Genetics as a Cause, Not Just a Risk, for Some Alzheimer’s
People with two copies of the gene variant APOE4 are almost certain to get Alzheimer’s, say researchers, who proposed a framework under which such patients could be diagnosed years before symptoms.

By Pam Belluck
Scientists are proposing a new way of understanding the genetics of Alzheimer’s that would mean that up to a fifth of patients would be considered to have a genetically caused form of the disease.
Currently, the vast majority of Alzheimer’s cases do not have a clearly identified cause. The new designation, proposed in a study published Monday, could broaden the scope of efforts to develop treatments, including gene therapy, and affect the design of clinical trials.
It could also mean that hundreds of thousands of people in the United States alone could, if they chose, receive a diagnosis of Alzheimer’s before developing any symptoms of cognitive decline, although there currently are no treatments for people at that stage.
The new classification would make this type of Alzheimer’s one of the most common genetic disorders in the world, medical experts said.
“This reconceptualization that we’re proposing affects not a small minority of people,” said Dr. Juan Fortea, an author of the study and the director of the Sant Pau Memory Unit in Barcelona, Spain. “Sometimes we say that we don’t know the cause of Alzheimer’s disease,” but, he said, this would mean that about 15 to 20 percent of cases “can be tracked back to a cause, and the cause is in the genes.”
The idea involves a gene variant called APOE4. Scientists have long known that inheriting one copy of the variant increases the risk of developing Alzheimer’s, and that people with two copies, inherited from each parent, have vastly increased risk.
The new study , published in the journal Nature Medicine, analyzed data from over 500 people with two copies of APOE4, a significantly larger pool than in previous studies. The researchers found that almost all of those patients developed the biological pathology of Alzheimer’s, and the authors say that two copies of APOE4 should now be considered a cause of Alzheimer’s — not simply a risk factor.
The patients also developed Alzheimer’s pathology relatively young, the study found. By age 55, over 95 percent had biological markers associated with the disease. By 65, almost all had abnormal levels of a protein called amyloid that forms plaques in the brain, a hallmark of Alzheimer’s. And many started developing symptoms of cognitive decline at age 65, younger than most people without the APOE4 variant.
“The critical thing is that these individuals are often symptomatic 10 years earlier than other forms of Alzheimer’s disease,” said Dr. Reisa Sperling, a neurologist at Mass General Brigham in Boston and an author of the study.
She added, “By the time they are picked up and clinically diagnosed, because they’re often younger, they have more pathology.”
People with two copies, known as APOE4 homozygotes, make up 2 to 3 percent of the general population, but are an estimated 15 to 20 percent of people with Alzheimer’s dementia, experts said. People with one copy make up about 15 to 25 percent of the general population, and about 50 percent of Alzheimer’s dementia patients.
The most common variant is called APOE3, which seems to have a neutral effect on Alzheimer’s risk. About 75 percent of the general population has one copy of APOE3, and more than half of the general population has two copies.
Alzheimer’s experts not involved in the study said classifying the two-copy condition as genetically determined Alzheimer’s could have significant implications, including encouraging drug development beyond the field’s recent major focus on treatments that target and reduce amyloid.
Dr. Samuel Gandy, an Alzheimer’s researcher at Mount Sinai in New York, who was not involved in the study, said that patients with two copies of APOE4 faced much higher safety risks from anti-amyloid drugs.
When the Food and Drug Administration approved the anti-amyloid drug Leqembi last year, it required a black-box warning on the label saying that the medication can cause “serious and life-threatening events” such as swelling and bleeding in the brain, especially for people with two copies of APOE4. Some treatment centers decided not to offer Leqembi, an intravenous infusion, to such patients.
Dr. Gandy and other experts said that classifying these patients as having a distinct genetic form of Alzheimer’s would galvanize interest in developing drugs that are safe and effective for them and add urgency to current efforts to prevent cognitive decline in people who do not yet have symptoms.
“Rather than say we have nothing for you, let’s look for a trial,” Dr. Gandy said, adding that such patients should be included in trials at younger ages, given how early their pathology starts.
Besides trying to develop drugs, some researchers are exploring gene editing to transform APOE4 into a variant called APOE2, which appears to protect against Alzheimer’s. Another gene-therapy approach being studied involves injecting APOE2 into patients’ brains.
The new study had some limitations, including a lack of diversity that might make the findings less generalizable. Most patients in the study had European ancestry. While two copies of APOE4 also greatly increase Alzheimer’s risk in other ethnicities, the risk levels differ, said Dr. Michael Greicius, a neurologist at Stanford University School of Medicine who was not involved in the research.
“One important argument against their interpretation is that the risk of Alzheimer’s disease in APOE4 homozygotes varies substantially across different genetic ancestries,” said Dr. Greicius, who cowrote a study that found that white people with two copies of APOE4 had 13 times the risk of white people with two copies of APOE3, while Black people with two copies of APOE4 had 6.5 times the risk of Black people with two copies of APOE3.
“This has critical implications when counseling patients about their ancestry-informed genetic risk for Alzheimer’s disease,” he said, “and it also speaks to some yet-to-be-discovered genetics and biology that presumably drive this massive difference in risk.”
Under the current genetic understanding of Alzheimer’s, less than 2 percent of cases are considered genetically caused. Some of those patients inherited a mutation in one of three genes and can develop symptoms as early as their 30s or 40s. Others are people with Down syndrome, who have three copies of a chromosome containing a protein that often leads to what is called Down syndrome-associated Alzheimer’s disease .
Dr. Sperling said the genetic alterations in those cases are believed to fuel buildup of amyloid, while APOE4 is believed to interfere with clearing amyloid buildup.
Under the researchers’ proposal, having one copy of APOE4 would continue to be considered a risk factor, not enough to cause Alzheimer’s, Dr. Fortea said. It is unusual for diseases to follow that genetic pattern, called “semidominance,” with two copies of a variant causing the disease, but one copy only increasing risk, experts said.
The new recommendation will prompt questions about whether people should get tested to determine if they have the APOE4 variant.
Dr. Greicius said that until there were treatments for people with two copies of APOE4 or trials of therapies to prevent them from developing dementia, “My recommendation is if you don’t have symptoms, you should definitely not figure out your APOE status.”
He added, “It will only cause grief at this point.”
Finding ways to help these patients cannot come soon enough, Dr. Sperling said, adding, “These individuals are desperate, they’ve seen it in both of their parents often and really need therapies.”
Pam Belluck is a health and science reporter, covering a range of subjects, including reproductive health, long Covid, brain science, neurological disorders, mental health and genetics. More about Pam Belluck
The Fight Against Alzheimer’s Disease
Alzheimer’s is the most common form of dementia, but much remains unknown about this daunting disease..
How is Alzheimer’s diagnosed? What causes Alzheimer’s? We answered some common questions .
A study suggests that genetics can be a cause of Alzheimer’s , not just a risk, raising the prospect of diagnosis years before symptoms appear.
Determining whether someone has Alzheimer’s usually requires an extended diagnostic process . But new criteria could lead to a diagnosis on the basis of a simple blood test .
The F.D.A. has given full approval to the Alzheimer’s drug Leqembi. Here is what to know about i t.
Alzheimer’s can make communicating difficult. We asked experts for tips on how to talk to someone with the disease .

An official website of the United States government
The .gov means it’s official. Federal government websites often end in .gov or .mil. Before sharing sensitive information, make sure you’re on a federal government site.
