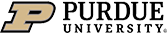

A FRAMEWORK TO INVESTIGATE KEY CHARACTERISTICS OF DIGITAL TWINS AND THEIR IMPACT ON PERFORMANCE
The modern world of manufacturing is in the middle of an industrial revolution with the digital and physical worlds integrating through cyber-physical systems. Through a virtual model that is able to communicate with its physical system known as the Digital Twin, catered decisions can be made based on the current state of the system. The digital twin presents immense opportunities and challenges as there is a greater need to understand how these new technologies work together.
This thesis is an experimental investigation of the characteristics of the essential components of the Digital Twin. A Digital Twin Framework is developed to explore the impacts of model accuracy and update frequency on the system’s performance measure. A simple inventory management system and a more complex manufacturing plant is modeled through the framework providing a method to study the interactions of the physical and digital systems with empirical data.
As the decision policies are affected by the state changes in the system, designing the Digital Twin must account for the direct and indirect impact of its components.
Furthermore, we show the importance of communication and information exchange between the Digital Twin and its physical system. A key characteristic for developing and applying a digital twin is to monitor the update frequency and its impact on performance. Through the study there are implications of optimal combinations of the digital twin components and how the physical system responds. There are also limits to how effective the Digital Twin can be in certain instances and is an area of research that needs further investigation.
The goal of this work is to help practitioners and researchers implement and use the Digital Twin more effectively. Better understanding the interactions of the model components will help guide designing Digital Twins to be more effective as they become an integral part of the future of manufacturing.
Degree Type
- Doctor of Philosophy
- Industrial Engineering
Campus location
- West Lafayette
Advisor/Supervisor/Committee Chair
Additional committee member 2, additional committee member 3, additional committee member 4, usage metrics.
- Modelling and simulation
- Manufacturing processes and technologies (excl. textiles)
- Manufacturing engineering not elsewhere classified
- Manufacturing management

Thank you for visiting nature.com. You are using a browser version with limited support for CSS. To obtain the best experience, we recommend you use a more up to date browser (or turn off compatibility mode in Internet Explorer). In the meantime, to ensure continued support, we are displaying the site without styles and JavaScript.
- View all journals
- Explore content
- About the journal
- Publish with us
- Sign up for alerts
- Perspective
- Published: 18 July 2022
Potential and limitations of digital twins to achieve the Sustainable Development Goals
- Asaf Tzachor ORCID: orcid.org/0000-0002-4032-4996 1 , 2 ,
- Soheil Sabri ORCID: orcid.org/0000-0002-2167-2717 3 ,
- Catherine E. Richards 1 , 4 ,
- Abbas Rajabifard 3 &
- Michele Acuto ORCID: orcid.org/0000-0003-4320-0531 5
Nature Sustainability volume 5 , pages 822–829 ( 2022 ) Cite this article
5386 Accesses
49 Citations
49 Altmetric
Metrics details
- Civil engineering
- Information technology
- Interdisciplinary studies
- Sustainability
Could computer simulation models drive our ambitions to sustainability in urban and non-urban environments? Digital twins, defined here as real-time, virtual replicas of physical and biological entities, may do just that. However, despite their touted potential, digital twins have not been examined critically in urban sustainability paradigms—not least in the Sustainable Development Goals framework. Accordingly, in this Perspective, we examine their benefits in promoting the Sustainable Development Goals. Then, we discuss critical limitations when modelling socio-technical and socio-ecological systems and go on to discuss measures to treat these limitations and design inclusive, reliable and responsible computer simulations for achieving sustainable development.
This is a preview of subscription content, access via your institution
Access options
Access Nature and 54 other Nature Portfolio journals
Get Nature+, our best-value online-access subscription
24,99 € / 30 days
cancel any time
Subscribe to this journal
Receive 12 digital issues and online access to articles
111,21 € per year
only 9,27 € per issue
Buy this article
- Purchase on Springer Link
- Instant access to full article PDF
Prices may be subject to local taxes which are calculated during checkout
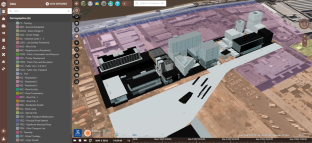
Similar content being viewed by others
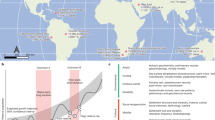
Frequent disturbances enhanced the resilience of past human populations
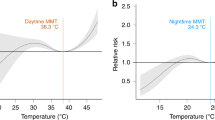
Heat health risk assessment in Philippine cities using remotely sensed data and social-ecological indicators
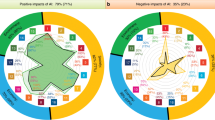
The role of artificial intelligence in achieving the Sustainable Development Goals
Wright, L. & Davidson, S. How to tell the difference between a model and a digital twin. Adv. Model. Simul. Eng. Sci. 7 , 13 (2020).
Article Google Scholar
Grieves, M. & Vickers, J. in Transdisciplinary Perspectives on Complex Systems (eds Kahlen, J. et al.) 85–113 (Springer, 2017).
Boschert, S. & Rosen, R. in Mechatronic Futures (eds Hehenberger, P. & Bradley, D.) 59–74 (Springer, 2016).
Tao, F. & Qi, Q. Make more digital twins. Nature 573 , 490–491 (2019).
Article CAS Google Scholar
Niederer, S. A., Sacks, M. S., Girolami, M. & Willcox, K. Scaling digital twins from the artisanal to the industrial. Nat. Comput. Sci. 1 , 313–320 (2021).
Bauer, P. et al. The digital revolution of Earth-system science. Nat. Comput. Sci. 1 , 104–113 (2021).
Rosen, R., Von Wichert, G., Lo, G. & Bettenhausen, K. D. About the importance of autonomy and digital twins for the future of manufacturing. IFAC PapersOnLine 48 , 567–572 (2015).
Tao, F., Zhang, H., Liu, A. & Nee, A. Y. Digital twin in industry: state-of-the-art. IEEE Trans. Industr. Inform. 15 , 2405–2415 (2018).
Cannoodt, R., Saelens, W., Deconinck, L. & Saeys, Y. Spearheading future omics analyses using dyngen, a multi-modal simulator of single cells. Nat. Commun. 12 , 3942 (2021).
Bruynseels, K., Santoni de Sio, F. & van den Hoven, J. Digital twins in health care: ethical implications of an emerging engineering paradigm. Front. Genet. 9 , 31 (2018).
Laubenbacher, R., Sluka, J. P. & Glazier, J. A. Using digital twins in viral infection. Science 371 , 1105–1106 (2021).
Bauer, P., Stevens, B. & Hazeleger, W. A digital twin of Earth for the green transition. Nat. Clim. Change 11 , 80–83 (2021).
Voosen, P. Europe is building a ‘digital twin’ of Earth to revolutionize climate forecasts. Science https://doi.org/10.1126/science.abf0687 (2020).
Deren, L., Wenbo, Y. & Zhenfeng, S. Smart city based on digital twins. Comput. Urban Sci. 1 , 4 (2021).
Francisco, A., Mohammadi, N. & Taylor, J. E. Smart city digital twin-enabled energy management: toward real-time urban building energy benchmarking. J. Manag. Eng. 36 , 04019045 (2020).
Jiang, Y., Yin, S., Li, K., Luo, H. & Kaynak, O. Industrial applications of digital twins. Phil. Trans. R. Soc. Lond. A 379 , 20200360 (2021).
Google Scholar
Marmolejo-Saucedo, J. A., Hurtado-Hernandez, M. & Suarez-Valdes, R. Digital twins in supply chain management: a brief literature review. In Proc. ICO 2019: Intelligent Computing and Optimization Vol. 1072 (eds Vasant, P. et al.) 653–661 (Springer, 2020).
El-Zahab, S. & Zayed, T. Leak detection in water distribution networks: an introductory overview. Smart Water 4 , 5 (2019).
Clemen, T. et al. Multi-agent systems and digital twins for smarter cities. In Proc. 2021 ACM SIGSIM Conference on Principles of Advanced Discrete Simulation 45–55 (ACM, 2021).
Havard, V., Jeanne, B., Lacomblez, M. & Baudry, D. Digital twin and virtual reality: a co-simulation environment for design and assessment of industrial workstations. Prod. Manuf. Res. 7 , 472–489 (2019).
Onile, A. E., Machlev, R., Petlenkov, E., Levron, Y. & Belikov, J. Uses of the digital twins concept for energy services, intelligent recommendation systems, and demand side management: A review. Energy Rep. 7 , 997–1015 (2021).
Dembski, F., Wössner, U., Letzgus, M., Ruddat, M. & Yamu, C. Urban digital twins for smart cities and citizens: the case study of Herrenberg, Germany. Sustainability 12 , 2307 (2020).
Lian, B. et al. Application of digital twins for remote operation of membrane capacitive deionization (mCDI) systems. Desalination 525 , 115482 (2022).
Designing Disruption: the critical role of Virtual Twins in accelerating Sustainability (Dassault Systèmes and Accenture, 2021).
Deng, S. et al. Edge intelligence: the confluence of edge computing and artificial intelligence. IEEE Internet Things J. 7 , 7457–7469 (2020).
Engström, R. E. et al. Succeeding at home and abroad: accounting for the international spillovers of cities’ SDG actions. npj Urban Sustain. 1 , 18 (2021).
Amirebrahimi, S., Rajabifard, A., Mendis, P. & Ngo, T. A BIM-GIS integration method in support of the assessment and 3D visualisation of flood damage to a building. J. Spat. Sci. 61 , 317–350 (2016).
Rajabifard, A. et al. in Sustainable Development Goals Connectivity Dilemma (ed. Rajabifard, A.) 243–255 (CRC, 2019).