The site is secure. The https:// ensures that you are connecting to the official website and that any information you provide is encrypted and transmitted securely.
- Publications
- Account settings
Preview improvements coming to the PMC website in October 2024. Learn More or Try it out now .
- Advanced Search
- Journal List
- Palliat Care Soc Pract
A UK qualitative study of living and dying with dementia in the last year of life
Jacqueline crowther.
Academic Palliative and Supportive Care Studies Group (APSCSG) and Primary Care and Mental Health, University of Liverpool, Liverpool, UK
Siobhan Horton
St Luke’s Hospice, Winsford, UK
Kenneth Wilson
Department of Psychiatry, University of Liverpool, Liverpool, UK
Mari Lloyd-Williams
Professor, Primary Care and Mental Health, Liverpool Health Partners and University of Liverpool, Brownlow Hill, Liverpool L69 3GB, UK
Background:
Dementia is a life-limiting illness, but the trajectory of dying can be difficult to establish and care at end of life can be variable and problematic.
This UK study was carried out to explore the end-of-life-care experiences of people with dementia from the perspective of their family carers. In-depth interviews were conducted with 40 bereaved family carers of people with dementia.
Forty family carers (male n = 9, female n = 31) age range: 18–86 years were interviewed. Issues with poor communication were common. The hard work of caring and issues regarding unpredictability of living and dying with dementia were also commonplace within the study. Only three patients were referred for specialist palliative care support at the end of life, all of whom had a dual diagnosis of dementia and cancer.
Conclusion:
This qualitative study has identified that there are several gaps in the end-of-life care of people with dementia, and frequently, there is poor communication during the last year of life. The need for high-quality integrated care for people dying with dementia with appropriate support during the last year of life is identified. COVID-19 has disproportionately affected people with dementia, and in the post-pandemic era, there is an urgent need to ensure every person dying with dementia is supported to die in their preferred place and that family members are supported and enabled to be treated as the ‘expert’ in terms of their knowledge of their relatives’ care and preferences.
What is already Known About the topic
- Dementia is a life-limiting illness, but the diagnosis of dying can be difficult.
- Many patients die with dementia in hospital or in care homes.
What this paper adds
- This qualitative study of family carers revealed that communication with the person with dementia and also with professionals was a major issue.
- Family carers felt worn down by the constant ‘battles’ they perceived they experienced in negotiating the healthcare system on behalf of the person with dementia.
- Dying of dementia was traumatic for family carers of those interviewed with little support and privacy and frequently no support from specialist palliative care.
Implications for practice theory or policy
- This study suggests that specialist or generalist palliative care is frequently not available for those with dementia dying in hospital or in care homes.
- Sensitive communication and respect for family carers is a basic necessity of good end-of-life care.
- Where advance care plans are not in place, family carers should be consulted and considered within the clinical decision-making process
Introduction
It is estimated that there are approximately 820,000 people with dementia in the United Kingdom, 1 and the incidence and prevalence of dementia will continue to rise with an ageing population. It is predicted that deaths from dementia in the United Kingdom will increase from around 59,000 per year in 2014 to around 220,000 per year by 2040. 2
Research suggests that people with dementia receive poorer end-of-life care, 3 – 5 inadequate pain relief, and limited access to palliative and hospice care. 6 – 9 Studies exploring end-of-life care for people with dementia have focused mainly on 24-h care environments, that is, hospital, nursing, and residential care, and frequently, the focus of research has been with paid carers and their understanding and approach to care towards the end of life. There is relatively little research and literature exploring personal experiences of family carers who have cared for a loved one with dementia up to and including death. Dementia is a challenging illness, and in the later stages, there is not only cognitive deterioration but also physical deterioration in terms of mobility, issues with nutrition, faecal and urinary incontinence, and in the later stages becoming bedfast.
In a study to explore decision-making at the end of life, interviewing carers and professionals, uncertainty and poor communication were reported as the main areas of difficulty. 10 A further qualitative study of professionals, along with people with dementia and family carers, reported seven key areas namely timely planning of discussions around end of life; recognition of patient being at the end of life and provision of supportive care; co-ordination of care; effective working relationships with primary-care teams; managing hospitalisation; continuing care after death; and valuing staff and ongoing learning. 11
Longitudinal studies are particularly valuable to capture experiences at different time points and a study from the Netherlands 12 to determine what should be available to enable good palliative care for people with dementia at the end of life, interviewed 10 family carers of people with dementia in focus groups at three time points. Family carers wanted familiarity at the end of life of having familiar people caring for the person with dementia and also wished care to be at home if at all possible and communication with professionals that they knew and trusted.
The aim of our study and this paper was to explore the direct personal experiences of a diverse group of family carers who had cared for people with dementia in the last year of life and up to death.
We report additional analysis of our national study 4 which recruited bereaved family carers from Scotland, England, and Wales. Participants were recruited via organizations, for example, Alzheimer’s Society and Age UK, social media, and community networks in order to obtain a broad range of experiences of care during the last year of life, and this paper reports additional analysis from the original study. As data collection and analysis progressed recruitment became more focused on certain under represented groups and locations, that is, male carers, semi-rural and rural areas. To facilitate this process, organisations such as the Alzheimer’s Society, Age UK, and Alzheimer’s Scotland were contacted and invited to publicise the appeal through newsletters and carers groups held within local branches. Full ethical approval from University of Liverpool ethics committee was obtained (RETH000206). Inclusion criteria included unpaid carer of someone with dementia in their last year of life and at the time of death; aged 18 years or over; death to have occurred within the last 5 years in any care environment (family home, care home, and hospital). Exclusion criteria included paid/formal carers; under 18 years of age; death occurred more than 5 years previously.
Family carers were invited to contact the first author if they were interested in participating in the study and were sent an information pack which included an information sheet and reply letter to invite the first author to contact them. Following written consent being obtained, interviews were conducted with participants and digitally recorded and transcribed verbatim. Interviews lasted between 30 and 80 min. Interviews were conducted face to face usually at the participants home, with a small number being conducted by telephone. The interview process was informed by narrative interviewing, 13 with the unstructured narrative interview designed to provide an opportunity for the participant to give a detailed account of a particular experience, event, or point in time.
Participants were encouraged to tell ‘their story’ as a narrative with minimal interruption from the first author which ensured events and issues important and significant to the participant were reported. The participants were encouraged to talk about the diagnosis and illness trajectory; what support was offered and how effective was support in the last year of life; were palliative care needs addressed; to discuss what happened in the last few weeks of life. This method was utilised in preference to a semi-structured interview design would have only allowed participants to select isolated parts from their own story and in effect filling in or completing pre-existing narratives by the first author. Field notes were collected to supplement the interpretation and analysis. The study was ongoing for 3 years with 18 months of this time spent on data collection. This prolonged period of time in the field helped the researcher who was an experienced mental health practitioner to acclimatise to researching an area of extreme sensitivity. It also supported development of skills that would help with conducting and understanding interviews and facilitated reflexivity which contributed to incorporating issues and theories from previous interviews into subsequent interviews. The field notes added to the context, nature, and circumstances of the interviews carried out.
During the research process and throughout interviews, the researcher attempted to create a climate of openness with participants in an attempt to reduce researcher effect. All participants were fully aware of the nature and purpose of the study. All transcripts were returned to the participants for verification and to ensure that participants’ narratives were recorded correctly. There were no requests for amendments following this process; however, many participants stated they had valued taking part and appreciated having a copy of their interview.