Sabri, S. & Rajabifard, A. in Sustainable Development Goals Connectivity Dilemma (ed. Rajabifard, A.) 199–211 (CRC, 2019).
Assarkhaniki, Z., Sabri, S. & Rajabifard, A. Using open data to detect the structure and pattern of informal settlements: an outset to support inclusive SDGs’ achievement. Big Earth Data 5 , 497–526 (2021).
Vinuesa, R. et al. The role of artificial intelligence in achieving the Sustainable Development Goals. Nat. Commun. 11 , 233 (2020).
Karvonen, A. et al. The ‘New Urban Science’: towards the interdisciplinary and transdisciplinary pursuit of sustainable transformations. Urban Transform. 3 , 9 (2021).
Bettencourt, L. M. A. Introduction to Urban Science: Evidence and Theory of Cities as Complex Systems (MIT Press, 2021).
Acuto, M. & Parnell, S. Leave no city behind. Science 352 , 873 (2016).
Kapteyn, M. G., Pretorius, J. V. & Willcox, K. E. A probabilistic graphical model foundation for enabling predictive digital twins at scale. Nat. Comput. Sci. 1 , 337–347 (2021).
Ragnedda, M. & Gladkova, A. (eds) Digital Inequalities in the Global South (Springer, 2020).
Pick, J. B. & Azari, R. Global digital divide: influence of socioeconomic, governmental, and accessibility factors on information technology. Inf. Technol. Dev. 14 , 91–115 (2008).
Chinn, M. D. & Fairlie, R. W. The determinants of the global digital divide: a cross-country analysis of computer and internet penetration. Oxf. Econ. Pap. 59 , 16–44 (2007).
Rodriguez, F. & Wilson, E. J. Are Poor Countries Losing the Information Revolution? (World Bank, 2000).
Niu, J., Tang, W., Xu, F., Zhou, X. & Song, Y. Global research on artificial intelligence from 1990–2014: spatially-explicit bibliometric analysis. ISPRS Int. J. Geoinf. 5 , 66 (2016).
Schrotter, G. & Hürzeler, C. The digital twin of the city of Zurich for urban planning. J. Photogramm. Remote. Sens. Geoinf. Sci. 88 , 99–112 (2020).
United Nations Statistics Division in The Sustainable Development Goals Report 2019 (United Nations, 2019); https://unstats.un.org/sdgs/report/2019/goal-11/
Derudder, B. & Van Meeteren, M. Engaging with ‘urban science’. Urban Geogr. 40 , 555–564 (2019).
Bai, X. et al. Networking urban science, policy and practice for sustainability. Curr. Opin. Environ. Sustain. 39 , 114–122 (2019).
Hillier, B. in Digital Urban Modeling and Simulation Vol. 242 (eds Arisona, S. M. et al.) 24–48 (Springer, 2012).
Smajgl, A., Brown, D. G., Valbuena, D. & Huigen, M. G. Empirical characterisation of agent behaviours in socio-ecological systems. Environ. Model. Softw. 26 , 837–844 (2011).
Karlsson, J. M., Bring, A., Peterson, G. D., Gordon, L. J. & Destouni, G. Opportunities and limitations to detect climate-related regime shifts in inland Arctic ecosystems through eco-hydrological monitoring. Environ. Res. Lett. 6 , 014015 (2011).
Laikre, L. et al. Compromising genetic diversity in the wild: unmonitored large-scale release of plants and animals. Trends Ecol. Evol. 25 , 520–529 (2010).
Edmonds, B. & Meyer, R. (eds) Simulating Social Complexity: A Handbook (Springer, 2013).
Slater, T. Shaking Up the City: Ignorance, Inequality, and the Urban Question (Univ. California Press, 2021).
Brusaporci, S. in 3D Printing: Breakthroughs in Research and Practice (ed. Information Resources Management Association) 333–360 (IGI Global, 2017).
Zou, J. & Schiebinger, L. AI can be sexist and racist—it’s time to make it fair. Nature 559 , 324–326 (2018).
Fuso Nerini, F. et al. Mapping synergies and trade-offs between energy and the Sustainable Development Goals. Nat. Energy 3 , 10–15 (2018).
Zhao, Z. et al. Synergies and tradeoffs among Sustainable Development Goals across boundaries in a metacoupled world. Sci. Total Environ. 751 , 141749 (2021).
Tzachor, A., Devare, M., King, B., Avin, S. & Ó hÉigeartaigh, S. Responsible artificial intelligence in agriculture requires systemic understanding of risks and externalities. Nat. Mach. Intell. 4 , 104–109 (2022).
Chen, Y. & Landry, D. Capturing the rains: comparing Chinese and World Bank hydropower projects in Cameroon and pathways for south–south and north nouth technology transfer. Energy Policy 115 , 561–571 (2018).
Stilgoe, J., Owen, R., & Macnaghten, P. in The Ethics of Nanotechnology, Geoengineering and Clean Energy (eds Maynard, A. & Stilgoe, J.) 347–359 (Routledge, 2020).