The study adopted a qualitative approach informed by phenomenology 14 and grounded theory. 15 , 16 Phenomenology as a philosophical stance due to the lived experiences being sought and grounded theory informed the study in an attempt to interpret and explain these experiences.
Analysis of the data using comparative analysis 15 commenced from the outset of data collection and transcripts were read by all authors and re-read by the first author. Line-by-line coding of transcripts, memo writing, development of categories, and emergence of themes followed and emerging themes were compared, discussed, and agreed by all authors who were part of the study team. Data saturation began to occur when recruitment reached 35 participants and a further five participants were recruited to ensure saturation had been fully achieved.
Participants were recruited from the North West of England: n = 21, North East of England: n = 4, Wales: n = 9 and Scotland: n = 6 and included participants living in urban, rural, and semi-rural areas. Thirty-one female and nine male family carers who had cared for people with dementia with an age range of 69–96 years (mean age 81.5 years) participated and included all socio-economic backgrounds. Efforts were made to recruit family carers where death had occurred in different care settings; however, the majority of participants had experienced death in hospital or care home with only four participants caring for a person with dementia within their family home until the end of life. No deaths had occurred in a hospice. Within the study, 22 people with dementia had died in Hospital, 14 within care homes and four within the family home ( Table 1 ) and the majority of family carers had experienced care in several different care settings in the year prior to death. Participants described how they had cared for the person with dementia for between 18 months to 20 years before death with a mean of 5.1 years and median of 5 years in a caring role. The deaths had occurred between 3 months and 5 years (mean 1.7 years, median 1.3 years) prior to the interviews.
Information regarding participants (reused with permission from Crowther et al. 17 ).
The mean length of time from formal diagnosis to death was 4.5 years; however, most participants reported cognitive problems for some time prior to diagnosis. Four participants were family carers for people who had a dual diagnosis of cancer and dementia. Of these, three experienced specialist palliative care services: one in a day hospice: one hospital-based and one a community-based specialist palliative care service. From the evidence of the interviews, no patient with a dementia-only diagnosis received specialist palliative care.
As a result of constant comparison of individual transcripts, 23 categories emerged from the data. Over a period of time, data were reduced further, and the following key themes began to emerge: communication; family carers as experts; the hard work of caring; and living and dying with dementia in the face of uncertainty. An example of an interview coding is included in Table 2 .
Examples of initial coding from text and memos.
Communication
Difficulties with communication in its broadest sense were a major theme to emerge from this study. Family carers knew the person and had established non-verbal means of communication
I knew when she was cold she would physically put her arms together and when she was too hot she would be unbuttoning her blouse I tried to get the staff to understand we had to look for non-verbal . . . when they don’t speak at all you’re having to work out what their needs are. (Family carer 36)
Family carers searched their creative knowledge of their loved ones in attempts to communicate and connect with the enduring self. This would involve playing music or having certain activities on a television channel they knew the person with dementia once enjoyed.
there was nothing we could give him, he had a television, we put it onto what we thought he enjoyed . . . in the summer he always liked to watch cricket we would tend to put that on . . . he liked hymns, certain singers, we tried to do this. (Family carer 39)
Just ‘being’ with the person with dementia became increasingly important for some family carers as the disease advanced and communication skills diminished.
Visiting . . . I couldn’t wait to get there . . . I was there every afternoon all afternoon for a fortnight . . . I went in everyday I held her hand . . . she knew me — I held her hand. (Family carer 40) I’d stay right through to evening . . . sit with mum just sit . . . talk to her . . . put the tv on . . . put some music on or I’d just sit there . . . (Family carer 31)
Communicating about care issues as the person with dementia deteriorated was another source of distress. The language used to inform family carers that the person with dementia was in the terminal phase was not always clear and added to the chaos and uncertainty experienced.
‘he’s very, very poorly, still very poorly’ they said. (Family carer 30)
Occasionally, a deterioration was reported and interpreted to mean the person with dementia was close to death. There appeared to be a subliminal message for family carers within the words used.
“about half past ten the staff nurse rang B and said “her breathing’s become a bit distressed” I said “right I’ll come now.” (Family carer 22)
A number of participants expressed concerns in relation to different languages and different cultures within UK hospitals and care homes. The quote below was the experience of a daughter whose father was Polish and reverting to his native language as dementia progressed – he had attended a memory clinic in the last year of life and the following were the daughter’s recollection of the clinic visit
he couldn’t write his name . . . my dad was Polish- the doctor was from another country . . . you can imagine . . . it were like never the twain shall meet. She wouldn’t let us stay in the room with him while she asked him these questions and we’d have to stand outside, we could hear what she was saying and she was getting quite agitated my dad wasn’t responding . . . he didn’t know how to respond . . . basically she wasn’t interested in him . . . she said there was no point giving him any tablets. (Family carer 21)
As dementia progresses, people often revert to the use of their native language and may totally loose their skills to speak or understand their second or third language. The importance of being able to communicate in the native language in order to facilitate assessment of cognitive deficits is demonstrated below.
she was lovely and spoke Welsh to him, she went through this rigmarole of questions and drawing then she said “will you write me a sentence?” . . . I thought “what’s going to happen here?” he said “of course I will” he picked up the paper, he wrote a sentence in Welsh do you know what that sentence was translated?.” it’s wonderful to have a young lady like you to visit me. (Family carer 39)
As dementia progressed, the ability to converse became more limited, and some family carers went to great lengths to choose 24-h care facilities which employed staff who could speak the native language of the person with dementia.
I really wanted him to go to a Welsh speaking care home I wanted people there who spoke to him in his mother tongue, that was very important. Not all of them did speak Welsh, but most of them understood Welsh and would know enough words to communicate. (Family carer 39)
Informal family carers as experts in care
Family carers within the study believed they were the “experts “ in care and in care preferences of their relative – in most cases, they had lived with, known, and cared for the person with dementia for many years prior to diagnosis and had witnessed the changes in the person with dementia following the diagnosis. Participants expressed specific wishes in relation to aspects of care. Informal family carers believed their level of knowledge and skill acquired, over many years of caring, was devalued, negated, and frequently ignored by professionals. Some expressed explicit wishes relating to the care of the person with dementia. The following quote was a patient with dementia and a cancer diagnosis – his wife found it unbearable that the oncologists insisted on informing her husband of his diagnosis at every clinic visit. Due to his dementia, he had no memory of previously being told his diagnosis but had sufficient insight to be devastated each time he was told his cancer diagnosis.
it was my belief he’d not got the mental capacity to know, every time he was told it would be like telling him again. She (oncologist) was really quite . . . not aggressive . . . it was her opinion he should know that was it I think relatives know - well I knew my husband better than anybody else, we’d been married for forty five years and it wasn’t appropriate to tell him . . . (Family carer 32)
As is demonstrated in the quote below, one of the most complex but important areas faced in caring for those with advancing disease is deciding when and how far to intervene when patients deteriorate. Including family carers in such decisions and listening to their views, where possible, can support the decision-making process and help arrive at solutions based on knowledge of that person and what maybe in their best interests.
they said about feeding him through his nose, I said “no” I knew he’d be traumatised, I knew he’d pull it out, he wouldn’t like being held down having that done so I said “absolutely no, no way that” When I go back in the afternoon they’d done it, he’d been very traumatised, I was furious. (Family carer 07)
The difficulty for family carers in hospitals when staff did not take notice of family carers’ views is illustrated below from the daughter of a patient who was sectioned (detained in a psychiatric hospital against their will) and admitted to a psychogeriatric ward
. . . he got put into ward X when he was sectioned, . . . “you’re in here Mr. H because you’ve hurt your wife” and I used to think, why are they saying that to him–how can you say that to somebody whose mind is like that . . ., It would make him so angry and he’d say “I do not, I do not hurt my wife!” you know and I feel me dad really, really suffered because he would not have hurt a fly and to keep being told . . . He’d say “this is punishment” he’d sit on ward X with his head in his hands and say “ ooh, they’ve said I’ve hurt P (wife), I love P, I’d never hurt P” you know and they were telling him all the time. It was terrible, it was absolutely dreadful and then he went into the nursing home thank God but I do feel for these people who haven’t got any family–what happens to them? (Family carer 09)
The hard work of caring
All interviews were retrospective accounts of events which had occurred months or some years previously, but recollections were vivid and emotional. Powerful words such as “fight,” “battles,” and “traumatic,” illustrated the difficulties confronting families and the hard work involved. Choice of words and language used were indicative of the enduring effect of the experiences and memories for the study participants.
then began the battle to get him out of hospital, it was a battle . . . they knew I wanted to get him home as soon as possible, it took 21 days to get him home, they told me it’s within the time limit. I pointed out to them S died 21 days after he came home so it may have seemed within their time limits, but it wasn’t within our time limits . . . we lost valuable time where he could have been at home didn’t we? (Family carer 32) I’d have to put him to bed, I think as a daughter, I think that’s been one of the hardest things which I have got over now, it would have made me cry so much it was just so upsetting. This fantastic man who’d done so much for so many other people, just become this shell terribly frightened, he just didn’t know where he was, kept asking for his own mother, I think quite a few times he thought I was his mum putting him to bed which for a daughter to think your father thinks you’re his mother was so very, very upsetting . . . (Family carer 03)
There was also the difficulties perceived by the attitude of hospital staff towards patients with dementia and pressure to move patients quickly to a care home. This very event for many families was traumatic, and the importance of going to a care home the family had chosen and wanted was important as illustrated below
. . . there was quite a bit of pressure actually from the local NHS here basically to get him out which I resisted because I wanted him to go into this particular nursing home, they wanted us to put him somewhere else . . . and wait for a bed . . . I felt that it was much better in one stage. (Family carer 39)
Living and dying with uncertainty and unpredictability – the last days
The level of uncertainty family carers of people with dementia may experience as death approached or appeared to approach caused distress to many family carers. Although told many times that a person with dementia may be near death, death could sometimes occur suddenly and unexpectedly. Uncertainty and unpredictability persisted sometimes over many months and occasionally years. Some family carers found themselves in situations where they were advised by medical staff the person with dementia was close to death only to see them recover, and for some, this occurred on several occasions during the disease trajectory and was a source of considerable anxiety and distress.
doctors were saying “he’s very poorly”, she was saying “you don’t know my dad, I’ll come in tomorrow, he’s going to be as right as rain, he’ll be off this mask” They were sort of like “right, right ok” that’s what happened to us so many times, then it got to Monday morning they said “he’s not going to last 24 hours. . . . (Family carer 20) he was a fighter that time he went in with pneumonia he wasn’t expected to come out . . . but he did. (Family carer 37)
Most family carers experienced death within a hospital setting and although for some, the care experience was good, it was common for people with dementia to die on an open busy ward with the promise of transfer to a side room not materialising.
it was horrible the way me dad died, absolutely horrible . . . it was dreadful . . . if he’d have been in a side room . . . had a little bit of dignity where it could have been quiet . . . (Family carer 9)
Of the four patients who had a dual diagnosis of cancer and dementia, one participant reported that her mother who had lung cancer and dementia had received day care in a local hospice while at home but died in a care home. Individual experiences of specialist palliative care services varied with one referral to a hospital team advising the family they were unable to help – this person died at home 4 weeks later. Only one family experienced palliative care in a hospital setting for a patient who had a diagnosis of gastric cancer and dementia. This participant was the only participant to mention the possibility of her father going to a hospice for end-of-life care, although this did not happen and he died on a hospital ward.
The one thing that I would have liked is for him to perhaps not have to stay so long in a clinical setting, he didn’t like hospitals at all and possibly, I think, we did discuss this with the doctors. . . . I think they thought that it wasn’t worth arranging to move him to a hospice or whatever because he wouldn’t survive . . . (Family carer 7)
Our study aimed to explore the experience of dementia in the last year of life in a diverse group of bereaved family carers from the UK in an attempt to identify and interpret what were the specific perceived needs of people with dementia and of their families at the end of life. Although a retrospective account, in all cases, carers gave an emotional and vivid account of events leading up to the death of the person with dementia. Communication in its broadest sense was the overarching issue of concern within this study and communication impacted on every aspect of care at the end of life.
As previously reported 18 , 19 communication issues were the main areas of concern in this study and were perceived as problematic by family carers in a range of circumstances, environments, and contexts and influenced every experience of care received. It was evident how difficulties with communication impacted on care and in this study, the experiences of communication were mostly negative. Non-verbal messages such as body language or para-verbal messages including tone and volume of voice have equal importance to verbal components of communication. 20 All aspects of communication influenced family carers’ experiences of end-of-life care. It is acknowledged that for many who work in health and social care in the United Kingdom, English is not their first language. Family carers experienced difficulties communicating with professionals in some circumstances and expressed concerns regarding the person with dementia who already had compromised communication skills as a result of their illness. For Welsh speaking family carers in Wales who participated in the study in particular, there was concern regarding the lack of access to support and care within their own native language and lack of access to care that was culturally acceptable. Sensitive communication and respect for family carers is a basic essential of good end-of-life care as is ensuring that family carers understand what is being said and listening to the family carers views and opinions.
Carers as experts
An expert is defined as ‘a person who is very knowledgeable about or skilful in a particular area’. Development of competent skills and extended knowledge about a particular subject, place, issue, or person is crucial to being assigned the title ‘expert’. There appeared to be a combination of experts in this study. Those who were professionals providing a service in which they were viewed as experts by lay people (i.e., the family carers interviewed for the study) and lay people (the family carers themselves) who considered themselves as experts in the care of the person with dementia. There was evidence of conflict and criticism mainly attributable to lack of acknowledgement and value perceived to be placed upon informal family carers expert knowledge and skills by professionals. Family carers felt they knew the person with dementia extremely well and based proxy decisions and choices on vast prior knowledge. They felt qualified as experts in care and found themselves, at times, in conflict and confrontation with professionals. Family carers considered themselves as expert in terms of knowledge and care of their loved ones 21 and what was in their best interests, while professionals appeared to consider themselves the experts in relation to dementia, disease trajectory, symptom management, and treatments. Families of people with dementia have frequently been living with the condition for a number of years prior to diagnosis and may have been making decisions based on their knowledge and previous wishes expressed by the person with dementia. These may, or may not, have been documented in a care plan. It was clear from this study that key areas of decision-making which created difficulties for the expert family carers and professionals alike included artificial hydration and nutrition, communication, and hospital admission. 22
Two recent papers based on a qualitative study of 30 bereaved carers of patients who had died of Lewy Body Disease also reported the lack of communication between health care teams and families and difficulty predicting death timing were two frequently expressed challenges. 23 , 24 Within our study, carers perceived professionals as inflexible; policy and procedures appeared to be followed regardless of information shared by informal family carers. It should be noted, that in this further analysis of a retrospective study, none of the participants in the study had an Advanced Care Plan (ACP); 25 , 26 however, a recent paper 27 reported that of over 6000 patients presenting with an acute medical emergency, only 4.8% had an ACP available for admitting medical team. In the study, 27 although 9.5% of patients over 80 and 12.6% of patients over 90 had an ACP, it is clear that the vast majority of older people, many of whom will have dementia as primary or co-morbidity do not have any documentation to state their wishes. Advance care planning (ACP) is a process of reflection on and communication of a person’s future health care wishes; 28 however, the process itself can be challenging as a recent systematic review reported and uncertainty with decision-making requires strategies and support to aid this complex process. 29 Since introduction of the Mental Capacity Act in the United Kingdom in 2005, 30 there has been increasing focus on ACP and treatment directives. This is in anticipation of loss of capacity and ability to make informed choices regarding treatments and wishes as capacity is lost and death may be approaching. In dementia, timing is crucial, as the person with dementia can be involved at an earlier phase but not necessarily later due to cognitive decline; however, for people newly diagnosed with dementia, it can be difficult to anticipate future care needs. What appeared most challenging for family carers within this study was the fact they felt they were not being listened to nor recognised by professionals.