Stahl, B. C. & Wright, D. Ethics and privacy in AI and big data: implementing responsible research and innovation. IEEE Secur. Priv. 16 , 26–33 (2018).
Jirotka, M., Grimpe, B., Stahl, B., Eden, G. & Hartswood, M. Responsible research and innovation in the digital age. Commun. ACM 60 , 62–68 (2017).
Kaissis, G. et al. End-to-end privacy preserving deep learning on multi-institutional medical imaging. Nat. Mach. Intell. 3 , 473–484 (2021).
Transforming our World: The 2030 Agenda for Sustainable Development (United Nations, 2015).
Download references
Acknowledgements
This paper was made possible through the support of a grant from Templeton World Charity Foundation, Inc. The opinions expressed in this publication are those of the author(s) and do not necessarily reflect the views of Templeton World Charity Foundation, Inc. We thank Y. Chen from the University of Melbourne for supporting the production of Figs. 1 – 3 .
Author information
Authors and affiliations.
University of Cambridge, Centre for the Study of Existential Risk (CSER), Cambridge, UK
Asaf Tzachor & Catherine E. Richards
Reichman University (IDC Herzliya), School of Sustainability, Herzliya, Israel
- Asaf Tzachor
University of Melbourne, School of Engineering, Department of Infrastructure Engineering, Centre for SDIs and Land Administration, Melbourne, Victoria, Australia
Soheil Sabri & Abbas Rajabifard
University of Cambridge, Department of Engineering, Cambridge, UK
- Catherine E. Richards
University of Melbourne, Faculty of Architecture, Building and Planning, Melbourne, Victoria, Australia
Michele Acuto
You can also search for this author in PubMed Google Scholar
Contributions
A.T., S.S., C.E.R., A.R. and M.A. developed the paper jointly and all contributed equally to the writing of the text.
Corresponding author
Correspondence to Asaf Tzachor .
Ethics declarations
Competing interests.
A.T., C.E.R., S.S. and M.A. declare no competing interests. A.R. manages the Centre for Spatial Data Infrastructures and Land Administration (CSDILA) at the University of Melbourne. CSDILA developed the Fishermans Bend Digital Twin proof of concept in partnership with the Department of Environment, Land, Water and Planning (DELWP), State Government of Victoria, Australia.
Peer review
Peer review information.
Nature Sustainability thanks Thomas Clemen, Fabian Dembski, Andrew Karvonen and Jack Stilgoe for their contribution to the peer review of this work.
Additional information
Publisher’s note Springer Nature remains neutral with regard to jurisdictional claims in published maps and institutional affiliations.
Rights and permissions
Reprints and permissions
About this article
Cite this article.
Tzachor, A., Sabri, S., Richards, C.E. et al. Potential and limitations of digital twins to achieve the Sustainable Development Goals. Nat Sustain 5 , 822–829 (2022). https://doi.org/10.1038/s41893-022-00923-7
Download citation
Received : 16 September 2021
Accepted : 31 May 2022
Published : 18 July 2022
Issue Date : October 2022
DOI : https://doi.org/10.1038/s41893-022-00923-7
Share this article
Anyone you share the following link with will be able to read this content:
Sorry, a shareable link is not currently available for this article.
Provided by the Springer Nature SharedIt content-sharing initiative
This article is cited by
The increasing potential and challenges of digital twins.
Nature Computational Science (2024)
Circular systems engineering
- Istvan David
- Dominik Bork
- Gerti Kappel
Software and Systems Modeling (2024)
Digital twins: a stepping stone to achieve ocean sustainability?
- Ofir Hendel
npj Ocean Sustainability (2023)
Rewards, risks and responsible deployment of artificial intelligence in water systems
- Richard Fenner
Nature Water (2023)
Digital Avatar of Metrology
- Sanjay Yadav
MAPAN (2023)
Quick links
- Explore articles by subject
- Guide to authors
- Editorial policies
Sign up for the Nature Briefing: AI and Robotics newsletter — what matters in AI and robotics research, free to your inbox weekly.

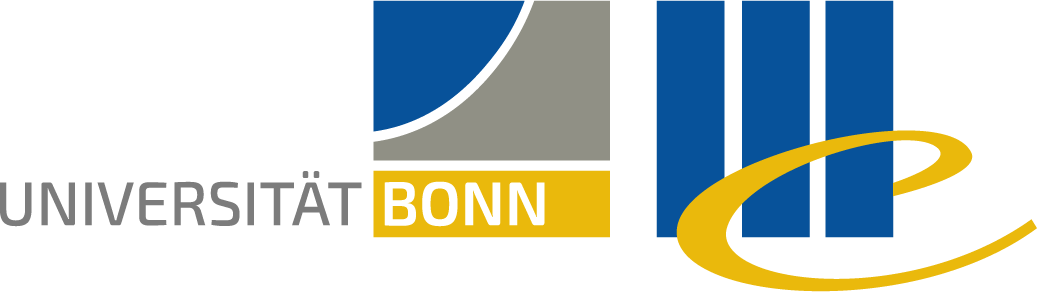
- About bonndoc
- bonndoc Home
- Fakultäten der Universität Bonn
- Mathematisch-Naturwissenschaftliche Fakultät
- E-Dissertationen
- Faculties of University Bonn
- The Faculty of Mathematics and Natural Sciences
Semantic Digital Twins in the Industrial Internet of Things
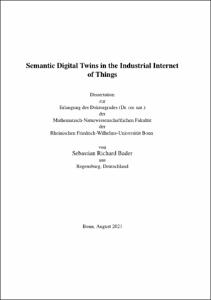

Type of Scholarly Publication
Date of exam, date of publication, involved institutions, citable links.
- Handle: https://hdl.handle.net/20.500.11811/9884
- URN: https://nbn-resolving.org/urn:nbn:de:hbz:5-66298
Classification (DDC)
- Zitiervorschlag
The following license files are associated with this item:
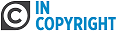
MIT Libraries home DSpace@MIT
- DSpace@MIT Home
- MIT Libraries
- Graduate Theses
Digital twin technology for enhanced upstream capability in oil and gas
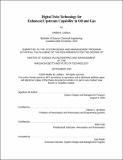
Other Contributors
Terms of use, description, date issued, collections.

- < Previous
Home > Robinson College of Business > Robinson College of Business Executive Programs > Business Administration > BUS_ADMIN_DISS > 162
Business Administration Dissertations
The concept of digital twins and its impact on organizational performance.
Deepa Vivekanandan , Georgia State University Follow
Author ORCID Identifier
https://orcid.org/0000-0002-4714-9713
Date of Award
Spring 5-6-2022
Degree Type
Dissertation
Degree Name
Doctor of Business Administration (DBA)
First Advisor
Dr. Richard L. Baskerville
Second Advisor
Dr. Lars Mathiassen
Third Advisor
Dr. Subhashish Samaddar
The world has become “digital” in many aspects and due to which witnessed a great deal of innovation in products, services, and experiences. The innovation using digital technologies has served meaningfully for many organizations. Digital Twin is an emerging concept that was introduced two decades ago which creates a virtual replica by extending the use of some of the advanced digital techniques and technologies.
Limited research is available to conceptualize Digital Twins clearly, analyze the stages of maturity of Digital Twins, and articulate the novel aspects of the technology with an emphasis on similarities, differences, and connections between the Digital Twin and other existing technologies such as modeling, simulation, artificial intelligence, and the internet of things which would be the first focus of the study. The characterization can provide clarity on Digital Twin technology to the academicians, practitioners, and organizational leadership.
Digital Twin has started to transition from being an idea or a prototype to a real-world implementation tied to one or more applications. Research studies, academic papers, and many case studies are available to demonstrate existing and potential applications in multiple industries and fields. There is very limited research that ties the applications to organizational performance constructs, namely financial performance, operational excellence, and customer perspective which would be the second focus of the study. The elaboration on how the Digital Twin technology impacts organizational performance aspects would benefit the audience looking for proven approaches and models to realize business value from the technology.
Organizations experience a number of challenges during the planning and implementation of Digital Twins that spans across data, business processes, systems and applications, infrastructure, stakeholders, business operating model, technology adoption, business value realization, regulatory aspects, and more. The third focus of the study would explore the Digital Twin related challenges using academic literature, practice literature, and practitioner experiences. The exploration of challenges would benefit the practitioners to effectively manage issues, mitigate risks, set realistic expectations with leadership, and plan communications with stakeholders.
Qualitative grounded theory research involving academic literature, practice sources, and practitioner interviews as the primary data collection techniques is used for the three focus areas of the study. The primary data analysis approaches involve coding, memo writing, and analytic induction. The study involves an iterative data collection and analysis from all three data sources. The record of the study is documented as a thesis for use by the relevant audience.
https://doi.org/10.57709/28941911
Recommended Citation
Vivekanandan, Deepa, "The Concept of Digital Twins and its Impact on Organizational Performance." Dissertation, Georgia State University, 2022. doi: https://doi.org/10.57709/28941911
File Upload Confirmation
Since May 02, 2022
Advanced Search
- Notify me via email or RSS
- Collections
- Disciplines
- Submit ETD (Thesis/Dissertation)
Home | About | FAQ | My Account | Accessibility Statement
Privacy Copyright

- < Previous
Home > Theses and Dissertations > 6483
Theses and Dissertations
Simulation-based and data-driven approaches to industrial digital twinning towards autonomous smart manufacturing systems, date of award.