The effects and impact of being a family carer for people with dementia has already been reported in the literature. 31 , 32 It became apparent that there were different elements to the hard work of caring namely the practical hard work and organisation of care; the physical hard work and the caring tasks required on a regular basis to support daily living and the emotional hard work related to burden, stress, anxiety, and guilt. The constant ‘battles’ family carers described when trying to negotiate the world of professional care was a drain on their emotional resources and such situations impacted on family carers causing stress and distress. 33 These findings are also echoed by a study which found that bereaved and current carers found it exhausting to co-ordinate care for the person with dementia especially regarding end-of-life care. 34
Uncertainty has been described as a common experience during illness, and dementia is no exception. 35 , 36 It could be argued that dying with dementia may be challenged with more uncertainty due to the potential length and unpredictable nature of the disease trajectory. The narratives shared within this study appeared to be fraught with chaos. Nothing within the last year of life including death itself appeared to be ordered or predictable. Issues regarding prognostication and dying in life-limiting illness remain problematic, and transfer from nursing home to hospital at end of life is common. 37 Many people with dementia also have co-morbidities. 38 It was of note that specialist palliative care services were rarely involved, and involvement in this study appeared limited to those with a dual diagnosis of dementia and cancer. Palliative care services for people with dementia were and are being developed but remain limited 17 , 39 and the debate regarding early introduction of palliative care in dementia continues. Currently, in the United Kingdom, less than 1% of people with dementia in the United Kingdom die within a hospice. In the United Kingdom, Admiral Nurses who are specialist nurses supporting people with dementia and their families are working in many areas including acute hospitals and can support both families and staff who are caring for patients with dementia at the end of life. Our findings in this study echo those of Bolt et al. 40 who found family members wanted to be recognised as an important caregiver and that a lack of person-centred care was an issue for many family members throughout the dementia illness.
Strengths and limitations
To our knowledge, this is one of the largest qualitative studies exploring the needs of patients with dementia at the end of life from the perspective of a diverse sample of bereaved family carers. Although every effort was made to include people from different ethnic backgrounds, this was not possible which limits the extent to which findings can be applied in other populations. The self-selecting nature of the sample could represent family carers with a more difficult experience wishing to participate and to share their experiences and those family carers who wished to participate may have been more engaged in caring for the person with dementia than the average family carer, and their desire to be heard, therefore greater. Conversely, it is possible that people with worse experiences of end-of-life care may not have wished to re-live the experience in an interview and that those interviewed for the study were possibly portraying better experiences of care. This was a United Kingdom–based study, and we acknowledge that findings may not be generalizable to other countries where provision of care is different.
Conclusion and recommendations
This large qualitative study of family carers revealed that communication in its broadest sense with the person with dementia and with professionals was a major issue. Family carers felt worn down by the constant ‘battles’ they experienced in negotiating the healthcare system on behalf of the person with dementia. Dying of dementia was frequently traumatic for family carers with little support and privacy and no support from specialist palliative care. Despite many publications and research on palliative care and dementia, 41 it is still important that all research is based on the experience of patients or their family carers who at the end of life are the advocates and the voice.
This paper is being written as we hopefully begin to emerge from the COVID-19 Pandemic which has seen a disproportionate number of people with dementia die of COVID-19. COVID-19 has disproportionately affected people with dementia, and in the post-pandemic era, there is an urgent need to ensure every person dying with dementia is supported to die in their preferred place and that family members are supported and enabled to be treated as the ‘expert’ in terms of their knowledge of their relatives’ care and preferences.
Communication with family members and determining their views regarding care at the end of life is essential as is ensuring people with dementia can die in their usual place of care among familiar surroundings. Although there is a belief that an ACP appears integral to aid delivery of good end of life for people with dementia, their completion can be problematic and very few people currently have a completed ACP. In such situations, it is essential to discuss with and include close family carers as partners in the care of the person with dementia as they know the person. If end-of-life decisions have not previously been discussed, family carers will be able to advise what may be the best care based on prior knowledge of what the person with dementia may or may not want. It is unclear whether the increase in numbers of people with dementia dying at home in the United Kingdom will continue, but what remains clear is the need for excellent communication along with support and respect for carers acknowledging their expertise, which with co-ordinated care will allow those with dementia to die in comfort and with dignity in the location of their choice.
Acknowledgments
The authors thank St Luke’s Hospice Winsford for a research grant which supported this study, and they thank all the family carers who so generously gave their time to be interviewed.
Ethics approval and consent to participate: Full ethical approval was awarded to this study by the University of Liverpool ethics committee. The authors are accountable for all aspects of the work in ensuring that questions related to the accuracy or integrity of any part of the work are appropriately investigated and resolved. All data collected for this study was with the full informed consent of all participants. Full ethical approval from University of Liverpool ethics committee was obtained for this study (RETH000206).
Consent for publication: All family carers who participated in the study consented to the publication of anonymised qualitative data.
Author contribution(s): Jacqueline Crowther: Data curation; Formal analysis; Investigation; Methodology; Writing – review & editing.
Kenneth Wilson: Formal analysis; Project administration; Writing – review & editing.
Mari Lloyd-Williams: Conceptualisation; Formal analysis; Funding acquisition; Investigation; Methodology; Supervision; Writing – original draft; Writing – review & editing.

Funding: The authors disclosed receipt of the following financial support for the research, authorship, and/or publication of this article: Research grant St Luke’s Hospice Winsford, Cheshire, UK.
Conflict of interest statement: The authors declared no potential conflicts of interest with respect to the research, authorship, and/or publication of this article.
Availability of data and materials: Raw data as in qualitative interviews is available by contact with authors
Contributor Information
Jacqueline Crowther, Academic Palliative and Supportive Care Studies Group (APSCSG) and Primary Care and Mental Health, University of Liverpool, Liverpool, UK.
Siobhan Horton, St Luke’s Hospice, Winsford, UK.
Kenneth Wilson, Department of Psychiatry, University of Liverpool, Liverpool, UK.
Mari Lloyd-Williams, Professor, Primary Care and Mental Health, Liverpool Health Partners and University of Liverpool, Brownlow Hill, Liverpool L69 3GB, UK.