Summer 2021
Document Type
Open Access Dissertation
Mechanical Engineering
First Advisor
A manufacturing paradigm shift from conventional control pyramids to decentralized, service-oriented, and cyber-physical systems (CPSs) is taking place in today’s Industry 4.0 revolution. Generally accepted roles and implementation recipes of cyber systems are expected to be standardized in the future of manufacturing industry. Developing affordable and customizable cyber-physical production system (CPPS) and digital twin implementations infuses new vitality for current Industry 4.0 and Smart Manufacturing initiatives. Specially, Smart Manufacturing systems are currently looking for methods to connect factories to control processes in a more dynamic and open environment by filling the gaps between virtual and physical systems.
The work presented in this dissertation first utilizes industrial digital transformation methods for the automation of robotic manufacturing systems, constructing a simulation-based surrogate system as a digital twin to visually represent manufacturing cells, accurately simulate robot behaviors, promptly predict system faults and adaptively control manipulated variables. Then, a CPS-enabled control architecture is presented that accommodates: intelligent information systems involving domain knowledge, empirical model, and simulation; fast and secured industrial communication networks; cognitive automation by rapid signal analytics and machine learning (ML) based feature extraction; and interoperability between machine and human. A successful semantic integration of process indicators is fundamental to future control autonomy. Hence, a product-centered signature mapping approach to automated digital twinning is further presented featuring a hybrid implementation of smart sensing, signature-based 3D shape feature extractor, and knowledge taxonomy. Furthermore, capabilities of members in the family of Deep Reinforcement Learning (DRL) are explored within the context of manufacturing operational control intelligence. Preliminary training results are presented in this work as a trial to incorporate DRL-based Artificial Intelligence (AI) to industrial control processes.
The results of this dissertation demonstrate a digital thread of autonomous Smart Manufacturing lifecycle that enables complex signal processing, semantic integration, automatic derivation of manufacturing strategies, intelligent scheduling of operations and virtual verification at a system level. The successful integration of currently available industrial platforms not only provides facile environments for process verification and optimization, but also facilitates derived strategies to be readily deployable to physical shop floor.
The dissertation finishes with summary, conclusions, and suggestions for further work.
© 2021, Kaishu Xia
Recommended Citation
Xia, K.(2021). Simulation-Based and Data-Driven Approaches to Industrial Digital Twinning Towards Autonomous Smart Manufacturing Systems. (Doctoral dissertation). Retrieved from https://scholarcommons.sc.edu/etd/6483
Since January 11, 2022
Included in
Mechanical Engineering Commons
Advanced Search
- Notify me via email or RSS
- Collections
- Disciplines
Submissions
- Give us Feedback
- University Libraries
Home | About | FAQ | My Account | Accessibility Statement
Privacy Copyright
Digital twins: When and why to use one
April 30, 2024 by Mickael Brossard , Mithun Kamat , Tomás Lajous , Kayvaun Rowshankish , and Cenk Tunasar
This blog post is the second in a three-part series on digital twins. The first post explained how generative AI and digital twins make a powerful pairing. The third post in the series will explore an in-depth case study on how generative AI and digital twins could be used to enhance customer experience.
When most people consider digital twins, they think of the visualization and simulation tools used to develop innovative new products. Offshore oil platforms, racing sailboats, luxury automobile engines, hotels, and train stations are just some of the things that have been designed and refined using advanced digital simulations.
These uses represent a fraction of what digital twins can accomplish. It is helpful to think of digital twins less as a tool for designers, engineers, and manufacturers and more as a laboratory in which nearly any organization can optimize its most precious resource—information—to continually push the boundaries of what it can accomplish. By using data to mirror real-world situations, digital twins can be deployed to create, fine-tune, or entirely reimagine nearly any complex process or system, including supply chains, public transit systems, and assembly lines.
For example, a global retailer recently set out to rethink its supply chain with an eye toward cutting costs, optimizing service, and boosting sustainability. It was a complex problem that involved optimizing an array of key levers, such as inventory positioning, product flow optimization, supply planning, and carbon emissions. Drawing on the organization’s vast quantities of real-time data, a team created a digital twin of its global supply chain operations—a sprawling system of manufacturing facilities, freight and cargo operations, third-party contractors, and distribution centers. The digital replica allowed the retailer to test more than 50 scenarios a day, examining potential outcomes for various large and small choices along the supply chain, all without any real-life disruptions. An optimization engine embedded within the digital twin provided users with informed recommendations in the meantime. Ultimately, the company made a series of optimized decisions that sparked a 7 percent reduction in carbon emissions and a 5 percent improvement in customer orders received on time.
Digital twins offer immense value, but only under the right circumstances
Clearly, digital twins have come a long way since the 1960s, when NASA engineers tested simulations of Apollo spacecraft using early digital models. It’s also clear that a growing number of organizations are intrigued by the technology and are looking at ways to adopt it. Yet it’s important to understand that digital twins are not a one-size-fits-all solution for business problems. Digital twins are as complex and nuanced as the systems and objects they seek to emulate and can deliver value only in particular circumstances. How, then, can a business determine when and where to implement a digital twin? There are several key characteristics to look for:
- High-stakes areas with high costs and real revenue on the line. The problem to be solved must be relevant enough to warrant significant investment. Building digital twins requires considerable effort and resources, and businesses should be fairly certain they can derive value from the effort.
- A complex or dynamic environment. Some products and systems are so complicated that inefficiencies and their root causes are difficult to identify. In the supply chain, for example, a change to one element can lead to second- or third-order effects that manifest over time. This is the kind of situation that could benefit from a digital twin. Simpler environments, characterized by more direct and apparent chains of cause and effect, can likely be optimized manually through more standard forms of investigation and analysis.
- The availability of high-quality data. Digital twins require a substantial amount of high-quality data to accurately represent their real-world counterparts and simulate the underlying product, system, or process. The data set must be robust and reliable across all the problem’s parameters for the issue to be solved; data that is incomplete or otherwise flawed can undermine, or even doom, a twin’s effectiveness.
- The environment being simulated is recurring. Digital twins are built to be used and reused, to repeatedly simulate and optimize multivariable problems. If the objective is to solve for a one-time optimization of a single variable, an optimization model would work better than a digital twin.
If these requirements are met, a digital twin can deliver considerable impact. For example, a leading semiconductor manufacturer was repeatedly losing bids because it relied on slow-moving, inefficient design and production processes. This was a high-stakes, complex problem with no immediately apparent root cause—precisely the kind of problem that digital twins are best suited to address. Using historical data, the business built a digital twin that leveraged AI and machine learning to rapidly run multiple simulations of potential designs. This process generated insights that decreased time to market and increased first-time-right designs by up to 25 percent. Engineering capacity saw a 20 percent boost as time spent on manual physics models, which previously had used traditional methods and took hours, was reduced to seconds through the creation of a deep learning surrogate model.
Even when it meets the foundational prerequisites (including a viable business case, high-quality data, and ongoing operational demand), a digital twin is far from a sure thing. Digital twins can fail to deliver for a number of reasons. One common pitfall is the incorrect configuration of technical components, which can compromise the accuracy and reliability of the digital twin’s simulations and insights. Additionally, the long-term viability of the business case—and thus the digital twin—may be limited as market conditions, technological advancements, or organizational priorities shift over time. Finally, a lack of sustained maintenance and updates to the digital twin can lead to its gradual obsolescence. These challenges underscore the importance of holistic planning, ongoing evaluation, and agile adaptation strategies to ensure the enduring success of digital-twin initiatives.
Taking the next step
With a clear understanding of business objectives and meticulous diligence in implementation, a digital twin can unlock new realms of possibilities. The emergence of generative AI, with its ability to analyze vast quantities of structured and unstructured data and generate insights via user-friendly interfaces, promises to supercharge the promise even more (see the first post in this series, “ Digital twins and generative AI: A powerful pairing ”). If your company has a complex and thorny problem that is holding back performance, the technology is well worth your consideration.
Mickael Brossard is a partner in McKinsey’s Paris office; Mithun Kamat is a partner in the Dallas office; Tomás Lajous and Kayvaun Rowshankish are senior partners in the New York office; and Cenk Tunasar is a partner in the Boston office.
- Hjem
- Universitetet i Stavanger
- Faculty of Science and Technology
- Department of Industrial Economics, Risk Management and Planning (TN-ISØP)
- Studentoppgaver (TN-ISØP)
- Vis innførsel
Digital twin technology: A study of differences from simulation modelling and applicability in improving risk analysis.
Ibrahim, oula, master thesis.
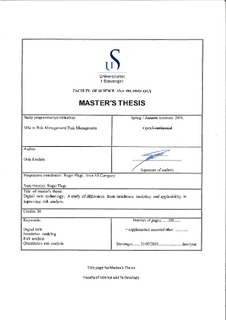
Permanent lenke
Utgivelsesdato.
- Studentoppgaver (TN-ISØP) [1411]
Beskrivelse

COMMENTS
A Thesis submitted in partial fulfilment of the requirements for the degree of: Master of Science in Construction Management University of Washington 2022 ... Digital Twin Technology is a newly emerging technology in the construction industry, but its concept is not new. The concept of Digital Twin (DT) came around 2002 when it was first used ...
A Comprehensive Review of Digital Twin - Part 1: Modeling and Twinning Enabling Technologies Adam Thelen1, Xiaoge Zhang2, Olga Fink3, Yan Lu4, Sayan Ghosh5, Byeng D. Youn6, Michael D. Todd7, Sankaran Mahadevan8, Chao Hu1* and Zhen Hu9* 1Department of Mechanical Engineering, Iowa State University, Ames, 50011, IA, USA. 2Department of Industrial and Systems Engineering, The Hong Kong Polytechnic
A digital twin is a computational model that evolves over time to persistently represent a unique physical asset. Digital twins underpin intelligent automation by enabling asset-specific analysis and data-driven decision-making. ... This thesis develops mathematical and computational foundations to support the transition from this custom ...
Digital twins can iterate and evolve through the seamless connection and fusion between the virtual and physical spaces1, and this consistency and synchronization can bring many benefits to numer ...