Research involvement of medical students in a medical school of India: exploring knowledge, attitude, practices, and perceived barriers
- Find this author on Google Scholar
- Find this author on PubMed
- Search for this author on this site
- ORCID record for Abhinav Jha
- ORCID record for Deepak Dhamnetiya
- For correspondence: [email protected]
- ORCID record for Ravi Prakash Jha
- Info/History
- Preview PDF
Introduction: Research in the medical discipline significantly impacts society by improving the general well-being of the population, through improvements in diagnostic and treatment modalities. However, of 579 Indian medical colleges, 332 (57.3%) did not publish a single paper from the year 2005 to 2014," indicating a limited contribution from medical fraternity In order to probe in to the cause of this a study was conducted to assess the knowledge, attitude, practices (KAP) and perceived barriers to research among students of a medical school in Delhi, India. Methods: A cross-sectional study was conducted among medical students and the data on academic-cum-demographic information, assessment of knowledge, attitude, practices and barriers to research was collected using a pre-tested, semi-structured questionnaire. Chi-square test was used to check the association of various factors with the KAP of research. A p-value less than 0.05 was considered significant. Results: A total of 402 (N) subjects were enrolled in the study. Majority were male (79.6%) and from clinical professional years (57%). Majority (266, 66.2%) of the subjects had adequate knowledge. Of the study subjects (61,15%) having inadequate knowledge of research, sixty percent were from pre- and para- clinical years, while around 70 % of those having good knowledge were from clinical professional years. However, only 16.9% of the participants had participated in a research project, and only 4.72% had authored a publication. Sixty one percent of study subjects having a positive attitude towards research, were from pre- and para- clinical years. Among the study subjects having a positive attitude towards research, over 60% were from pre- and para- clinical years. The barriers for conducting research were mostly; lack of funds/laboratory equipment/infrastructure (85.1%), lack of exposure to opportunities for research in the medical (MBBS) curriculum (83.8%), and lack of time (83.3%). There was a statistically significant association between knowledge and attitude towards research with a professional year of study. Conclusions: The study revealed that while most of the students had a positive attitude towards research as well as an adequate knowledge of research, there was a poor level of participation in research. These challenges can be overcome by incorporating research as a part of the medical school curriculum from early years on, setting aside separate time for research, and establishing student research societies that can actively promote research.
Competing Interest Statement
The authors have declared no competing interest.
Funding Statement
This study did not receive any funding.
Author Declarations
I confirm all relevant ethical guidelines have been followed, and any necessary IRB and/or ethics committee approvals have been obtained.
The details of the IRB/oversight body that provided approval or exemption for the research described are given below:
The ethics committee of Dr Baba Saheb Ambedkar Medical College and Hospital, New Delhi gave ethical approval for this work.
I confirm that all necessary patient/participant consent has been obtained and the appropriate institutional forms have been archived, and that any patient/participant/sample identifiers included were not known to anyone (e.g., hospital staff, patients or participants themselves) outside the research group so cannot be used to identify individuals.
I understand that all clinical trials and any other prospective interventional studies must be registered with an ICMJE-approved registry, such as ClinicalTrials.gov. I confirm that any such study reported in the manuscript has been registered and the trial registration ID is provided (note: if posting a prospective study registered retrospectively, please provide a statement in the trial ID field explaining why the study was not registered in advance).
I have followed all appropriate research reporting guidelines, such as any relevant EQUATOR Network research reporting checklist(s) and other pertinent material, if applicable.
Data Availability
All data produced in the present study are available upon reasonable request to the authors.
View the discussion thread.
Thank you for your interest in spreading the word about medRxiv.
NOTE: Your email address is requested solely to identify you as the sender of this article.

Citation Manager Formats
- EndNote (tagged)
- EndNote 8 (xml)
- RefWorks Tagged
- Ref Manager
- Tweet Widget
- Facebook Like
- Google Plus One
- Addiction Medicine (324)
- Allergy and Immunology (628)
- Anesthesia (165)
- Cardiovascular Medicine (2381)
- Dentistry and Oral Medicine (289)
- Dermatology (207)
- Emergency Medicine (380)
- Endocrinology (including Diabetes Mellitus and Metabolic Disease) (838)
- Epidemiology (11777)
- Forensic Medicine (10)
- Gastroenterology (703)
- Genetic and Genomic Medicine (3751)
- Geriatric Medicine (350)
- Health Economics (635)
- Health Informatics (2401)
- Health Policy (933)
- Health Systems and Quality Improvement (899)
- Hematology (341)
- HIV/AIDS (782)
- Infectious Diseases (except HIV/AIDS) (13320)
- Intensive Care and Critical Care Medicine (768)
- Medical Education (366)
- Medical Ethics (105)
- Nephrology (398)
- Neurology (3510)
- Nursing (198)
- Nutrition (528)
- Obstetrics and Gynecology (675)
- Occupational and Environmental Health (665)
- Oncology (1825)
- Ophthalmology (538)
- Orthopedics (219)
- Otolaryngology (287)
- Pain Medicine (233)
- Palliative Medicine (66)
- Pathology (446)
- Pediatrics (1035)
- Pharmacology and Therapeutics (426)
- Primary Care Research (420)
- Psychiatry and Clinical Psychology (3180)
- Public and Global Health (6149)
- Radiology and Imaging (1280)
- Rehabilitation Medicine and Physical Therapy (749)
- Respiratory Medicine (828)
- Rheumatology (379)
- Sexual and Reproductive Health (372)
- Sports Medicine (323)
- Surgery (402)
- Toxicology (50)
- Transplantation (172)
- Urology (146)
Thank you for visiting nature.com. You are using a browser version with limited support for CSS. To obtain the best experience, we recommend you use a more up to date browser (or turn off compatibility mode in Internet Explorer). In the meantime, to ensure continued support, we are displaying the site without styles and JavaScript.