Digital twin technology is part of the rise of new digital technologies that support digital transformation by providing capabilities to enable new business models and decision support systems. ... He has directed 42 Ph.D. dissertations and 24 M. S. theses, and has taught several industry short courses on reliability and risk analysis methods ...
Massachusetts Institute of Technology
This digital twin consists of an organizational view and a product view, thereby solving the knowledge boundaries that have been identified in a case study in a Philips hardware development team. Next to this, a distinction is made between a field digital twin and design digital twin. The thesis ends with several implications
The motivation for this thesis is to investigate the possibilities introduced by creating a personal medical Digital Twin that follows you from birth to death and beyond, keeping you informed on your health and storing all your health related data safely in the cloud. This thesis will focus on the area of gathering speci c medical info on an indi-
The digital twin presents immense opportunities and challenges as there is a greater need to understand how these new technologies work together. This thesis is an experimental investigation of the characteristics of the essential components of the Digital Twin. A Digital Twin Framework is developed to explore the impacts of model accuracy and ...
Recently, it has been proposed to build digital twins also of environmental and climate systems.22 As the climate depends on consumption and emission patterns, such a digital twin would probably consider - at least to some extent - models of the world economy and societies on our planet. Some experts, therefore, demand that such digital twins
digital twin has increasing attention by both industry and academic. Existing primary applications of the digital twin in industry are briefly summarised as follows. Figure 3: Evaluation of digital twin (Source: PTC) The concept of DT was first proposed and primarily used in product or equipment prognostics and health management (PHM).
Masters thesis 2022 64 pages, 15 figures, 3 tables and 1 appendix ... Digital twin implementation in the equipment maintenance in a real gas and oil company 43 3.1. Case description 43 3.2. The economic parameters of implementing a digital twin of ESP pump 46 3.3. Proposed architecture for digital twin of pump 49
The Fishermans Bend Digital Twin project aggregated more than 1,400 data layers including historical and real-time data in two- and three-dimensions, from both public and private platforms, into a ...
Type of Thesis: Master's Thesis Title: Digital Twin implementation in Operations and Maintenance phase - Components and Benefits Author: George Michael Quaye Study no: 20190146 ~ iii ~ Abstract The Digital twin concept which is a result of the fourth industrial revolution has led to many
The digital twin is a real-time virtual representation of a physical product, platform or ecosystem that enables you to model, visualize, predict and provide feedback through simulations performed on the ... As it was probably the case with many master students, my master thesis project also contained quite some challenges. To overcome these ...
1.2.2. Past work on review. Negri, Fumagalli, and Macchi (Citation 2017) reviewed the roles of the digital twin in the cyber-physical system (CPS)-based production systems.This paper also presented the history of the concept, the definitions of the digital twin in scientific literature, its role within Industry 4.0 and some recommendations for future research.
The concept of Digital Twins is extended and specified to consistent and self-declarative entities imposing the atomic building blocks for the integration of components, facilities, and applications across networks, companies, and domains. Based on proven technologies, widely accepted conventions, and new extensions, new patterns are outlined ...
Abstract. Digital twins are receiving considerable attention as a cutting-edge technology that will transform the oil and gas industry. Powered by a digital thread that connects data across the product lifecycle, a digital twin virtually mirrors or emulates processes, assets, and projects in real-time to generate highly valuable insights.
The world has become "digital" in many aspects and due to which witnessed a great deal of innovation in products, services, and experiences. The innovation using digital technologies has served meaningfully for many organizations. Digital Twin is an emerging concept that was introduced two decades ago which creates a virtual replica by extending the use of some of the advanced digital ...
The concept of the Digital Twin dates back to a University of Michigan. presentation to industry in 2002 for the formation of a Product Lifecycle. Management (PLM) center. The presentation slide ...
This thesis conducts a qualitative technology assessment to determine the effects that adopting digital twins has on the DON's enterprise architecture. Analysis of an enterprise-wide adoption identifies opportunities and risks of digital twins within the context of the DON's strategy, processes, people, technology, cyber security, and risk ...
Xia, K. (2021). Simulation-Based and Data-Driven Approaches to Industrial Digital Twinning Towards Autonomous Smart Manufacturing Systems. (Doctoral dissertation). Retrieved from https://scholarcommons.sc.edu/etd/6483. A manufacturing paradigm shift from conventional control pyramids to decentralized, service-oriented, and cyber-physical ...
The environment being simulated is recurring. Digital twins are built to be used and reused, to repeatedly simulate and optimize multivariable problems. If the objective is to solve for a one-time optimization of a single variable, an optimization model would work better than a digital twin. If these requirements are met, a digital twin can ...
Digital twin excels simulation with many new features which would help a lot enhancing risk analysis through the lifecycle of a project, such as managing operational risk in the operation phase or supporting decision-making by providing experience data for future analysis. ... Master's thesis in Risk Management. Utgiver University of Stavanger ...