- View all journals
- Explore content
- About the journal
- Publish with us
- Sign up for alerts
- Published: 08 May 2024
Accurate structure prediction of biomolecular interactions with AlphaFold 3
- Josh Abramson ORCID: orcid.org/0009-0000-3496-6952 1 na1 ,
- Jonas Adler ORCID: orcid.org/0000-0001-9928-3407 1 na1 ,
- Jack Dunger 1 na1 ,
- Richard Evans ORCID: orcid.org/0000-0003-4675-8469 1 na1 ,
- Tim Green ORCID: orcid.org/0000-0002-3227-1505 1 na1 ,
- Alexander Pritzel ORCID: orcid.org/0000-0002-4233-9040 1 na1 ,
- Olaf Ronneberger ORCID: orcid.org/0000-0002-4266-1515 1 na1 ,
- Lindsay Willmore ORCID: orcid.org/0000-0003-4314-0778 1 na1 ,
- Andrew J. Ballard ORCID: orcid.org/0000-0003-4956-5304 1 ,
- Joshua Bambrick ORCID: orcid.org/0009-0003-3908-0722 2 ,
- Sebastian W. Bodenstein 1 ,
- David A. Evans 1 ,
- Chia-Chun Hung ORCID: orcid.org/0000-0002-5264-9165 2 ,
- Michael O’Neill 1 ,
- David Reiman ORCID: orcid.org/0000-0002-1605-7197 1 ,
- Kathryn Tunyasuvunakool ORCID: orcid.org/0000-0002-8594-1074 1 ,
- Zachary Wu ORCID: orcid.org/0000-0003-2429-9812 1 ,
- Akvilė Žemgulytė 1 ,
- Eirini Arvaniti 3 ,
- Charles Beattie ORCID: orcid.org/0000-0003-1840-054X 3 ,
- Ottavia Bertolli ORCID: orcid.org/0000-0001-8578-3216 3 ,
- Alex Bridgland 3 ,
- Alexey Cherepanov ORCID: orcid.org/0000-0002-5227-0622 4 ,
- Miles Congreve 4 ,
- Alexander I. Cowen-Rivers 3 ,
- Andrew Cowie ORCID: orcid.org/0000-0002-4491-1434 3 ,
- Michael Figurnov ORCID: orcid.org/0000-0003-1386-8741 3 ,
- Fabian B. Fuchs 3 ,
- Hannah Gladman 3 ,
- Rishub Jain 3 ,
- Yousuf A. Khan ORCID: orcid.org/0000-0003-0201-2796 3 ,
- Caroline M. R. Low 4 ,
- Kuba Perlin 3 ,
- Anna Potapenko 3 ,
- Pascal Savy 4 ,
- Sukhdeep Singh 3 ,
- Adrian Stecula ORCID: orcid.org/0000-0001-6914-6743 4 ,
- Ashok Thillaisundaram 3 ,
- Catherine Tong ORCID: orcid.org/0000-0001-7570-4801 4 ,
- Sergei Yakneen ORCID: orcid.org/0000-0001-7827-9839 4 ,
- Ellen D. Zhong ORCID: orcid.org/0000-0001-6345-1907 3 ,
- Michal Zielinski 3 ,
- Augustin Žídek ORCID: orcid.org/0000-0002-0748-9684 3 ,
- Victor Bapst 1 na2 ,
- Pushmeet Kohli ORCID: orcid.org/0000-0002-7466-7997 1 na2 ,
- Max Jaderberg ORCID: orcid.org/0000-0002-9033-2695 2 na2 ,
- Demis Hassabis ORCID: orcid.org/0000-0003-2812-9917 1 , 2 na2 &
- John M. Jumper ORCID: orcid.org/0000-0001-6169-6580 1 na2
Nature ( 2024 ) Cite this article
261k Accesses
1 Citations
1189 Altmetric
Metrics details
We are providing an unedited version of this manuscript to give early access to its findings. Before final publication, the manuscript will undergo further editing. Please note there may be errors present which affect the content, and all legal disclaimers apply.
- Drug discovery
- Machine learning
- Protein structure predictions
- Structural biology
The introduction of AlphaFold 2 1 has spurred a revolution in modelling the structure of proteins and their interactions, enabling a huge range of applications in protein modelling and design 2–6 . In this paper, we describe our AlphaFold 3 model with a substantially updated diffusion-based architecture, which is capable of joint structure prediction of complexes including proteins, nucleic acids, small molecules, ions, and modified residues. The new AlphaFold model demonstrates significantly improved accuracy over many previous specialised tools: far greater accuracy on protein-ligand interactions than state of the art docking tools, much higher accuracy on protein-nucleic acid interactions than nucleic-acid-specific predictors, and significantly higher antibody-antigen prediction accuracy than AlphaFold-Multimer v2.3 7,8 . Together these results show that high accuracy modelling across biomolecular space is possible within a single unified deep learning framework.
You have full access to this article via your institution.
Similar content being viewed by others
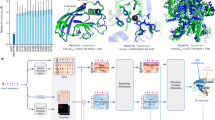
Highly accurate protein structure prediction with AlphaFold
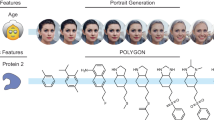
De novo generation of multi-target compounds using deep generative chemistry
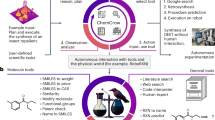
Augmenting large language models with chemistry tools
Author information.
These authors contributed equally: Josh Abramson, Jonas Adler, Jack Dunger, Richard Evans, Tim Green, Alexander Pritzel, Olaf Ronneberger, Lindsay Willmore
These authors jointly supervised this work: Victor Bapst, Pushmeet Kohli, Max Jaderberg, Demis Hassabis, John M. Jumper
Authors and Affiliations
Core Contributor, Google DeepMind, London, UK
Josh Abramson, Jonas Adler, Jack Dunger, Richard Evans, Tim Green, Alexander Pritzel, Olaf Ronneberger, Lindsay Willmore, Andrew J. Ballard, Sebastian W. Bodenstein, David A. Evans, Michael O’Neill, David Reiman, Kathryn Tunyasuvunakool, Zachary Wu, Akvilė Žemgulytė, Victor Bapst, Pushmeet Kohli, Demis Hassabis & John M. Jumper
Core Contributor, Isomorphic Labs, London, UK
Joshua Bambrick, Chia-Chun Hung, Max Jaderberg & Demis Hassabis
Google DeepMind, London, UK
Eirini Arvaniti, Charles Beattie, Ottavia Bertolli, Alex Bridgland, Alexander I. Cowen-Rivers, Andrew Cowie, Michael Figurnov, Fabian B. Fuchs, Hannah Gladman, Rishub Jain, Yousuf A. Khan, Kuba Perlin, Anna Potapenko, Sukhdeep Singh, Ashok Thillaisundaram, Ellen D. Zhong, Michal Zielinski & Augustin Žídek
Isomorphic Labs, London, UK
Alexey Cherepanov, Miles Congreve, Caroline M. R. Low, Pascal Savy, Adrian Stecula, Catherine Tong & Sergei Yakneen
You can also search for this author in PubMed Google Scholar
Corresponding authors
Correspondence to Max Jaderberg , Demis Hassabis or John M. Jumper .
Supplementary information
Supplementary information.
This Supplementary Information file contains the following 9 sections: (1) Notation; (2) Data pipeline; (3) Model architecture; (4) Auxiliary heads; (5) Training and inference; (6) Evaluation; (7) Differences to AlphaFold2 and AlphaFold-Multimer; (8) Supplemental Results; and (9) Appendix: CCD Code and PDB ID tables.
Reporting Summary
Rights and permissions.
Reprints and permissions
About this article
Cite this article.
Abramson, J., Adler, J., Dunger, J. et al. Accurate structure prediction of biomolecular interactions with AlphaFold 3. Nature (2024). https://doi.org/10.1038/s41586-024-07487-w
Download citation
Received : 19 December 2023
Accepted : 29 April 2024
Published : 08 May 2024
DOI : https://doi.org/10.1038/s41586-024-07487-w
Share this article
Anyone you share the following link with will be able to read this content:
Sorry, a shareable link is not currently available for this article.
Provided by the Springer Nature SharedIt content-sharing initiative
This article is cited by
Major alphafold upgrade offers boost for drug discovery.
- Ewen Callaway
Nature (2024)
By submitting a comment you agree to abide by our Terms and Community Guidelines . If you find something abusive or that does not comply with our terms or guidelines please flag it as inappropriate.
Quick links
- Explore articles by subject
- Guide to authors
- Editorial policies
Sign up for the Nature Briefing: Translational Research newsletter — top stories in biotechnology, drug discovery and pharma.


IMAGES
VIDEO
COMMENTS
Alzheimer's disease (AD) is the most common form of dementia, and accounts for over 60% of cases. Aging is the biggest risk factor for dementia. According to the World Health Organization (WHO ...
Introduction. Worldwide around 50 ... and an unfamiliar or changing environment. 286 Delirium in older people should prompt consideration of underlying dementia. Most research on delirium prevention has been in people without dementia. ... GL, JH, EBL, AS, DA, and ELS wrote first drafts of sections of the paper. GL wrote the first draft of the ...
INTRODUCTION. Dementia is a common public health problem. 1 Worldwide, ... 65 + years" (to exclude studies about less common causes of dementia). Original research studies with sample sizes less than 100 persons were excluded. OBSERVATIONS. The search yielded 200 articles published in the past five and a half years. We excluded 37 studies ...
1. Introduction. Alzheimer's disease (AD) (named after the German psychiatric Alois Alzheimer) is the most common type of dementia and can be defined as a slowly progressive neurodegenerative disease characterized by neuritic plaques and neurofibrillary tangles (Figure 1) as a result of amyloid-beta peptide's (Aβ) accumulation in the most affected area of the brain, the medial temporal ...
Dementia is a common public health problem. 1 Worldwide, approximately 47 million people have dementia, and this number is expected to increase to 131 million by 2050. 1 Reductions in age-adjusted incidence of dementia have occurred in the United States and other developed countries over the last 20 years, perhaps associated with increasing formal educational attainment.
Dementia, not a particular disease but rather a gathering of conditions which ascribes the debilitation of atleast two cerebrum capacities, cognitive decline and memory judgment has became ubiquous all over the world. Achieving accuracy and timely diagnosis is a major challenge in dementia detection. The recent advancements in neuroimaging techniques has setup some benchmarks. The amalgamation ...
IntroductionPatient involvement is a critical component of dementia research priority-setting exercises to ensure that research benefits are relevant and ... Introduction. It has been six years since the ... The idea that research should investigate the specific support needed for people living with dementia was evident in the papers reviewed. ...
Abstract and Figures. Dementia is a neurodegenerative disorder characterized by progressive and continuous loss of cognitive functions. The neuropsychiatric symptoms include apathy; agitation and ...
Most people with a record of dementia in linked UK EHR had some corroborating evidence for diagnosis. The estimated 10-year risk of dementia was higher than published population-based estimations. EHR are therefore a promising source of data for dementia research. Introduction
Here the authors present a deep learning framework for dementia diagnosis, which can identify persons with normal cognition, mild cognitive impairment, Alzheimer's disease, and dementia due to ...
Some specific causes of dementia disorders are explained below. Alzheimer's disease (AD) is the most common cause of dementia in older adults. More than 6 million Americans age 65 and older may have AD. Like other forms of dementia, the risk increases with age, but it can also occur in midlife, between a person's 30s and mid-60s. Early-onset AD
1 BACKGROUND. Prevalence of Alzheimer's disease and related dementias (ADRD) is high, affecting more than 10% of those aged 65 years or more, 1 with risk doubling every 5 years. 2 The Lancet Commission has identified 12 modifiable "life-course" risk factors responsible for an estimated 40% of the population attributable risk for dementia, 3 with age, genetics, and other factors accounting ...
Dening T, Sandilyan MB (2015) Dementia: definitions and types. Nursing Standard. 29, 37, 38-42. Date of submission: March 2 2014; date of acceptance: August 22 2014. Abstract. This articl e is ...
The number of older people, including those living with dementia, is rising, as younger age mortality declines. However, the age-specific incidence of dementia has fallen in many countries, probably because of improvements in education, nutrition, health care, and lifestyle changes. Overall, a growing body of evidence supports the nine potentially modifiable risk factors for dementia modelled ...
Introduction. Dementia is an umbrella term for multiple different subtypes, including Alzheimer's disease dementia, vascular dementia, and Lewy Body dementia, to name a few. ... quantitative and non-dementia papers included) and therefor findings should be interpreted with caution. The aim of this qualitative systematic review was to explore ...
Dementia is a clinical diagnosis requiring new functional dependence on the basis of progressive cognitive decline. It is estimated that 1.3% of the entire UK population, or 7.1% of those aged 65 or over, have dementia. Applying these to 2013 population estimates gives an estimated number of 19,765 people living with dementia in Northern Ireland.
About the Journal. Alzheimer's & Dementia® aims to bridge the knowledge gaps across a wide range of bench-to-bedside investigation in dementia and Alzheimer's Disease.. Announcement . We are pleased to announce that Alzheimer's & Dementia® will join Wiley's Open Access portfolio as of January 1, 2024. As a result, all submissions received from July 25, 2023 are subject to an a rticle p ...
Alzheimer's & Dementia: Translational Research & Clinical Interventions journal bridges drug discovery research and clinical studies for dementia & Alzheimer's. Abstract INTRODUCTION New therapies to prevent or delay the onset of symptoms, slow progression, or improve cognitive and behavioral symptoms of Alzheimer's disease (AD) are needed.
This VSI looks at the history of dementia research and examines the genetic, physiological, and environmental risk factors and how individuals might reduce them. It also investigates developments in diagnosis and symptom management, and the economic and political context of dementia care. Keywords: cure, dementia, diagnosis, treatment, ageing ...
Nowadays, Artificial Intelligence (AI) and machine learning (ML) have successfully provided automated solutions to numerous real-world problems. Healthcare is one of the most important research areas for ML researchers, with the aim of developing automated disease prediction systems. One of the disease detection problems that AI and ML researchers have focused on is dementia detection using ML ...
Introduction: Alzheimer's disease, something we hear about online, in commercials, on news stations, and in many other parts of life. ... Alzheimer's disease is a form of dementia, which is just an umbrella term used to describe loss of memory, language, problem solving, and other thinking abilities. ... However, a lot of research is still ...
Abstract. Although dementia has been described in ancient texts over many centuries (e.g., "Be kind to your father, even if his mind fail him.". - Old Testament: Sirach 3:12), our knowledge of its underlying causes is little more than a century old. Alzheimer published his now famous case study only 110 years ago, and our modern ...
In the NHS and HPFS, a lower risk of neurodegenerative disease mortality, including dementia mortality, was observed with higher olive oil consumption (HR, 0.81 [95% CI, 0.78-0.84]). 11 Evidence that pertains to cognitive decline or incident dementia is more widely available than it is for dementia mortality. 6,22 In the French Three-City Study ...
This paper will define dementia, its manifestations and types, discuss three research articles that deal with preventing dementia, and provide suggestions for future research on this topic. Defining Dementia. Dementia is a neurodegenerative disorder that results in the irreversible loss of brain functionality.
The study on APOE4 homozygosity indicates a genetic variant of Alzheimer's disease with early symptom onset and distinct biomarker progression, highlighting the need for specialized treatment ...
People with two copies, known as APOE4 homozygotes, make up 2 to 3 percent of the general population, but are an estimated 15 to 20 percent of people with Alzheimer's dementia, experts said.
Introduction. It is estimated that there are approximately 820,000 people with dementia in the United Kingdom, 1 and the incidence and prevalence of dementia will continue to rise with an ageing population. It is predicted that deaths from dementia in the United Kingdom will increase from around 59,000 per year in 2014 to around 220,000 per year by 2040. 2
Introduction: Research in the medical discipline significantly impacts society by improving the general well-being of the population, through improvements in diagnostic and treatment modalities. However, of 579 Indian medical colleges, 332 (57.3%) did not publish a single paper from the year 2005 to 2014," indicating a limited contribution from medical fraternity In order to probe in to the ...
The introduction of AlphaFold 21 has spurred a revolution in modelling the structure of proteins and their interactions, enabling a huge range of applications in protein modelling and design2-6.